Predictive QA Insights unlock valuable perspectives on customer satisfaction, revealing patterns that often go unnoticed. Companies today collect vast amounts of quality assurance data, yet many struggle to convert this information into actionable insights. As businesses prioritize understanding their customers, integrating predictive insights can provide a significant competitive edge.
The ability to analyze QA data effectively allows organizations to anticipate customer needs and respond proactively. By unveiling these insights, companies can enhance their service delivery and improve overall customer experiences. Understanding how to leverage this data will empower businesses to foster stronger relationships with their clientele.
Analyze qualitative data. At Scale.
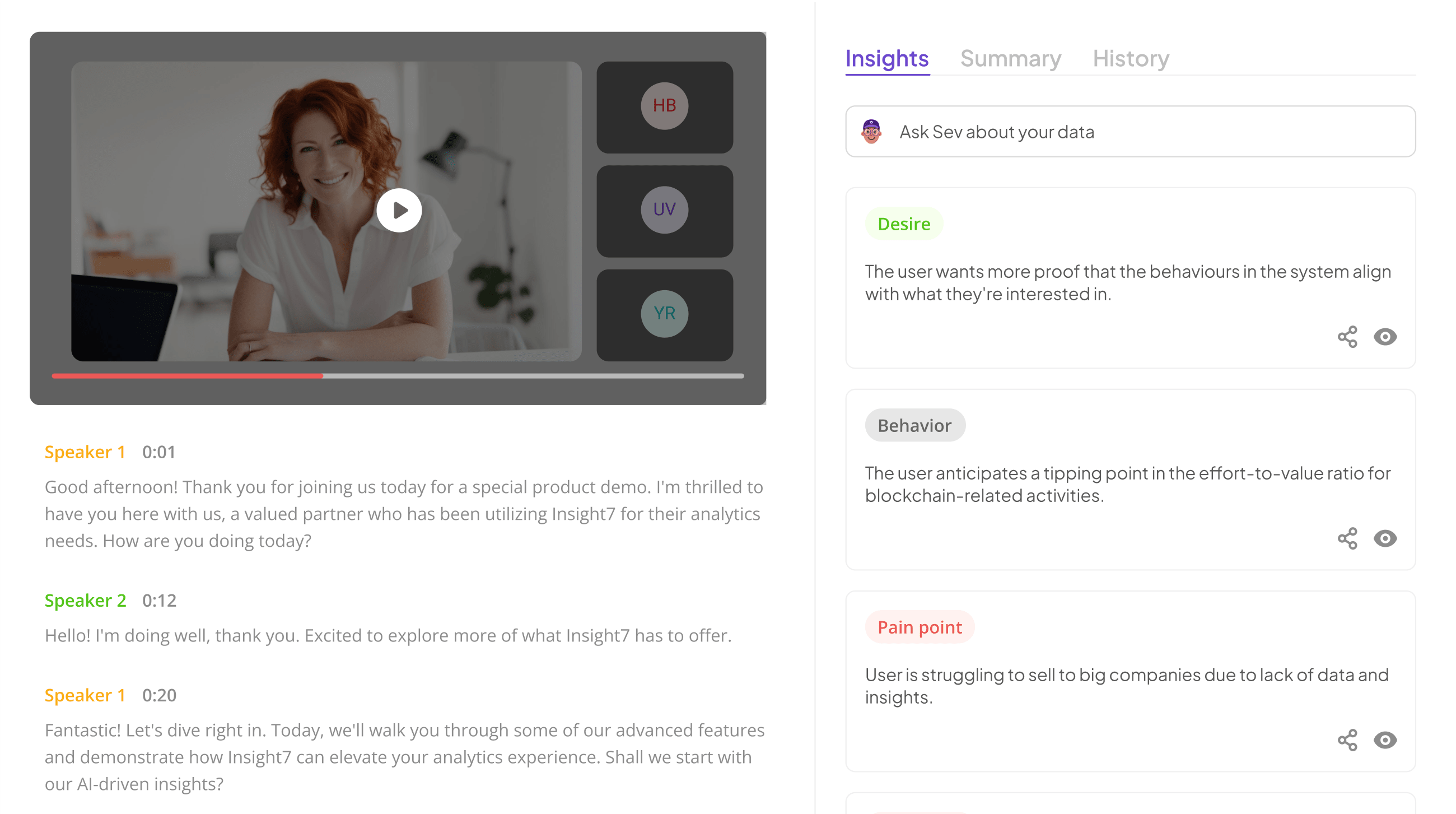
Understanding Predictive QA Insights and Customer Satisfaction
Predictive QA insights are pivotal for understanding customer satisfaction trends. By analyzing QA data, businesses can gain a clearer picture of customer preferences and expectations. This alignment fosters proactive decision-making, enabling companies to enhance their products and services based on reliable data signals.
