AI Coaching Evaluation introduces a transformative approach to understanding coaching effectiveness over time. Picture a coach who aims to refine their techniques, yet struggles to gauge progress accurately. With AI's capabilities, we are stepping into a realm where evaluations are not just periodic but are ongoing and data-driven. By harnessing AI technology, coaches can receive insights that are far more precise and actionable.
Understanding AI Coaching Evaluation is essential for coaches seeking to improve their methods. It offers a systematic way to measure performance, ensuring that feedback is relevant and timely. This section will explore how AI can support coaches in refining their practices, ultimately leading to enhanced coaching outcomes and more significant impacts on their teams' performances.
Analyze qualitative data. At Scale.
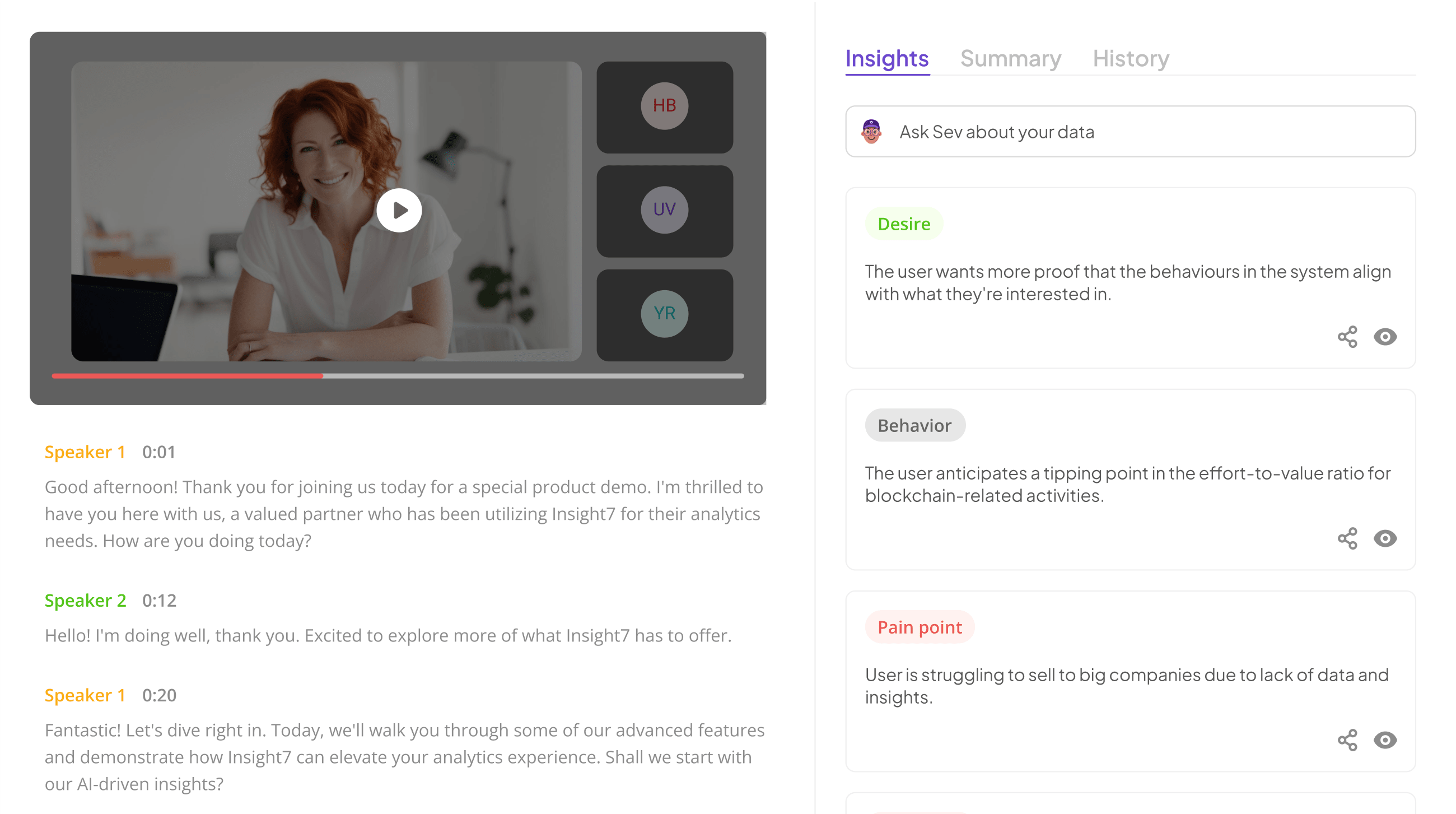
The Evolution of AI Coaching Evaluation Over Time
The evolution of AI coaching evaluation reflects a significant transformation in how coaches measure their effectiveness. In the early years, technology was merely a supplement to traditional coaching methods, primarily limited to analytics and feedback. Progressively, AI systems began integrating more extensive data analysis capabilities, allowing coaches to track performance metrics in real time. This shift has enabled a deeper understanding of individual and team dynamics, fostering a more personalized coaching approach.
