Sentiment-Driven Coaching is transforming the way chatbots interact with users by enhancing the conversational experience. Imagine a chatbot capable of recognizing the emotional tone of a user’s voice, allowing it to tailor responses accordingly. This approach enables chatbots to shift from merely answering questions to engaging users in meaningful dialogues that reflect their feelings and needs.
In today’s competitive landscape, harnessing call sentiment data is essential for effective coaching. By analyzing emotional cues, chatbots can improve their responses, create better user interactions, and ultimately drive customer satisfaction. The implementation of sentiment-driven coaching not only aids in understanding user emotions but also establishes a foundation for personalized engagement that can lead to more successful outcomes.
Analyze & Evaluate Calls. At Scale.
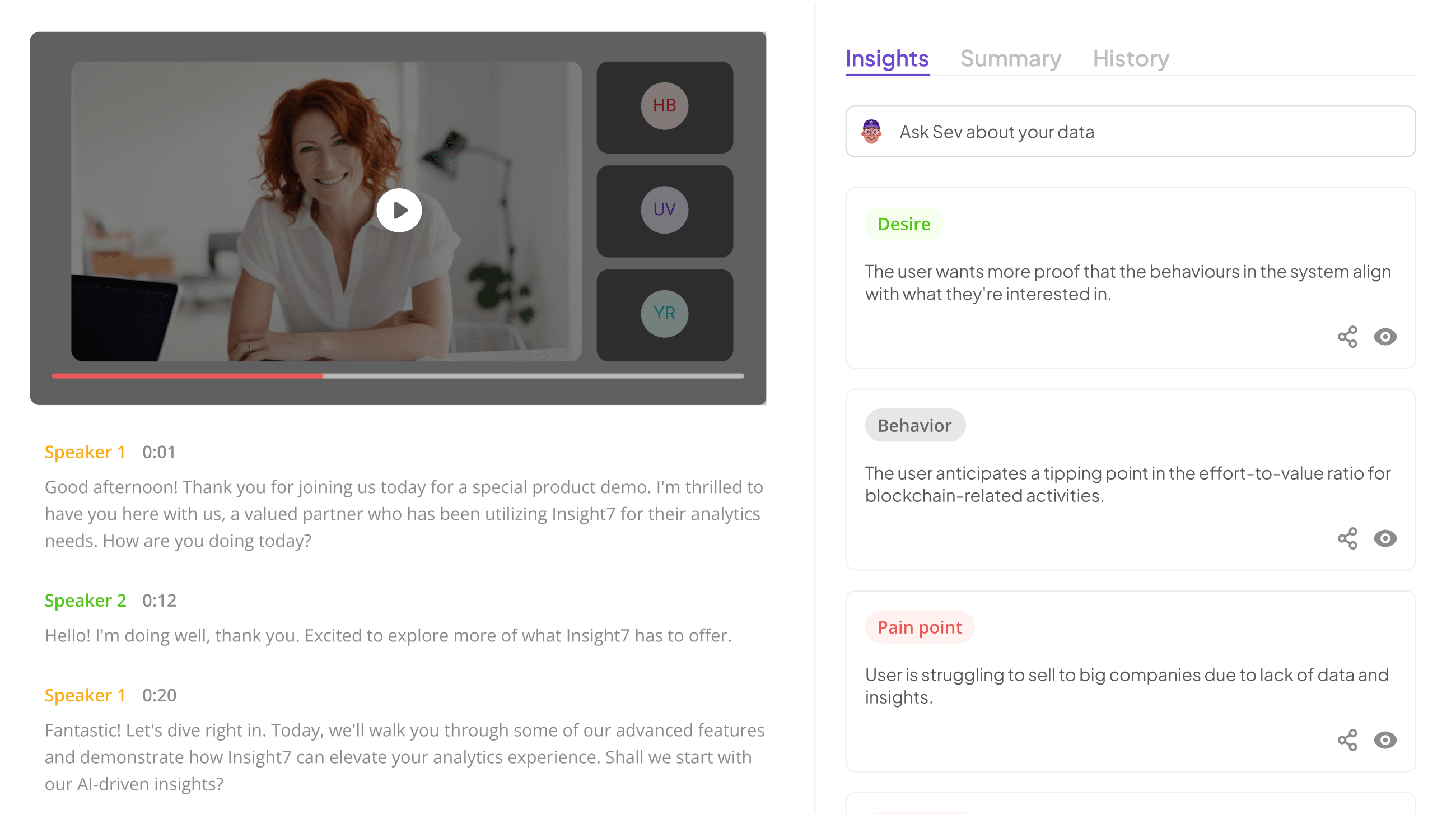
Understanding Sentiment-Driven Coaching
Sentiment-Driven Coaching offers a transformative approach to enhance the interactions between chatbots and users. By analyzing the emotions expressed within a conversation, this method tailors responses and coaching prompts to fit the emotional context, resulting in more effective communication. For instance, if a customer is frustrated, a sentiment-driven chatbot can provide empathetic responses that acknowledge the user's feelings, thereby fostering a more supportive environment.
