In the realm of modern business, maintaining high standards of quality monitoring is more crucial than ever. AI-Enhanced Quality Monitoring transforms traditional evaluation methods by incorporating advanced speech recognition technology. This integration allows organizations to analyze communication patterns effectively and derive insights that drive operational improvements.
As companies seek to enhance their performance metrics, the role of speech recognition becomes increasingly significant. By automating the quality monitoring process, businesses can identify strengths and weaknesses in real-time. Consequently, organizations gain invaluable feedback that informs training and development efforts, ultimately fostering a culture of continuous improvement. Embracing AI-Enhanced Quality Monitoring sets the foundation for a more efficient and insightful operational framework.
Analyze & Evaluate Calls. At Scale.
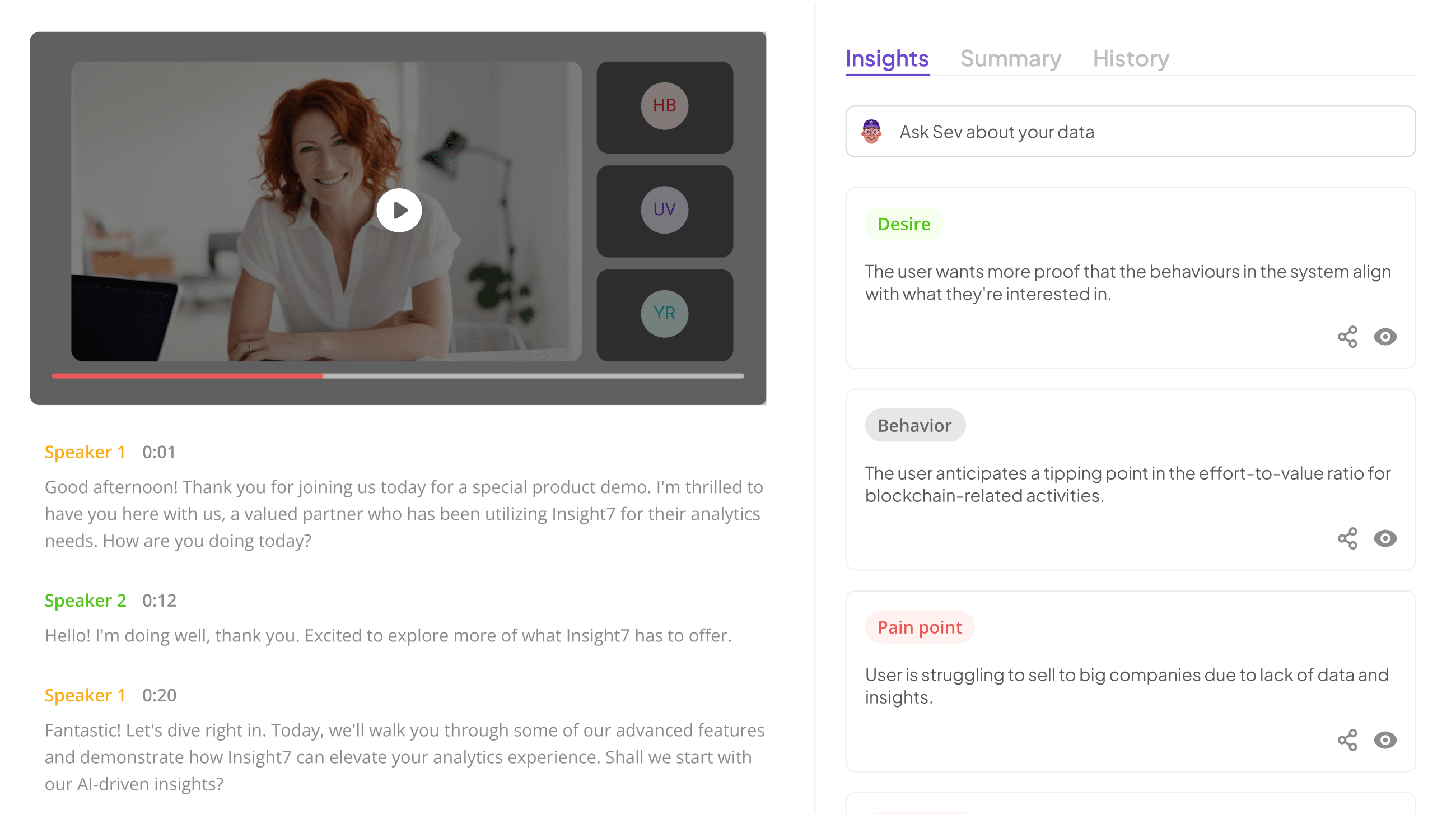
The Role of AI-Enhanced Quality Monitoring in Speech Recognition
AI-Enhanced Quality Monitoring plays a transformative role in the realm of speech recognition. By utilizing advanced algorithms, it allows organizations to analyze spoken interactions with a level of detail previously unattainable. This technology enables the identification of speaker dynamics, contextual nuances, and compliance metrics, thus offering valuable insights into communication effectiveness.
