Predictive Speech Assessment is revolutionizing how organizations interpret and utilize conversational data. Imagine a tool that not only analyzes customer interactions but also anticipates future needs and behaviors. This innovative approach allows businesses to identify trends and improve customer experience significantly, by harnessing the power of speech analytics.
Incorporating predictive speech assessment into analytics tools involves evaluating raw speech data and transforming it into actionable insights. By implementing advanced algorithms, organizations can gain a deeper understanding of their customers’ preferences. Not only does this enhance operational efficiency, but it also drives sustainable growth and strengthens customer relationships over time.
Analyze qualitative data. At Scale.
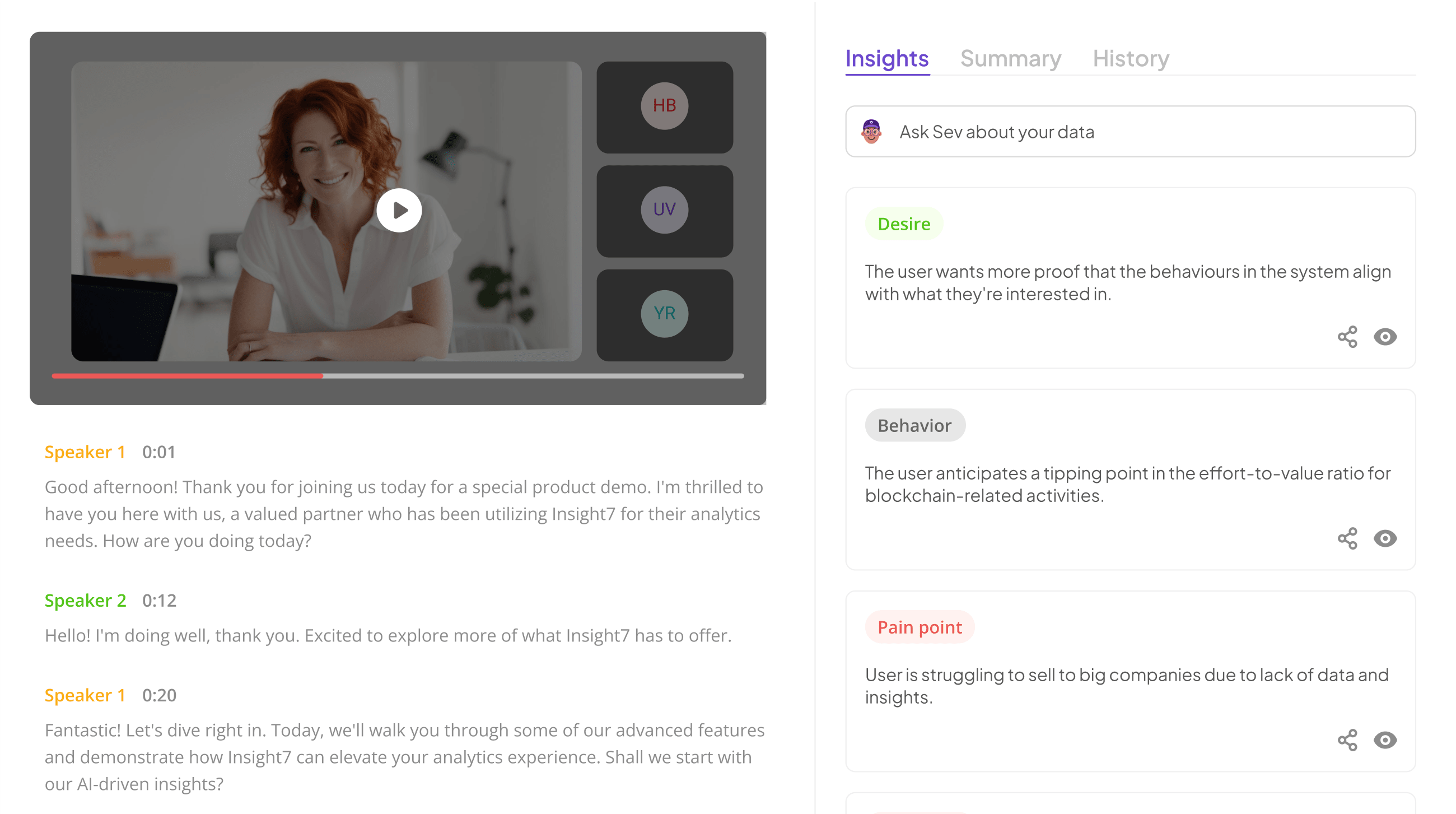
Understanding Predictive Speech Assessment
Predictive Speech Assessment plays a crucial role in the realm of speech analytics, enhancing the ability to analyze spoken interactions. Understanding its components helps illustrate how such tools can forecast outcomes based on historical data. Key elements include data collection, modeling, and performance evaluation, which together form an effective framework for assessing speech interactions.