The significance of predictive QA insights lies in their ability to reveal patterns that are not immediately obvious. For instance, a consistent drop in quality scores can indicate looming issues that might affect customer satisfaction. By dissecting QA data, companies can prioritize areas for improvement that directly impact the customer experience, ensuring that issues are addressed swiftly and effectively. This approach not only helps in retaining existing customers but also attracts new ones through positive word-of-mouth and enhanced service reliability.
Predictive QA Insights serve as a valuable asset for understanding customer satisfaction. By analyzing Quality Assurance (QA) data, companies can identify trends that highlight customer experiences. This data encompasses various interactions, providing insight into agents' performance, effectiveness in communication, and overall service quality. When agents demonstrate expertise in addressing customer concerns, it can significantly enhance satisfaction levels. However, it's crucial to differentiate between agent-related issues and other factors affecting customer emotions.
To harness Predictive QA Insights effectively, organizations should focus on specific strategies that dive deeper into their QA data. Firstly, gathering comprehensive data from customer interactions establishes a solid foundation for analysis. Next, identifying key metrics, such as response times and resolution rates, allows businesses to pinpoint specific areas for improvement. Lastly, utilizing analytical tools can transform raw data into actionable insights, illuminating patterns that inform strategies to enhance customer satisfaction over time.
The Role of QA Data in Predicting Consumer Needs
QA data plays a crucial role in understanding consumer needs, as it serves as a vital source of information. By analyzing quality assurance data, businesses can uncover patterns and trends that indicate customer satisfaction levels. This data not only reflects customer feedback but also highlights elements that may require improvement. Consequently, organizations can develop strategies that more closely align with consumer expectations.
Predictive QA insights provide a pathway to anticipating future consumer demands. By systematically extracting information from QA interactions, businesses can determine common themes that affect customer experiences. This analysis helps identify strengths and areas for development. Additionally, proactively addressing these insights allows companies to enhance service quality, thereby improving overall customer satisfaction and ensuring they remain ahead of market demands.
- Explanation of QA data and its importance
QA data serves as a vital resource for understanding customer experiences and behaviors. It encompasses feedback from quality assurance processes, including customer interactions and service assessments. The analysis of this data can reveal trends that are indicative of customer satisfaction levels. By harnessing QA data, businesses can extract valuable insights that help in predicting future customer needs and satisfaction, creating a roadmap to enhance their overall service.
The importance of QA data lies in its ability to pinpoint areas for improvement and identify strengths in the customer service process. It provides a clear picture of customer sentiments, enabling teams to make informed decisions. Predictive QA insights can transform raw data into actionable strategies, allowing businesses to proactively address potential issues. Ultimately, leveraging QA data fosters a deeper understanding of customer preferences, thereby facilitating improved engagement and loyalty.
- How QA data can signal customer satisfaction levels
QA data plays a crucial role in assessing customer satisfaction levels. It serves as a direct line to customer experiences, highlighting both strengths and areas needing improvement. By analyzing feedback and interactions captured in QA processes, businesses can gain insights into what customers value and where their expectations may not be met. This understanding enables organizations to enhance their offerings, ultimately increasing customer loyalty.