To effectively implement sentiment-driven coaching, it’s essential to understand several key elements. Firstly, gathering detailed sentiment data allows the chatbot to decipher emotional cues and adjust its responses accordingly. Secondly, integrating sophisticated algorithms enables chatbots to interpret these sentiments, shaping coaching prompts that resonate with users. Lastly, continuous refinement and analysis of interaction patterns enhance the system's ability to adapt and improve, ensuring lasting engagement and satisfaction.
What is Sentiment-Driven Coaching?
Sentiment-Driven Coaching refers to a technique that harnesses emotional cues from user interactions to refine coaching strategies in real-time. By analyzing the sentiment expressed during conversations, these coaching methods adapt to individual needs, promoting a more personalized approach. This adaptation is critical in fostering a supportive environment, enhancing users' experience and engagement.
In practice, Sentiment-Driven Coaching utilizes data from calls to identify themes like pain points, desires, and challenges. This information can reveal trends, allowing for tailored feedback that resonates with users. For instance, if a user communicates frustration, the coaching prompts can adjust to offer reassurance and guidance. This dynamic approach not only improves the learning experience but also builds trust between the bot and the user, ultimately leading to better outcomes. By proactively addressing emotions expressed in conversations, organizations can significantly enhance their coaching efforts.
The Role of Sentiment Analysis in Coaching Prompts
Sentiment-driven coaching plays a pivotal role in enhancing the effectiveness of chatbots. By analyzing user emotions and feedback, chatbots can tailor their responses to meet individual needs more effectively. The integration of sentiment analysis allows chatbots to identify pain points, desires, and complaints expressed during interactions. This capability transforms generic responses into personalized coaching prompts that address specific user concerns.
A key aspect of sentiment analysis is its ability to extract themes from conversations. Chatbots can highlight recurring issues or positive feedback, enabling continuous improvement in service delivery. When users express frustration, chatbots can provide solutions or empathy, fostering a more engaging experience. Conversely, when users share achievements or positive sentiments, chatbots can amplify this positivity through encouragement or relevant resources. Ultimately, sentiment analysis empowers chatbots to drive relevant coaching prompts, enhancing user satisfaction and overall outcomes.
Extract insights from interviews, calls, surveys and reviews for insights in minutes
Implementing Sentiment-Driven Coaching in Chatbots
Implementing sentiment-driven coaching in chatbots involves integrating sentiment analysis into the conversation flow to enhance user engagement. Initially, it requires gathering and analyzing sentiment data from customer interactions. By systematically assessing emotions expressed during conversations, chatbots can adapt their responses to be more empathetic and supportive. This process not only improves user satisfaction but also creates a more personalized experience.
Once the sentiment data is collected, the next step is to build coaching algorithms that react accordingly. For instance, if a user expresses frustration, the chatbot can prompt a solution-oriented response or offer additional assistance. Utilizing advanced natural language processing technologies enables chatbots to discern underlying emotions. As a result, companies can foster deeper connections with their customers through a more empathetic approach. Implementing sentiment-driven coaching ultimately empowers organizations to refine their customer interactions and achieve higher satisfaction levels.