Recent advancements have introduced sophisticated algorithms capable of analyzing communication patterns and coaching sessions. AI coaching evaluation now takes into account various factors, including emotional intelligence and client engagement. These advancements help coaches refine their methods and improve client outcomes. By continuously adapting to this evolving landscape, coaches can harness AI to enhance their effectiveness in guiding clients toward reaching their goals.
The Evolution of AI Coaching Evaluation Over Time
AI Coaching Evaluation has dramatically evolved, reflecting advancements in technology and a deeper understanding of coaching dynamics. Initially, evaluation methods were simplistic, often focusing on subjective feedback from individual coaches without robust data backing. As AI began to permeate coaching, algorithms emerged that could assess conversations, coaching styles, and interaction quality based on structured data. This allowed for more objective evaluations, giving insights into coaching effectiveness that were previously unattainable.
Over time, sophisticated AI analytics tools have provided clearer metrics related to engagement and success rates. Moreover, recent developments in natural language processing have enabled AI to analyze coaching sessions in real-time, leading to dynamic feedback for coaches. This transformation has not only enhanced evaluation accuracy but has also encouraged a more consultative approach, engaging coaches in continuous improvement. As AI continues to advance, the methods employed in coaching evaluation will inevitably become more intricate, allowing for nuanced assessments that can significantly influence coaching strategies.
Early Approaches to AI in Coaching
In the early days of AI in coaching, the emphasis was primarily on automating repetitive tasks and data collection. Coaches began to adopt AI systems that could analyze athlete performance based on historical data, enabling them to tailor training programs more effectively. These initial systems laid the groundwork for a more data-driven approach to coaching, aiming to enhance both individual and team performance.
As practitioners began to see the potential of AI Coaching Evaluation, they recognized the importance of specific metrics. Over time, AI tools evolved to provide real-time feedback, pushing the boundaries of how coaches could assess effectiveness. This marked a shift toward more actionable insights derived from data analysis, allowing for continuous improvement in coaching methods. The transition wasnโt just about technology; it was about redefining how coaching could be understood and measured. By focusing on outcomes and performance indicators, AI started to reshape the coaching experience fundamentally.
Assessing how effective coaching is over time requires a deep understanding of the processes and methodologies involved. AI Coaching Evaluation plays a crucial role in this assessment, particularly as it evolves to meet the unique demands of coaches and clients alike. By utilizing sophisticated algorithms and data analytics, AI can provide unbiased feedback on coaching interactions, thus offering an objective lens through which effectiveness can be measured.
Understanding the various dimensions of coaching effectiveness is essential. Key aspects to consider include the clarity of communication, the depth of industry expertise demonstrated, and the ability of the coach to tailor their approach based on individual client needs. As AI continues to advance, it will increasingly enable coaches to refine these elements, leading to enhanced client satisfaction and ultimately better outcomes. By focusing on these areas, AI Coaching Evaluation can greatly improve the coaching process and its efficacy over time.