In practice, Predictive Speech Assessment allows businesses to gain valuable insights into customer behavior and agent performance. By employing advanced algorithms, these tools can analyze thousands of conversations swiftly, identifying trends that may predict customer satisfaction or compliance issues. Incorporating this predictive methodology not only streamlines evaluation processes but also enhances decision-making capabilities, ensuring organizations can respond proactively rather than reactively. As the landscape of customer interaction evolves, the significance of predictive assessment in speech analytics remains vital for continuous improvement.
What is Predictive Speech Assessment?
Predictive Speech Assessment refers to the capacity of speech analytics tools to forecast outcomes based on verbal interactions. This innovative approach leverages advanced algorithms and AI technologies to analyze audio data, enabling organizations to gain insights into communication patterns, customer sentiments, and agent performance. By evaluating these aspects, businesses can predict potential issues, customer satisfaction levels, and the effectiveness of their speech strategies.
The core components of predictive speech assessment include data collection, analysis, and predictive modeling. Through meticulous data gathering and refinement, organizations can develop predictive models that serve as the backbone of their analytics tools. These models provide a framework for understanding the interplay between spoken interactions and desired outcomes, ultimately aiding in informed decision-making. By prioritizing predictive evaluations, companies can enhance operational efficiency, tailor their customer interactions, and maintain higher standards of compliance.
- Definition and importance of predictive evaluation in speech analytics.
Predictive Speech Assessment refers to the process of analyzing speech data to forecast future trends and behaviors. This proactive evaluation plays a pivotal role in the realm of speech analytics by enabling businesses to anticipate customer needs and improve service quality. By integrating predictive capabilities, organizations can identify patterns and develop insights that inform decision-making, ultimately enhancing customer satisfaction.
The importance of predictive evaluation lies in its ability to transform raw data into actionable intelligence. With robust speech analytics tools, companies can evaluate calls against predefined criteria, such as compliance and quality assurance. This systematic assessment empowers organizations to address potential issues before they escalate, ensuring high standards of communication. As a result, predictive speech assessment not only optimizes operational efficiency, but also aligns customer interactions with business goals, thus enhancing overall performance.
- Key components that make up predictive speech assessment.
Predictive Speech Assessment comprises several crucial components that work collaboratively to provide insightful evaluations in speech analytics tools. First, it involves data collection, whereby audio recordings from diverse sources are gathered and processed for analysis. This foundational step ensures that the material is relevant and of high quality, setting the stage for effective assessment.
The next key element is the development of predictive models. These models utilize machine learning techniques to analyze speech data, identifying patterns and trends that reveal customer behaviors and sentiments. Furthermore, an effective evaluation framework is essential. This framework outlines specific criteria for assessing interactions, ensuring that evaluations are objective and comprehensive. Finally, continuous feedback and optimization are vital to enhancing the predictive capabilities. Regularly updating models in response to evolving data ensures sustained accuracy and relevance in assessments. Together, these components form a robust system that enhances the utility of predictive evaluation in speech analytics tools.
How Predictive Speech Assessment Enhances Analytics
Predictive Speech Assessment plays a crucial role in modern speech analytics by transforming the approach organizations take to data analysis. This technology enhances traditional analytics by enabling proactive insights rather than reactive reporting. By employing advanced algorithms, it identifies patterns and predicts future behaviors based on historical speech data, allowing companies to anticipate customer needs more accurately.