Key Steps to Develop a Sentiment-Driven Chatbot
To develop a sentiment-driven chatbot, begin by gathering and analyzing sentiment data from customer interactions. This data serves as the foundation for understanding user emotions and preferences. Utilize voice and text analysis to identify patterns that help gauge overall sentiment during conversations. Having a robust dataset will enable the chatbot to offer more relevant and empathetic responses, thereby enhancing user satisfaction.
Next, focus on building coaching algorithms based on the sentiment data collected. Create frameworks that allow the chatbot to recognize when a user is frustrated or needs additional support. Implementing these algorithms ensures that the chatbot can deliver timely and tailored coaching prompts based on user sentiment. This responsive approach fosters a more engaging interaction, ultimately aiding in driving positive outcomes for users. Consider integrating diverse tools that facilitate sentiment analysis to refine these steps effectively.
Step 1: Gathering and Analyzing Sentiment Data
The first stage in establishing effective sentiment-driven coaching involves meticulously gathering and analyzing sentiment data. Begin by conducting thorough call analyses to unearth emotions expressed by users. High attention to detail will reveal key themes including pain points, preferences, and feedback. By scrutinizing these elements, you can identify significant challenges that customers encounter, ensuring your insights are rooted in their genuine experiences.
Next, employ sentiment analysis tools to quantify emotions and attitudes conveyed during calls. This process translates subjective feelings into actionable insights, allowing your chatbot to understand and respond with empathy. By bringing together qualitative data from call transcripts and quantitative sentiment scores, you set a solid groundwork for developing tailored coaching prompts. Ultimately, this precise gathering and analyzing of sentiment data forms the bedrock for implementing effective coaching strategies that resonate with users and enhance their interactions within the chatbot framework.
Step 2: Building Coaching Algorithms Based on Sentiment
In the next phase of developing sentiment-driven coaching algorithms, it is essential to focus on the data collected from prior interactions. By leveraging sentiment analysis, chatbots can identify user emotions and attitudes expressed during conversations. This technique enables the algorithm to categorize sentiments into positive, negative, or neutral, supporting targeted follow-ups based on emotional cues. Understanding these sentiments fosters a more empathetic interaction, leading to improved user experiences and satisfaction.
To build effective coaching algorithms, consider the following key components. First, establish a framework for identifying common themes in user feedback and interactions. Next, integrate pattern recognition capabilities that can detect changes in sentiment over time. Finally, program the chatbot to generate tailored coaching prompts based on the sentiment trends observed in the data. These foundational elements collectively enhance the chatbot's ability to deliver meaningful coaching interventions that resonate with users.
Top Tools for Sentiment-Driven Coaching
In the realm of sentiment-driven coaching, various tools can enhance how chatbots interact with users. These technologies employ advanced sentiment analysis to modify coaching prompts based on customer emotions and feedback. Understanding the nuances of communication enables chatbots to deliver tailored coaching, fostering a more engaging customer experience.
First, Insight7 excels at analyzing conversations at scale, allowing businesses to extract valuable insights quickly. IBM Watson Tone Analyzer focuses on identifying emotional cues, empowering coaches to adjust their strategies effectively. Google Cloud Natural Language API provides robust language processing to understand user sentiments deeply. Microsoft Azure Text Analytics offers versatile sentiment analysis solutions, while Lexalytics specializes in extracting insights from unstructured data. Each of these tools enhances the potential for sentiment-driven coaching, enabling organizations to respond intelligently to customer needs and improve overall service delivery.
Insight7
Chatbots that utilize call sentiment as a basis for coaching prompts can dramatically enhance employee performance. Understanding customer emotions during interactions allows organizations to tailor feedback that resonates more deeply with users. This approach shifts coaching strategies from generic advice to targeted insights, enabling representatives to develop skills in areas that truly matter to their performance.
Sentiment-driven coaching ensures that feedback is not just a formality but a powerful tool for improvement. By implementing effective sentiment analysis, organizations can transform their coaching processes. The focus should be on understanding individual emotional contexts, making feedback sessions more personalized and impactful. Emotional intelligence in coaching leads to a healthier work environment and better customer satisfaction outcomes.
