Intelligent Data Extraction serves as a cornerstone in modern AI data mining practices. With the continuous growth of data generated across various platforms, the ability to intelligently extract relevant information is vital. This capability enhances decision-making processes by transforming raw data into actionable insights, ultimately optimizing business strategies.
The techniques for Intelligent Data Extraction involve using advanced algorithms to analyze and synthesize data from diverse sources. By employing these methods, organizations can efficiently identify patterns, trends, and key metrics that inform their operations. As industries evolve, harnessing robust data extraction techniques is essential for staying competitive in the market.
Analyze qualitative data. At Scale.
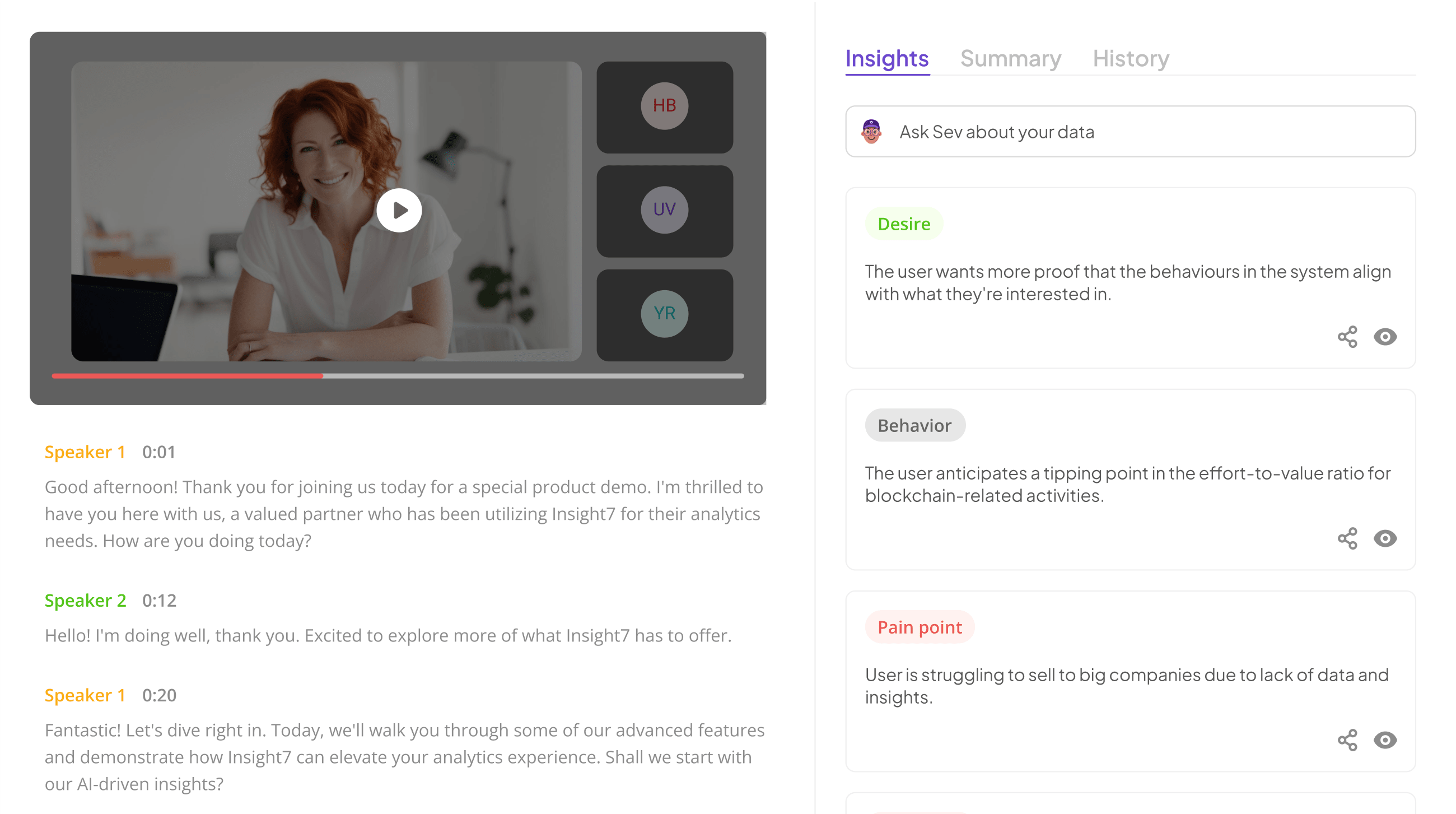
Techniques for Intelligent Data Extraction in AI
Intelligent Data Extraction is a crucial component of AI-powered data mining techniques. It enables organizations to gather and analyze vast amounts of information effectively. By utilizing advanced algorithms and machine learning models, businesses can extract relevant insights from unstructured data, such as audio, text, and images. This transformation from raw data to actionable insights streamlines decision-making processes and enhances strategic planning.