One effective way to leverage QA data is through identifying patterns in customer feedback. Regularly collected data allows teams to pinpoint specific trends in satisfaction or dissatisfaction, presenting opportunities for actionable change. Predictive QA insights help businesses stay ahead of customers' needs, facilitating timely adjustments to services or products based on empirical evidence. By utilizing this data, companies not only improve customer experiences but also anticipate future demand, ensuring long-term success.
Analyzing QA Data: A Step-by-Step Guide to Predictive Insights
To effectively analyze QA data for predictive insights, itโs crucial to follow a systematic approach. Start by gathering and organizing your QA data, ensuring it is clean and structured for easy access. This foundational step allows you to move forward with confidence. Next, identify key metrics that influence customer satisfaction, such as response times, resolution rates, and customer feedback. These metrics will guide your analysis and help pinpoint factors impacting satisfaction levels.
Employ analytical tools to delve deeper into the data and extract predictive insights. By utilizing these insights, you can forecast customer satisfaction trends and make informed decisions. Additionally, analyzing patterns among different datasets can provide valuable intelligence for enhancing service quality. By following these steps, businesses can transform their QA data into actionable insights, ultimately improving customer satisfaction and fostering loyalty.
- Step 1: Gathering and Organizing QA Data
To effectively begin with Predictive QA Insights, organizations must focus on gathering and organizing QA data. This initial step serves as the foundation for analyzing customer interactions and understanding satisfaction levels. Start by collecting data from various sources, including call recordings, chat transcripts, and customer feedback. Itโs important to ensure that this data is comprehensive and covers diverse customer experiences, as this helps in creating a more accurate picture.
After data collection, the next phase involves structuring and categorizing the information for easy analysis. Proper organization allows for the identification of trends and patterns related to customer satisfaction. It is essential to set up templates and criteria that evaluate the collected data against quantified standards, such as compliance and quality assurance metrics. By doing so, you can derive meaningful insights that inform strategies for enhancing customer satisfaction based on the gathered QA data.
- Step 2: Identifying Key Metrics for Customer Satisfaction
Key metrics for customer satisfaction are essential to understanding how your service or product resonates with users. These metrics can vary widely, encompassing elements like call resolution rates, customer feedback scores, and response times to inquiries. Data collected through Quality Assurance (QA) practices can reveal patterns and insights that lead to improved customer experiences. By precisely tracking these metrics, teams can start to connect the dots between their QA findings and overall customer satisfaction.
To effectively identify key metrics, consider focusing on the following aspects:
Resolution Rate: This metric measures how successfully customer issues are resolved during initial interactions. A high resolution rate often correlates with higher customer satisfaction.
Customer Effort Score: This score reflects how easy it is for customers to get their issues resolved. Lower effort typically indicates an enhanced experience.
Net Promoter Score (NPS): This widely used metric gauges customer loyalty by asking how likely customers are to recommend the service. A higher NPS can be a strong indicator of overall satisfaction.
Average Handling Time: This metric looks at the time taken to resolve customer queries. Shorter handling times can contribute to improved customer satisfaction.
By honing in on these critical metrics, organizations can leverage Predictive QA Insights to fine-tune their offerings and deliver tailored experiences that foster customer loyalty.
- Step 3: Employing Analytical Tools to Derive Predictive Insights
Employing analytical tools to derive predictive insights involves turning raw QA data into actionable knowledge about customer satisfaction. These tools enable organizations to sift through large volumes of data, identifying trends and patterns that signal how customers feel about their products or services. By leveraging various analytics software, businesses can easily pinpoint customer pain points and preferences, leading to more informed decision-making.
To start, the process generally includes several key actions: first, data collection must be thorough and well-organized, ensuring high-quality insights. Next, effective metrics should be identified to measure customer satisfaction comprehensively. Finally, utilizing advanced analytical tools, such as Tableau or Qualtrics, can help visualize data in a meaningful way. These tools not only facilitate understanding customer sentiments but also predict potential future behaviors, making predictive QA insights invaluable for improving overall customer experiences.