One of the key benefits is that organizations can identify training gaps for agents based on predictive evaluation metrics. For instance, by analyzing call interactions, companies can determine which representatives excel in creating rapport or resolving issues effectively. Additionally, the predictive assessment enables tailored coaching strategies, improving both individual and overall team performance. This integration ensures that decision-makers have access to actionable insights that drive continuous improvement, ultimately enhancing customer satisfaction and operational efficiency. Predictive Speech Assessment thus serves as a vital tool for organizations aiming to stay ahead of the curve in competitive markets.
- The role of predictive modeling in improving speech analytics.
Predictive modeling plays an essential role in refining speech analytics by enabling organizations to foresee trends and improve customer interaction outcomes. Through Predictive Speech Assessment, businesses can identify emerging patterns within spoken data, which influences decision-making strategies. By harnessing historical data, predictive models can help organizations understand customer sentiment and predict future behaviors.
By integrating advanced machine learning techniques, predictive modeling analyzes large volumes of speech data effectively. This allows companies to optimize their customer experiences by tailoring responses to anticipated needs. Furthermore, the insights derived from predictive assessments can highlight training opportunities for staff, leading to enhanced performance. The ultimate goal is to transform raw audio into actionable insights, promoting a continuous improvement cycle that benefits both the organization and its customers.
- Benefits of incorporating predictive assessment into analytics tools.
Incorporating predictive assessment into analytics tools brings numerous advantages. Firstly, it enhances the accuracy of evaluations by identifying patterns and trends in speech data. This allows for better forecasting of customer satisfaction and sentiment based on historical interactions. By leveraging predictive speech assessment, businesses can anticipate customer needs, enabling more proactive service improvements.
Additionally, such assessments facilitate targeted strategies based on insightful data. It helps in tailoring interactions to specific customer segments, enhancing engagement and satisfaction. This proactive approach fosters loyalty and retention by addressing potential issues before they escalate. Moreover, predictive assessment can streamline operations, optimizing resource allocation and training needs. Overall, embracing predictive speech assessment cultivates a more data-driven, customer-centric environment, leading to greater organizational success and improved performance.
Extract insights from interviews, calls, surveys and reviews for insights in minutes
Implementing Predictive Evaluation in Speech Analytics
Implementing predictive evaluation in speech analytics requires a structured approach to enhance insights drawn from interactions. First, data collection and preparation are crucial. Organizations must gather high-quality audio recordings that represent various scenarios. Preprocessing involves cleaning this data to ensure accuracy before analysis. This step forms the foundation for robust predictive speech assessment.
Next, modeling and analysis take center stage. Here, predictive models are developed using machine learning techniques on the processed speech data. These models provide insights into patterns and trends, offering a predictive angle to performance metrics. Lastly, evaluation and optimization are essential. Continuous testing of model accuracy helps refine the insights generated, making them more actionable over time. By following these steps, businesses can implement predictive evaluation effectively, leading to improved outcomes in customer interactions and overall performance.
Steps to Incorporate Predictive Assessment
To effectively incorporate predictive assessment into speech analytics tools, the initial step involves data collection and preparation. High-quality audio data is crucial for meaningful analysis, so identifying the right sources is essential. Once collected, this data must undergo preprocessing to remove noise and ensure clarity, making it ready for analysis.
The next phase is modeling and analysis, where predictive models are developed using the collected speech data. This often involves applying machine learning techniques that enhance the ability to predict trends and identify patterns within the data. Once models are built, evaluation and optimization come into play. Testing for accuracy ensures the model’s reliability, while ongoing optimization is necessary to adapt to new data and improve predictive capabilities continuously.
By following these structured steps, organizations can successfully integrate a predictive speech assessment framework that enhances their analytics tools. This process not only enriches their understanding of customer interactions but also drives strategic improvements.
Step 1: Data Collection and Preparation
The journey of predictive speech assessment begins with a critical phase: data collection and preparation. This step lays the foundation for any effective analysis, as high-quality audio data is integral to achieving accurate insights. First, it is essential to gather audio recordings from various sources, ensuring that they represent a wide range of customer interactions. This diverse dataset provides a comprehensive view of speech patterns and customer sentiments.
