Predictive Brand Sentiment is revolutionizing the way retailers engage with their customers. By analyzing vast amounts of consumer feedback, brands can identify trends that influence purchasing decisions. This proactive approach not only anticipates customer reactions but also aligns marketing strategies with their preferences.
In an increasingly competitive retail environment, understanding consumer sentiment has become paramount. Retailers can leverage insights from predictive brand sentiment analysis to tailor their offerings and improve customer experiences. This section will explore the importance of these insights, highlighting how they can drive brand loyalty and enhance overall business performance.
Extract insights from Customer & Employee Interviews. At Scale.
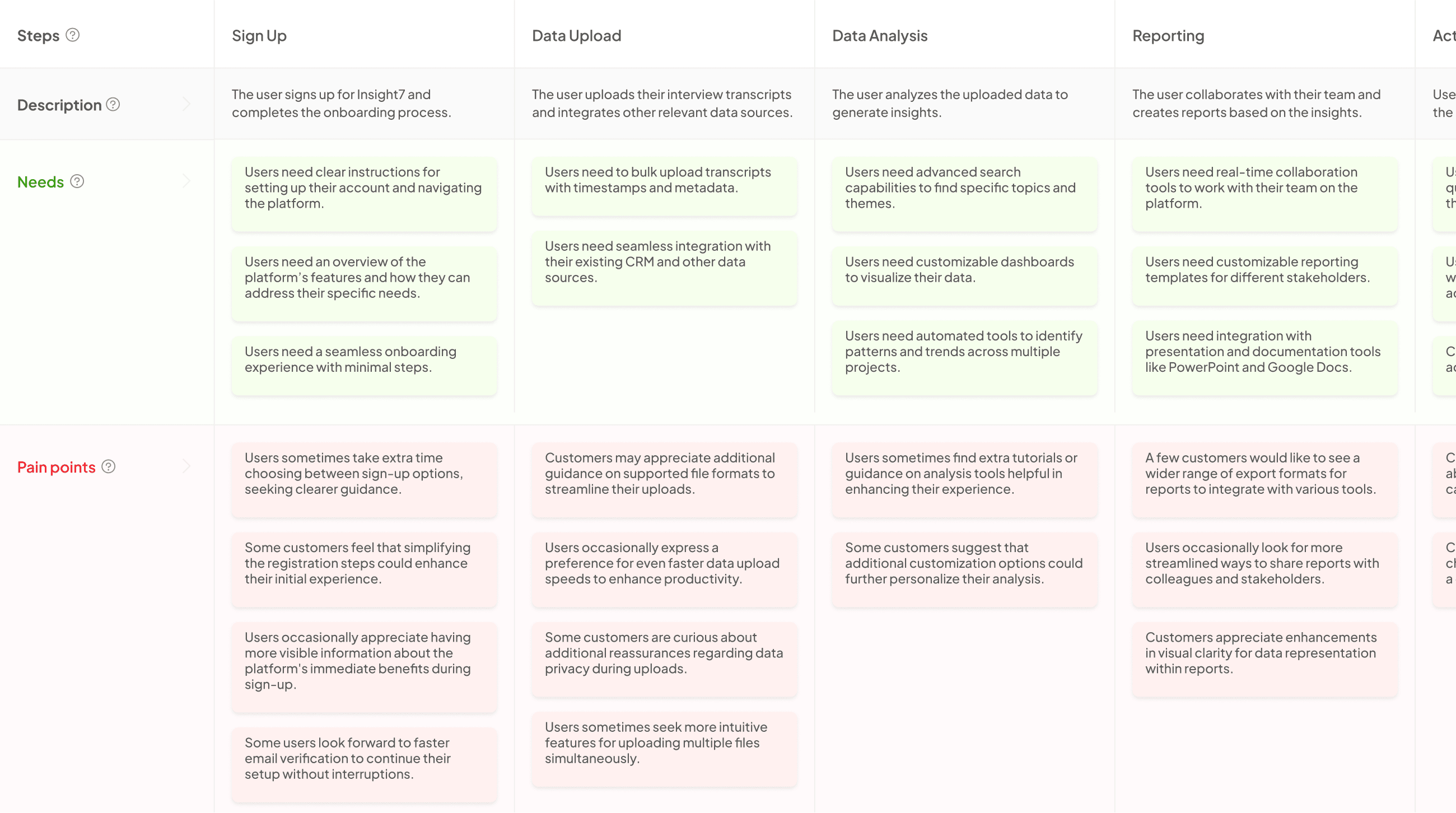
The Role of AI Agents in Retail Sentiment Analysis
AI agents play a pivotal role in the realm of retail sentiment analysis, particularly in enhancing predictive brand sentiment. By sifting through vast quantities of customer feedback, reviews, and social media interactions, these agents can uncover nuanced insights that traditional methods often overlook. This capability allows retailers to understand customer emotions and perceptions, helping them make informed decisions that align with consumer expectations.