Several techniques enhance Intelligent Data Extraction, starting with natural language processing (NLP), which analyzes human language and extracts meaningful patterns. Additionally, optical character recognition (OCR) identifies text within images, making previously inaccessible data usable. Another technique is data classification, which categorizes information based on predefined criteria, aiding in efficient retrieval. Lastly, sentiment analysis can gauge customer attitudes from textual feedback, providing organizations with essential customer insights. These techniques collectively empower businesses to harness their data efficiently, driving innovation and growth in today's competitive landscape.
Understanding Intelligent Data Extraction
Understanding Intelligent Data Extraction involves unraveling the complexity behind how AI systems process and interpret data. At its core, this technique utilizes advanced algorithms to sift through vast amounts of unstructured information, such as text or audio, to extract meaningful insights. By automating the analysis of this data, organizations can quickly transform raw inputs into actionable outputs that drive decision-making.
The process typically consists of three crucial steps: data acquisition, processing, and output interpretation. Data acquisition involves gathering information from various sources, including call transcripts and user feedback. Following this, processing applies techniques like natural language processing to identify trends and patterns. Finally, output interpretation involves presenting these insights in an understandable manner, enabling teams to address customer needs effectively. Through Intelligent Data Extraction, businesses enhance their analytical capabilities, leading to improved strategic actions.
Steps for Implementing Intelligent Data Extraction in AI Data Mining
Implementing Intelligent Data Extraction in AI Data Mining involves a systematic approach to maximize the utility of your data. First, assess your data sources to determine which data types you wish to extract. This could include structured datasets or unstructured data from calls, emails, or surveys. Next, select an appropriate AI tool that suits your extraction needs, ensuring it supports various data formats for seamless integration.
Once tools are in place, train your AI system to recognize relevant data patterns through machine learning algorithms. This training phase is crucial as it allows the system to identify and extract meaningful insights automatically. Finally, continually monitor and refine the extraction process by evaluating the accuracy of the data extracted. Ultimately, this iterative approach will enhance your Intelligent Data Extraction capabilities, leading to more informed decision-making within your organization.
Tools for Intelligent Data Extraction
In the realm of AI data mining, tools for intelligent data extraction are essential for transforming raw data into actionable insights. These tools streamline the process of acquiring and analyzing data from various sources, allowing businesses to harness valuable information quickly. By effectively automating data extraction, organizations can save time and allocate resources more efficiently, enabling data-driven decision-making.
Several leading tools specialize in intelligent data extraction. First, natural language processing (NLP) tools enhance the capability to extract relevant information from unstructured text. Additionally, machine learning algorithms are employed to identify and predict trends, offering deeper insights into customer behaviors. Cloud-based platforms further provide scalability, allowing teams to collaborate and analyze data in real time. Each of these tools contributes significantly to improving the efficiency and accuracy of data extraction, ultimately empowering companies to make informed decisions based on reliable data.
Extract insights from interviews, calls, surveys and reviews for insights in minutes
Top Tools for AI Data Mining
In the realm of AI data mining, selecting the right tools is crucial for effective intelligent data extraction. These tools vary widely in functionality but share the common goal of transforming raw data into actionable insights. For instance, platforms like RapidMiner and KNIME offer robust capabilities for data manipulation and analysis, enabling users to extract meaningful patterns from vast datasets. Such tools not only streamline the data processing workflow but also enhance the overall accuracy of extracted information.
Another essential tool in this space is Orange Data Mining, which provides a user-friendly interface for visual programming. This can significantly simplify the data mining process, making it accessible to those with limited experience. Weka also stands out with its machine learning algorithms, allowing users to implement predictive modeling and data preprocessing directly. By utilizing these leading tools, organizations can unlock the full potential of their data, ensuring that intelligent data extraction is accurate and effective.
insight7: Your Go-to Solution
Effective data mining hinges on the capability to extract valuable insights from vast amounts of information. Insight7 serves as a premier solution in this realm, addressing the crucial need for Intelligent Data Extraction. By automating and optimizing the extraction process, this platform empowers users to transform raw data into actionable insights swiftly.