Extract insights from interviews, calls, surveys and reviews for insights in minutes
Tools for Harnessing Predictive QA Insights
Effective tools for harnessing predictive QA insights play a vital role in anticipating customer satisfaction. These tools streamline the process of analyzing QA data, making it accessible to all team members, regardless of their technical expertise. With user-friendly interfaces, individuals can swiftly generate reports and identify friction points in customer interactions. This efficiency fosters a proactive approach to quality assurance, enabling businesses to respond promptly to customer needs and expectations.
To enhance predictive analytics, consider utilizing tools such as Zendesk for effective support data management, which can reveal trends in customer satisfaction. SurveyMonkey allows organizations to implement customer feedback loops, capturing insights that inform service improvements. Tableau is instrumental in visualizing QA trends and patterns, facilitating deeper understanding of customer sentiments. Lastly, Qualtrics provides robust data analysis capabilities to uncover valuable customer insights. Together, these tools empower businesses to leverage predictive QA insights strategically, ultimately improving customer satisfaction.
Insight7: Leading the Way in Predictive Analytics
Harnessing Predictive QA Insights signifies a transformative step in understanding consumer behavior. By analyzing customer feedback and quality assurance data, organizations can glean valuable insights that drive decision-making. This predictive approach allows businesses to proactively address potential issues, ensuring customer satisfaction levels are maintained and even enhanced over time.
The framework of predictive analytics involves collecting and processing QA data efficiently. Companies can identify patterns and trends in customer interactions, helping to predict future satisfaction. By incorporating advanced analytical tools, organizations not only streamline their analysis but also gain the ability to turn insights into actionable strategies. As a result, they can stay ahead of market demands and shifting consumer expectations. Adopting predictive analytics furthers a companyโs commitment to enhancing customer experiences and loyalty.
- Overview of Insight7โs features for QA data analysis
Insight7 offers a robust platform designed to streamline QA data analysis, making it accessible for users at any skill level. The interface allows users to effortlessly upload and organize call files, facilitating quick access to critical information. From the homepage, you can begin generating insights or preparing reports without extensive training. This democratization of data encourages team collaboration in understanding customer experiences.
The platform excels in its analytical capabilities, pulling out significant insights such as pain points and customer desires. Each call can be individually analyzed, providing evidence-based summaries and thematic insights. Notably, projects can group multiple calls, enabling broader analysis over large datasets. These features empower businesses to turn raw QA data into predictive QA insights that enhance understanding of customer satisfaction levels. By effectively utilizing these tools, organizations can identify trends and improve overall customer experiences.
Additional Tools to Enhance Predictive Analysis
To drive effective predictive analysis, leveraging additional tools can significantly enhance the quality of Predictive QA Insights. These tools not only facilitate data collection but also allow for easier interpretation of crucial patterns that determine customer satisfaction. By employing industry-leading solutions, teams can transform raw quality assurance data into actionable insights, ultimately leading to better customer experiences.
Key tools that support this process include Zendesk, which harnesses support data to gauge customer sentiment effectively. SurveyMonkey allows organizations to create feedback loops tailored to diverse customer needs. Tableau shines in visualizing QA trends and patterns, making it easier to identify shifts in consumer behavior. Lastly, Qualtrics excels at performing in-depth data analysis, ensuring that businesses can derive comprehensive insights from customer interactions. These tools together create a robust framework for analyzing data and predicting customer satisfaction outcomes, ensuring your organization remains ahead of the curve in understanding consumer needs.
- Zendesk: Leveraging Support Data for Satisfaction
Utilizing support data effectively can offer significant insights into customer satisfaction. Companies can gather various metrics from support interactions to understand how well they are meeting customer needs. Feedback trends can be analyzed to highlight positive and negative experiences, creating opportunities for improvement. With Predictive QA Insights, organizations can uncover valuable patterns from support data, aiding in proactive decision-making.