Following data collection, the next challenge is preprocessing the audio data. This involves cleaning the recordings to remove background noise and irrelevant information. Techniques for organizing and formatting the data include transcribing conversations to text and tagging key attributes relevant to analysis. By ensuring that the data is well-prepared, analysts can lay the groundwork for robust predictive modeling that will enhance overall speech analytics tools. Well-prepared data not only speeds up analysis but also significantly increases the reliability of predictive outcomes derived from speech analytics.
- Gathering high-quality audio data.
Gathering high-quality audio data is essential for effective predictive speech assessment in speech analytics tools. It begins with selecting the right recording environments where external noise is minimized. Clear, undistorted recordings form the backbone of analysis, enabling accurate insights into customer interactions. Furthermore, the choice of recording equipment significantly influences audio quality. High-fidelity microphones and appropriate settings ensure that subtle vocal nuances are captured for more reliable assessments.
After gathering audio data, preprocessing is crucial. This involves cleaning the audio files to remove any background noise and enhancing the clarity. Ensuring that the data is well-organized and labeled allows for efficient analysis and facilitates the training of predictive models. By prioritizing these steps, businesses can significantly enhance the accuracy of their speech analytics. In turn, this allows organizations to utilize predictive evaluation effectively, driving better customer engagement and optimizing overall service quality.
- Preprocessing and cleaning for analysis.
Effective preprocessing and cleaning of audio data are crucial steps in predictive speech assessment. Initially, raw audio recordings must undergo transcription, converting spoken words into text for easier analysis. This transcription should accurately capture every nuance of the conversation, ensuring the dataset reflects true speaker intent and sentiment.
Following transcription, it is essential to clean the data to eliminate any irrelevant information or noise. This involves removing filler words, background noise, and any inaccuracies that may interfere with analysis outcomes. By organizing and categorizing this clean data, analysts can significantly enhance the predictive capabilities of speech analytics tools. The cleaner the data, the more reliable patterns and insights generated from predictive speech models will be, ultimately leading to better decision-making based on the analysis.
Step 2: Modeling and Analysis
Modeling and analysis are crucial steps in predictive speech assessment, serving as the bridge between raw data and actionable insights. This phase involves developing predictive models that utilize speech data to uncover patterns and trends. By leveraging advanced machine learning techniques, organizations can analyze various aspects of this data, such as customer sentiment and feedback frequency.
During this process, it’s essential to utilize visual data representations to make complex information more digestible. Analysts can embark on queries that explore both positive and negative feedback across datasets, revealing underlying trends that inform strategic decisions. As these insights emerge, they provide a foundation for continuous optimization, enabling businesses to refine their approaches and enhance customer experiences effectively. Predictive speech assessment not only aids in immediate analytics but also drives long-term operational improvements, fostering a proactive approach to customer engagement.
- Developing predictive models using speech data.
Developing predictive models using speech data is a crucial aspect of advancing speech analytics tools. The process begins with collecting and preparing high-quality audio data, which serves as the foundation for effective predictive speech assessment. Ensuring the data is clean and relevant directly influences the model's accuracy and reliability.
Once the data is prepared, machine learning techniques come into play. These techniques facilitate the creation of robust predictive models that can analyze patterns and trends within speech data. The resulting insights not only help organizations enhance decision-making but also improve customer interactions. By continuously testing and optimizing these models, businesses can ensure they consistently deliver accurate predictive evaluations, significantly impacting strategic outcomes. This structured approach transforms raw speech data into valuable insights, ultimately driving business performance forward.
- Applying machine learning techniques.
Machine learning techniques play a crucial role in predictive speech assessment, a pioneering approach that enhances the interpretation of spoken language data. By utilizing sophisticated algorithms, these techniques can analyze vast amounts of voice samples to generate actionable insights. For instance, one might train models to identify positive and negative sentiments expressed in customer calls. This ability to dissect emotional undertones allows businesses to refine their customer interactions significantly.