Recent Advancements in AI Coaching Evaluation
Recent advancements in AI coaching evaluation have transformed how organizations assess coaching effectiveness. AI capabilities have significantly evolved, allowing for enhanced data analysis and personalized feedback mechanisms. With machine learning algorithms, these systems can identify patterns in performance data that human evaluators may overlook. This has led to more comprehensive insights into coaching practices and outcomes.
Furthermore, modern AI solutions now offer real-time feedback during coaching sessions. This immediacy allows coaches to adapt and refine their strategies instantaneously, promoting a proactive approach to coaching development. Additionally, natural language processing capabilities enable AI to analyze communication patterns, providing a deeper understanding of interpersonal dynamics. Consequently, organizations can make informed decisions about coaching effectiveness over time, driving continuous improvement and ensuring alignment with training goals.
Extract insights from interviews, calls, surveys and reviews for insights in minutes
Tools and Techniques for AI Coaching Evaluation
Evaluating AI coaching requires robust tools and techniques that ensure effective assessment over time. These tools can help measure various aspects of coaching performance, including consultative skills, engagement levels, and adherence to established standards. By leveraging data-driven insights, organizations can discern patterns and provide feedback that enhances the coaching experience.
One essential technique involves utilizing AI-powered analytics to score coaching sessions based on predefined criteria. This scoring system can guide coaches on areas needing improvement, promoting a culture of continuous learning. Additionally, employing qualitative analysis through feedback collection can complement quantitative scores, offering a more holistic view of coaching effectiveness. Overall, a combination of these tools and techniques will not only improve individual coaching performance but also foster a more effective coaching environment over time.
insight7: Leading the Charge in AI Coaching
AI coaching is revolutionizing how organizations evaluate coaching effectiveness. By adopting advanced AI coaching evaluation strategies, businesses can unravel insights that were previously difficult to access. This change is crucial in a landscape where timely responses to customer signals can significantly impact competitive advantage. Organizations finding efficient ways to analyze interviews and conversations are well-positioned to drive better coaching outcomes.
Key aspects of AI coaching include automated data analysis, streamlined feedback loops, and enhanced collaboration among teams. Automated data analysis helps organizations process vast amounts of information quickly, enabling them to focus on strategic actions rather than getting bogged down by data management. Streamlined feedback loops empower coaches to adjust their strategies in real-time, fostering a more dynamic coaching environment. Lastly, collaboration tools make it easier for teams to share insights and develop strategies together. Overall, AI coaching acts as a catalyst for sustained improvement in coaching effectiveness over time.
Comparing Insight7 with Other AI Tools
In the realm of AI coaching evaluation, various tools vie for effectiveness and innovation. When comparing different AI tools, it's essential to consider aspects like user experience, data analysis capabilities, and the extent of actionable insights provided. Some tools focus primarily on data mining, while others emphasize real-time feedback to optimize coaching strategies.
One significant advantage of certain AI platforms is their ability to integrate with existing systems, enhancing usability for coaches and organizations alike. For instance, while traditional tools may require manual intervention for data processing, newer AI solutions automate this process, allowing coaches to concentrate on implementing the insights gained. Evaluating AI coaching effectiveness over time hinges on how well these approaches align with the evolving needs of coaching professionals and their clients. Ultimately, selecting the right AI tool can greatly influence the overall coaching experience and outcomes.
Conclusion: The Future of Assessing Coaching Effectiveness With AI
As we look towards the future of AI Coaching Evaluation, it becomes clear that harnessing this technology can reshape how we measure coaching effectiveness. With insightful data and performance metrics, AI can bring a level of objectivity and consistency that traditional methods struggle to achieve. By establishing clear standards and criteria, stakeholders can derive actionable insights, continually refining coaching practices.
Furthermore, the potential for AI to enable personalized coaching experiences is immense. As algorithms learn from each interaction, they can guide coaches on best practices and highlight areas for improvement. This evolution in AI coaching not only enhances the evaluation process but also positions organizations to optimize their coaching strategies for better outcomes.