This solution enhances the way businesses interact with customer data, particularly through interviews and conversations. Organizations often find themselves overwhelmed by the volume of customer signals generated. Manual analysis can be slow and inefficient, leading to missed opportunities. Insight7 addresses these challenges by streamlining data processing. As a result, teams can focus on understanding customer needs and building strategies that propel them ahead of their competition. Embrace Intelligent Data Extraction to unlock the potential of your data and improve decision-making processes in your organization.
RapidMiner
RapidMiner offers a comprehensive platform designed to facilitate intelligent data extraction through user-friendly interfaces and robust analytical capabilities. Users can easily upload diverse datasets, enabling the exploration and analysis of intricate data relations. By utilizing various algorithms, the platform empowers organizations to derive meaningful insights, which can drive strategic decisions and enhance efficiency.
To successfully implement intelligent data extraction using this tool, consider the following steps:
- Data Preparation: Begin with cleansing and organizing your data to ensure quality and relevance.
- Model Selection: Choose the appropriate machine learning model that aligns with your business objectives.
- Running Analyses: Utilize the platformโs visual analytics tools to explore data patterns and gather insights.
- Interpretation: Analyze the results generated to inform decisions and strategies effectively.
By following these steps, businesses can maximize the value of their data assets through intelligent data extraction techniques tailored to their unique needs.
KNIME
KNIME is a powerful open-source platform aimed at simplifying data analytics and intelligent data extraction. Its user-friendly interface enables users, regardless of their technical expertise, to create complex data workflows effortlessly. This democratization of data processing allows businesses to tap into insights without needing advanced programming skills.
The platform supports a variety of data types, allowing users to visualize and analyze their datasets effectively. KNIMEโs node-based architecture facilitates the integration of different data sources and analytical techniques, streamlining the process of extracting meaningful information. With its ability to handle operations ranging from data preprocessing to advanced machine learning, KNIME stands out as a versatile tool in the realm of AI data mining. Companies can seamlessly evaluate customer interactions, identify pain points, and optimize decision-making processes, driving growth and innovation.
Overall, KNIME exemplifies how intelligent data extraction tools can transform raw data into actionable insights, making it an invaluable asset for any organization looking to enhance its analytics capabilities.
Orange Data Mining
Orange Data Mining is a powerful, open-source tool designed to facilitate visual programming for data analysis. Users can leverage this interactive environment to create data mining workflows efficiently. Its intuitive approach makes it accessible for both beginners and experienced data scientists, enabling quick analysis and insightful reporting.
One of the key features of Orange Data Mining is its extensive library of widgets, which allow users to perform various tasks, such as data preprocessing, visualization, and modeling. This modular design permits seamless integration and adjustment of different components. By effectively utilizing this tool, teams can enhance their strategies for intelligent data extraction, leading to actionable insights in business contexts.
With its rich visualization capabilities, Orange enhances the understanding of complex datasets. Users can easily identify patterns or anomalies, driving informed decision-making based on robust analytics. This makes Orange a vital asset for organizations looking to optimize their data-driven processes significantly.
Weka
Weka is a powerful tool designed for intelligent data extraction in AI data mining. It provides a user-friendly interface that makes data analysis accessible to both experts and novices. Weka offers a collection of machine learning algorithms for data mining tasks, ranging from classification and regression to clustering and association rule mining. This flexibility enables users to analyze diverse datasets quickly and effectively.
To maximize its potential, users can harness Weka's capabilities by following several key approaches. First, data preprocessing is crucial for cleaning and organizing data to enhance analysis accuracy. Second, selecting the right machine learning algorithm is essential for achieving optimal results. Finally, evaluating and interpreting the outputs generated by Weka helps users derive valuable insights from their data. Through these methods, Weka stands out as a vital tool for organizations seeking to implement intelligent data extraction strategies in their operations.
Conclusion: The Future of Intelligent Data Extraction in AI Data Mining
As we look toward the future of intelligent data extraction in AI data mining, advancements will likely redefine how we access and utilize information. Intelligent data extraction techniques are evolving rapidly, harnessing AI's power to automate processes and uncover insights from vast datasets. This transformation can lead to more accurate decision-making and streamlined operations across various industries.
Moreover, emerging tools will enhance the capabilities of intelligent data extraction, making it accessible to organizations of all sizes. By continuing to innovate in this field, businesses will be better equipped to analyze customer feedback, predict market trends, and drive strategic growth. Ultimately, these advancements will shape a more data-driven world, enabling more informed choices and fostering a culture of continuous improvement.