The application of AI in sentiment analysis not only streamlines data processing but also enables retailers to react swiftly to changing sentiments. For instance, AI agents can analyze trends in real time, providing brands with actionable insights to adjust their marketing strategies or maintain product quality. Moreover, the continuous learning aspect of these agents ensures that they adapt to evolving consumer behaviors, thereby increasingly refining predictive brand sentiment analysis. In a marketplace where customer opinions significantly influence purchasing decisions, the integration of AI agents offers retailers a competitive edge essential for long-term success.
How AI Agents Work in Predictive Brand Sentiment Analysis
AI agents play a pivotal role in achieving effective predictive brand sentiment analysis. These intelligent systems analyze vast amounts of consumer data, extracting insights about brand perceptions and emotions. By employing natural language processing (NLP) and machine learning techniques, AI agents can identify sentiment trends from customer interactions on various platforms, including social media and reviews. This automated analysis allows retailers to stay ahead of market shifts, understanding how customers feel about their products or services.
To utilize AI agents in predictive brand sentiment, several key components are essential. First, they gather data from multiple sources, ensuring a comprehensive view of consumer sentiment. Next, sentiment models are trained using labeled data to enhance accuracy in sentiment classification. Finally, these models are continuously monitored and refined to adapt to changing consumer behavior. By harnessing the capabilities of AI agents, retailers can effectively manage their brand reputation and make data-driven decisions for future strategies.
Benefits of Using AI Agents for Retailers
Using AI agents in retail offers various advantages, particularly in understanding Predictive Brand Sentiment. First, AI agents can analyze vast amounts of customer data quickly and accurately. This capability helps retailers identify emerging trends and shifts in consumer perceptions, allowing them to adapt their marketing strategies accordingly.
Secondly, the integration of AI agents streamlines the customer feedback process. Retailers can gather insights from multiple sources, including social media, reviews, and customer interactions. By synthesizing this information, they can better understand customer emotions and sentiments surrounding their brands. Ultimately, the use of AI agents not only enhances decision-making but also fosters a proactive approach to maintaining brand reputation in an ever-changing marketplace. Employing these technological advancements invites a new era of informed retail strategies, enabling businesses to resonate with their target audiences effectively.
Generate Journey maps, Mind maps, Bar charts and more from your data in Minutes
Deploying AI Tools for Enhanced Predictive Brand Sentiment
Deploying AI tools for enhanced predictive brand sentiment is essential for retailers aiming to stay ahead in a competitive market. The first step involves comprehensive data collection and preprocessing, which lays the foundation for accurate sentiment analysis. Retailers must gather data from various customer touchpoints, including social media, customer reviews, and direct feedback. This data needs to be cleaned and organized to ensure that insights are reliable and actionable.
Once the data is prepared, the focus shifts to model training and testing. Here, AI algorithms are taught to recognize patterns in sentiment, differentiating between positive, neutral, and negative comments. Continuous monitoring after deployment is crucial to ensure that the model adapts to new data and evolving consumer sentiment. This ongoing adjustment allows retailers to respond proactively to customer opinions, enhancing brand loyalty over time. By strategically deploying these tools, retailers can transform insights into actionable strategies that foster deeper connections with their customers.
Essential Steps for Implementing AI Predictive Sentiment Analysis
To effectively implement AI predictive sentiment analysis, several essential steps must be followed. First, data collection and preprocessing are crucial; gather diverse customer feedback from various sources, including social media, reviews, and surveys. Ensure the data is cleaned and organized to enhance the accuracy of insights derived from it. This foundational step sets the stage for successful analysis of predictive brand sentiment.