Moreover, machine learning facilitates the continuous optimization of predictive models. As new data is incorporated, these algorithms learn and adapt, ensuring accuracy over time. Additionally, validation techniques help assess model performance, leading to better reliability in predictions. The integration of machine learning elevates predictive speech assessment, making analytics tools more effective in understanding customer behavior and improving service quality.
Step 3: Evaluation and Optimization
Evaluation and optimization are crucial in predictive speech assessment. At this stage, organizations analyze the performance of their speech analytics tools to ensure they are meeting the intended objectives. Data derived from evaluations guides refinements in the assessment tools, enhancing their overall effectiveness. Organizations can look for specific metrics, such as accuracy in understanding customer intent and the ability to adapt to changing speech patterns, during this evaluation phase.
To optimize performance, organizations should consider three key factors:
Model Accuracy: Regular testing against real-world scenarios helps maintain the reliability of predictions. Adjustments may be required to ensure accurate results.
Feedback Integration: Incorporating user feedback can refine models and bring crucial insights that can lead to even better predictions in future assessments.
Continuous Improvement: Predictive analytics is an evolving field. Staying updated with the latest algorithms and technologies is essential for ongoing optimization in the predictive speech assessment processes. By systematically evaluating and optimizing these elements, organizations can significantly enhance their speech analytics outcomes.
- Testing model accuracy and reliability.
Testing model accuracy and reliability is a crucial phase in the predictive speech assessment process. This step ensures that the models used to analyze speech data yield actionable and trustworthy insights. It involves comparing the model's predictions against actual outcomes to determine its effectiveness and precision.
There are several key considerations during this testing phase. First, the model should be validated using varied datasets to ensure it performs well across different contexts and scenarios. Next, statistical measures like precision, recall, and F1-score are essential in assessing performance. Continuous monitoring is also vital; as new data comes in, the model may require adjustments to maintain accuracy. Ultimately, ensuring model reliability contributes to producing valuable insights that can drive decision-making in organizations.
- Continuous optimization for improved outcomes.
Continuous optimization plays a crucial role in enhancing outcomes within predictive speech assessment. By systematically evaluating performance metrics, organizations can identify areas of improvement, allowing for the refinement of speech analytics tools. Each call analyzed contributes valuable insights into customer interactions, enabling the system to evolve based on real-world feedback.
To facilitate continuous optimization, consider the following steps:
Regular Data Review: Consistent analysis of collected audio data ensures up-to-date evaluations. Monitoring trends over time can help track improvements and shifting customer needs.
Incorporating Feedback Loops: Engaging customer service representatives and other stakeholders in sharing insights helps create a more responsive predictive model. Their experiences can unveil crucial questions often overlooked.
Predictive Adjustments: Utilize predictive insights to inform training and refine operational practices. This not only aligns the team with customer expectations but also enhances overall service quality.
By embedding these practices into the routine evaluation process, organizations can expect significant advancements in their predictive speech assessment capabilities, resulting in improved outcomes.
Top Tools for Predictive Speech Assessment
In the realm of predictive speech assessment, selecting the right tools is crucial for effective analytics. Various technologies can help organizations analyze and derive insights from speech data. One widely recognized tool is CallMiner, which specializes in voice analytics to accurately predict customer satisfaction. By capturing spoken interactions, it assesses sentiment and helps improve customer experiences.
Another prominent option is Verint Speech Analytics, which not only provides predictive insights but also enhances operational efficiency. This tool offers features that identify trends and potential issues, allowing organizations to make proactive adjustments. NICE Nexidia stands out with its comprehensive approach to predictive and real-time analytics, enabling teams to react swiftly to changing customer needs. These tools exemplify how predictive speech assessments can significantly improve understanding of customer behavior and refine engagement strategies.
- Insight7: Leading tool for integrated speech predictive evaluation.
Insight7 stands out as a leading tool for integrated speech predictive evaluation. This innovative platform transforms how organizations analyze customer conversations by turning traditionally manual processes into efficient, automated evaluations. Such predictive speech assessment enables businesses to collect and interpret vast quantities of data quickly and effectively.