IBM Watson Tone Analyzer
The IBM Watson Tone Analyzer serves as a powerful tool in enhancing chatbots' capabilities to understand and respond to user sentiments effectively. By analyzing text inputs for emotional tone, it helps in tailoring coaching prompts directly aligned with user feelings. For instance, if a user expresses frustration, the chatbot can adjust its response to offer support and reassurance, helping to de-escalate tensions. This ability to gauge emotions contributes significantly to the effectiveness of sentiment-driven coaching in chatbots.
Key features of the Tone Analyzer include its ability to assess various emotional and linguistic tones, such as joy, sadness, anger, and analytical traits. By leveraging these insights, chatbots can provide personalized responses that resonate better with users. This approach not only enhances user satisfaction but also informs training programs for customer service representatives, ensuring they can deliver a more empathetic and effective response during interactions.
Google Cloud Natural Language API
The Google Cloud Natural Language API serves as a powerful tool in the landscape of sentiment-driven coaching. This API enables chatbots to analyze text and discern emotional undertones, transforming interactions into valuable insights. By leveraging advanced machine learning techniques, it can evaluate the sentiment behind customer conversations, identifying nuances in tone that human oversight might miss. This capability helps organizations provide tailored responses that resonate with caller emotions, enhancing the overall customer experience.
The efficiency of this API is evident in its ability to extract sentiments from large volumes of data swiftly. Once integrated, chatbots can generate prompts that guide interactions based on emotional cues. This not only empowers coaching initiatives but also aids in creating a more empathetic communication framework. As a result, organizations can optimize their support processes, ensuring that customer interactions are both meaningful and contextually relevant.
Microsoft Azure Text Analytics
Integrating Microsoft Azure Text Analytics into chatbot development opens a gateway to enhanced customer interactions. This robust platform excels at performing sentiment analysis, which is crucial for informing coaching prompts. By analyzing real-time communication data, organizations can pinpoint customer emotions and identify areas for improvement. The insights drawn from this analysis enable chatbots to respond more adeptly to customer needs, creating a more engaging experience.
Sentiment-driven coaching becomes transformative when paired with powerful analytical tools. These tools can extract key phrases, assess tonal nuances, and provide metrics that help identify sentiment trends over time. Coaches can utilize this data to tailor their strategies, ensuring agents are prepared for various emotional contexts. Ultimately, effective use of text analytics not only enriches chatbot interactions but also drives continuous improvement in team performance and customer satisfaction.
Lexalytics
Integrating advanced sentiment analysis capabilities into coaching practices can significantly enhance interaction outcomes. With the right tools, chatbots can effectively assess the emotional tone of conversations, providing insights that lead to improved coaching strategies. This technology transforms how feedback is delivered, ensuring that coaching prompts are not only timely but also contextually relevant.
The importance of accurate sentiment analysis cannot be overstated. It minimizes biases and inconsistencies that may arise during discussions, allowing for a more objective understanding of user experiences. Coaches can utilize these insights to refine their approaches, ensuring that each interaction is personalized and impactful. The adoption of such technologies paves the way for a new era in coaching, where emotions and sentiments play a central role in shaping meaningful engagements. Ultimately, sentiment-driven coaching can elevate performance, leading to transformative results across various domains.
Conclusion on Sentiment-Driven Coaching in Chatbots
Sentiment-Driven Coaching in chatbots represents a transformative approach to enhancing customer interactions. By effectively leveraging sentiment analysis, chatbots can gain insights into users’ emotional states, leading to tailored coaching prompts that resonate with their needs. This personalized touch not only improves user satisfaction but also fosters stronger connections between customers and brands.
As we move forward, the integration of sentiment analysis will likely become a pivotal practice in customer service. Organizations that embrace this technology will find themselves better equipped to address customer concerns proactively, refining their training and evaluation processes. Ultimately, the journey toward implementing sentiment-driven coaching will pave the way for a more empathetic and responsive chatbot experience.