Next, sentiment model training and testing should take place. This involves selecting the right algorithms to interpret the data accurately and testing those models to measure their effectiveness. Iterative refinements based on performance metrics will enhance the quality of sentiment predictions. Finally, deployment and continuous monitoring are vital. Once integrated into your systems, regularly track the model’s performance and address any discrepancies to adapt to evolving customer opinions. By adhering to these steps, retailers can harness the power of AI to gauge predictive brand sentiment effectively.
Step 1: Data Collection and Preprocessing
Data collection and preprocessing are crucial initial steps in effective predictive brand sentiment analysis. This process begins with gathering data across multiple channels, such as social media platforms, customer reviews, and survey responses. Diverse data sources help capture varied consumer sentiments, which is essential for building a comprehensive understanding of brand perception.
Once the data is collected, preprocessing occurs to enhance its quality and usability. This step involves cleaning the data by removing duplicates, correcting errors, and dealing with missing values. Additionally, text normalization techniques, such as stemming and lemmatization, ensure that similar sentiments are categorized accurately. By addressing these aspects, brands can obtain reliable insights for predictive brand sentiment analysis, ultimately leading to strategic improvements in their marketing efforts and customer engagement.
Step 2: Sentiment Model Training and Testing
The process of training and testing a sentiment model is pivotal for achieving accurate predictive brand sentiment analysis. At this stage, vast amounts of preprocessed data serve as the foundation for developing robust models. Utilizing supervised learning techniques, the model is trained to recognize and interpret various sentiments, such as positive, negative, or neutral responses. This involves feeding the model labeled data, allowing it to learn patterns and anomalies crucial for accurate predictions.
Once trained, the model undergoes rigorous testing to evaluate its performance against a separate validation dataset. Key metrics, such as precision, recall, and F1 score, are used to measure its effectiveness. By fine-tuning parameters and adjusting the model based on test results, researchers ensure the model can generalize well to new, unseen data. This critical step not only enhances the reliability of predictive brand sentiment analysis but also sets the stage for its eventual deployment in real-time retail scenarios.
Step 3: Deployment and Continuous Monitoring
Deploying AI tools for predictive brand sentiment is a critical phase in maximizing their impact. Once the predictive model is well-crafted, it must be implemented within the retail environment. This deployment can be executed in two primary modes: a self-service interface for users or automated report generation to keep stakeholders updated on sentiment trends. Whichever mode you choose, aligning the system features with user needs ensures usability.
Continuous monitoring plays an essential role in the deployment phase. It allows real-time insights into brand sentiment fluctuations, enabling teams to act swiftly. Regularly updating the model with fresh data not only enhances accuracy but also adapts to changing consumer behaviors. In this way, predictive brand sentiment analysis becomes an ongoing cycle of improvement rather than a one-time event, paving the way for insightful decision-making and strategic marketing adjustments.
Top Tools for Predictive Brand Sentiment Analysis
To effectively conduct predictive brand sentiment analysis, the right tools can make all the difference. These tools help retailers analyze customer opinions, enabling them to adapt quickly to market changes and consumer preferences. Key technologies include advanced AI platforms that parse large datasets, providing insights into positive and negative sentiments expressed by customers. Understanding these sentiments creates opportunities for retailers to improve their strategies.
Among the top tools available are IBM Watson, renowned for its natural language processing capabilities, and Brandwatch, which excels in social media analysis. MonkeyLearn offers user-friendly text analysis capabilities that can be tailored for specific business needs. Additionally, Lexalytics provides robust sentiment analysis solutions that integrate well with existing workflows. These tools not only facilitate data visualization but also empower retailers to make informed decisions based on customer sentiment. By leveraging these technologies, brands can anticipate trends and stay ahead in a competitive landscape.
insight7: Leading the Charge
In the realm of predictive brand sentiment, retailers are beginning to understand the importance of proactive data analysis. AI agents are at the forefront, leading the charge by monitoring customer signals in real-time. These intelligent systems capture insights from various customer interactions, identifying trends that traditional methods might overlook. This capability enables retailers to respond swiftly to shifts in public perception, driving their competitive advantage.