The core functionality of Insight7 hinges on leveraging advanced analytics to derive actionable insights. It allows companies to dig deeper into customer interactions, ensuring they can effectively address customer needs and preferences. By integrating predictive models, Insight7 not only improves the speed of analysis but also enhances the accuracy of insights drawn from speech data. This results in a dynamic approach to understanding customer satisfaction and refining strategies accordingly. As competition intensifies, using Insight7 can provide businesses the edge they need to proactively respond to their markets.
- CallMiner: Uses voice analytics to predict customer satisfaction.
Voice analytics technology plays a crucial role in predicting customer satisfaction by analyzing speech patterns and responses during calls. Through advanced algorithms, the technology identifies key indicators of customer sentiment and engagement. By focusing on the customers' tone, word choice, and questions, it provides invaluable insights into how well their needs are being addressed.
Incorporating predictive speech assessment enables businesses to evaluate the effectiveness of their customer service representatives in real time. This predictive approach not only helps in understanding customer satisfaction levels but also assists in refining training programs for staff. By tracking specific metrics across numerous calls, organizations can ensure a consistent quality of service, ultimately leading to enhanced customer experiences and loyalty. The power of voice analytics in driving actionable insights cannot be underestimated in today’s competitive business environment.
- Verint Speech Analytics: Offers predictive insights to improve operations.
Verint Speech Analytics offers predictive insights that significantly enhance operational efficiency. By harnessing the power of data, this tool enables businesses to identify key trends in customer interactions and behavior. Predictive Speech Assessment allows organizations to move beyond historical data, anticipating future needs and preferences based on past conversations. This proactive approach leads to improved decision-making and optimized customer experiences.
The platform streamlines data access, allowing users without advanced training to generate meaningful insights effortlessly. It features robust analytics capabilities designed to extract vital information, such as pain points and customer desires, from recorded interactions. As companies utilize these insights, they can refine their strategies and enhance service delivery, ultimately driving customer satisfaction. By adopting a forward-thinking stance with predictive analytics, businesses are better equipped to meet present and future challenges in their operations.
- NICE Nexidia: A comprehensive tool for predictive and real-time analytics.
NICE Nexidia serves as a robust platform, transforming the way organizations approach predictive speech assessment. With its capability for real-time analytics, businesses can quickly glean insights from audio data. This functionality allows for the timely identification of patterns and trends, essential for effective decision-making.
The tool excels in managing call projects by streamlining data collection and analysis. Users can easily upload audio files, access transcription services, and organize calls into manageable projects. This facilitates in-depth exploration of customer conversations, revealing both challenges and opportunities. By employing predictive modeling, Nexidia enhances the understanding of customer sentiments, thus enabling proactive strategies for improvement. In essence, it empowers organizations to make informed, data-driven decisions that align closely with customer needs and expectations.
- Interactive Intelligence: Known for innovative speech prediction capabilities.
Interactive intelligence tools are transforming how organizations evaluate speech analytics, particularly through their innovative predictive speech assessment capabilities. These tools utilize advanced algorithms to analyze spoken interactions, providing insights that exceed traditional evaluation methods. The predictive capabilities allow businesses to not only understand past customer interactions but also anticipate future customer needs and behaviors based on speech patterns.
In practical applications, this means organizations can streamline quality assurance processes and enhance customer engagement strategies. By assessing speech data, these tools can generate detailed reports on agent performance, pinpoint strengths and weaknesses, and make real-time recommendations for improving communication skills. Ultimately, the integration of predictive speech assessment into analytics offers businesses a powerful way to enhance their service delivery and maintain a competitive edge in the market.
Conclusion on Predictive Speech Assessment
Predictive Speech Assessment serves as a cornerstone in enhancing the effectiveness of speech analytics tools. By evaluating and predicting communication trends, organizations can gain valuable insights into agent performance and customer interactions. This process focuses on identifying patterns within speech data, which helps enhance decision-making and operational efficiency.
Moreover, incorporating predictive assessment allows businesses to tailor their training programs based on identified strengths and weaknesses among team members. As a result, companies can foster better customer relations and improve compliance outcomes. Ultimately, embracing Predictive Speech Assessment equips organizations with the intelligence needed to stay ahead in a competitive landscape.