To maximize the benefits, itโs essential to focus on three key areas: first, gathering a comprehensive dataset, which includes both positive and negative customer responses. Next, identifying critical metrics will provide a clearer picture of customer satisfaction levels. Finally, employing analytical tools can help in translating raw data into actionable insights. By systematically analyzing support data, companies can refine their strategies to enhance customer experiences and drive satisfaction levels higher.
- SurveyMonkey: Implementing Customer Feedback Loops
Implementing customer feedback loops through survey platforms significantly enhances the understanding of client needs and satisfaction. By utilizing feedback collected through surveys, businesses can effectively develop Predictive QA Insights that not only measure current satisfaction levels but also predict future trends. Regularly gathering input allows organizations to identify areas of improvement, ensuring that customer experiences are responsive and tailored to their expectations.
To establish effective feedback loops, organizations must focus on three key elements:
Consistent Survey Distribution: Regularly send out surveys to customers following interactions or purchase completions. This ensures that feedback is timely and relevant, capturing fresh insights.
Targeted Question Design: Craft questions that gauge specific aspects of the customer experience. Use open-ended questions for qualitative insights alongside quantifiable ratings to analyze satisfaction levels.
Data Analysis and Response: Analyze collected data to identify patterns and themes. Itโs crucial to act on this information, demonstrating to customers that their feedback leads to tangible changes.
Incorporating these elements can facilitate a continuous cycle of understanding and improving customer satisfaction.
- Tableau: Visualizing QA Trends and Patterns
Tableau serves as an exceptional tool for visualizing QA trends and patterns, transforming raw data into actionable insights. By presenting data visually, Tableau allows organizations to easily identify trends over time, which can shed light on various quality assurance factors. This visualization process not only highlights consistent metrics but also uncovers outliers or anomalies that could impact customer satisfaction.
Using Tableau, teams can create dashboards that track key performance indicators, facilitating real-time analysis. For example, visualizing positive and negative feedback side by side helps pinpoint areas needing improvement. With the ability to compare datasetsโsuch as files from different locationsโQA teams can detect regional patterns and tailor strategies accordingly. This approach ensures that quality assurance not only reacts to customer feedback but anticipates their needs, ultimately leading to predictive QA insights that enhance customer satisfaction and drive informed decision-making.
- Qualtrics: In-depth Data Analysis for Customer Insights
In the realm of customer insights, Qualtrics offers sophisticated tools for in-depth data analysis, transforming raw QA data into actionable insights. The ability to interpret customer interactionsโsuch as feedback, complaints, and service requestsโallows organizations to recognize patterns in customer behavior. This analytical process can reveal invaluable predictive QA insights, enabling businesses to forecast customer satisfaction levels with greater accuracy.
By systematically analyzing diverse data points, organizations can uncover potential pain points before they escalate. Implementing advanced analytical techniques can help visualize trends and correlations that might otherwise go unnoticed. As businesses harness these predictive QA insights, they can proactively address customer needs, improving engagement and satisfaction. In a competitive environment, utilizing data analysis effectively allows businesses to remain ahead, ensuring they respond timely to evolving customer demands.
Conclusion: Embracing the Power of Predictive QA Insights for Enhanced Customer Satisfaction
The insights gained from predictive QA insights offer organizations a valuable pathway to enhance customer satisfaction. By closely examining the interplay between quality assurance data and customer feedback, businesses can proactively identify trends and address potential issues before they escalate. This shift from reactive to proactive approaches fosters a deeper connection with customers, as their needs are anticipated and met efficiently.
Furthermore, embracing predictive QA insights means implementing robust analytical tools that transform raw data into actionable strategies. Organizations can streamline decision-making processes, improve collaboration among teams, and ultimately create a more satisfying customer experience. In todayโs competitive landscape, harnessing these insights can differentiate companies and position them as leaders committed to customer-centric service.