To effectively leverage predictive brand sentiment, retailers must embrace a structured approach. First, they should focus on robust data collection methods that ensure comprehensive insights. Next, training sentiment analysis models with historical data enriches their predictive capabilities. Lastly, continuous monitoring allows businesses to adapt strategies as sentiment evolves. These steps empower retailers to not just react, but to strategically lead their industries in aligning with customer expectations. By harnessing AI, businesses can transform insight into actionable strategies, fostering lasting relationships with their audience.
IBM Watson
In recent years, advanced AI capabilities have transformed how businesses conduct predictive brand sentiment analysis. One such pioneering technology employs robust data processing and machine learning techniques. This AI system analyzes customer interactions, reviews, and social media mentions to derive meaningful insights about consumer sentiment toward brands.
The application of AI in sentiment analysis offers several advantages. First, it increases the speed and accuracy of data interpretation, transforming vast amounts of unstructured information into actionable insights. Second, it empowers retailers to proactively manage their brand image by identifying potential issues before they escalate. Furthermore, these AI solutions help in customizing marketing strategies, ensuring they resonate with consumer sentiments. By tapping into the power of AI, retailers can effectively enhance customer engagement and loyalty, driving sales and success in an increasingly competitive environment.
MonkeyLearn
In the evolving field of predictive brand sentiment analysis, certain AI tools stand out due to their accessibility and functionality. These tools enable retailers to deploy advanced algorithms that interpret customer sentiments effectively. Designed for ease of use, some platforms allow users without specialized training to generate insights from extensive datasets. This democratization of data analysis empowers teams to identify sentiment trends and adjust strategies accordingly.
When utilizing these tools, users can engage with features that systematically extract valuable insights from customer data. For instance, the platform offers functionalities that highlight pain points, needs, and customer compliments. This information culminates in actionable reports that can enhance brand strategies. As the retail industry shifts towards data-driven decision-making, employing predictive brand sentiment tools will refine how businesses connect with, interpret, and respond to their customers. Embracing these innovations ensures that retailers stay ahead in understanding consumer preferences and behaviors.
Lexalytics
Predictive Brand Sentiment analysis is a critical tool for retailers seeking to understand customer perceptions and preferences. It allows businesses to gauge real-time brand health based on customer interactions and feedback. AI-driven platforms offer an intuitive experience by organizing customer data and insights into accessible formats for different teams within an organization.
One prominent approach in this field involves providing various functionalities, such as the ability to generate reports and visualize customer conversations with ease. By analyzing multiple data points, these tools can extract valuable sentiments, pain points, and desires. They empower companies to identify trends and issues promptly, ensuring a proactive response to customer needs. Retailers can thus develop a more tailored strategy based on reliable insights, ultimately fostering deeper connections with their customers and enhancing overall satisfaction. Moreover, this capability significantly aids in refining marketing strategies and improving customer experiences.
Brandwatch
As the retail landscape continues to evolve, understanding consumer sentiment becomes increasingly crucial. The adoption of sophisticated tools plays a vital role in the analysis of predictive brand sentiment, offering insights that can guide marketing strategies and product development. The right tools can help retailers gauge public perception and identify trends that impact brand positioning.
Among available options, specific platforms equip businesses with the capability to track and analyze brand sentiment effectively. These tools utilize advanced analytics and AI algorithms to streamline data analysis. By converting raw data into actionable insights, retailers can improve customer engagement. Moreover, employing a robust system contributes to continuous improvement in brand strategies, ensuring that retailers stay ahead of emerging trends. Integrating these tools allows for effective monitoring of consumer feedback and the ability to respond proactively to shifts in sentiment, ultimately driving success in an ever-competitive market.
Conclusion on AI Agents for Predictive Brand Sentiment Analysis in Retail
The integration of AI agents in predictive brand sentiment analysis revolutionizes how retailers gauge customer emotions and preferences. By harnessing advanced algorithms, these agents can process vast data volumes to identify patterns in consumer sentiment. Retailers can now proactively respond to feedback, enabling better customer experiences and enhanced brand loyalty.
As businesses increasingly rely on predictive brand sentiment insights, they must prioritize effective data management and analysis. This approach not only helps in understanding customer needs but also positions brands to adapt in a rapidly changing retail environment. Moving forward, embracing these technological advancements will be key to staying competitive and relevant in the market.