One of the key benefits of AI-Enhanced Quality Monitoring is its ability to significantly enhance accuracy and efficiency in quality assessments. Traditional methods often fall short due to human error and inconsistency. In contrast, AI provides real-time analysis and objective reporting, ensuring that quality monitoring is both reliable and scalable. This capability empowers businesses to refine their processes and improve overall performance, ultimately leading to better customer experiences and more informed decision-making.
Understanding AI-Enhanced Quality Monitoring
AI-Enhanced Quality Monitoring is transforming how organizations evaluate and improve their service quality. This innovative approach utilizes advanced algorithms to analyze conversations in real time, allowing businesses to identify performance trends, compliance issues, and training opportunities. By automating the monitoring process, companies can save time and resources while achieving more consistent evaluations of agent interactions.
Through speech recognition technology, AI can discern various elements within a conversation, such as sentiment, keywords, and even engagement levels. This information helps businesses streamline their quality assurance processes, enabling them to provide actionable insights more quickly. The integration of AI-Enhanced Quality Monitoring results in greater operational efficiency, ultimately leading to improved customer satisfaction and outcomes. Embracing this technology paves the way for continuous improvement in service delivery.
- Explanation of AI-enhanced quality monitoring and its significance in todays technological landscape.
AI-Enhanced Quality Monitoring marks a pivotal shift in how organizations evaluate service interactions. By implementing advanced speech recognition technology, businesses can now analyze conversations with unprecedented accuracy. This capability allows for the tracking of performance metrics, compliance adherence, and customer engagement in real-time, significantly enhancing quality assessment processes.
The significance of AI-Enhanced Quality Monitoring extends beyond efficiency; it fosters informed decision-making, reduces human error, and promotes a culture of continuous improvement. As organizations navigate the complexities of customer interactions, the insights derived from AI-driven analyses empower teams to refine their strategies. With immediate access to detailed performance reports, leaders can swiftly identify areas for enhancement and ensure that standards are not just met but exceeded, ultimately leading to a superior customer experience. In todayโs fast-moving digital era, adopting these technologies is not just beneficial; it is essential for staying competitive.
- The impact of AI on improving accuracy and efficiency in quality monitoring processes.
AI-Enhanced Quality Monitoring significantly transforms quality monitoring processes by improving accuracy and efficiency. The integration of artificial intelligence allows for the precise analysis of spoken content, minimizing human error. Traditional methods often struggle with subjective evaluations, but AI systems can systematically assess performance criteria based on objective data. This shift leads to quicker identification of training gaps and enhanced feedback loops for agents.
One prominent benefit of AI is its ability to process large volumes of data rapidly. AI systems can analyze numerous calls within minutes, providing actionable insights that were previously unattainable. For example, organizations can generate detailed performance reports that rank agents and highlight areas needing improvement. By utilizing AI-Enhanced Quality Monitoring, businesses not only streamline their monitoring processes but also elevate the overall quality of their customer interactions, ultimately fostering a culture of continuous improvement.
Key Components of AI Speech Recognition Technology
AI speech recognition technology operates through a combination of sophisticated algorithms and linguistic analysis. At its core, natural language processing (NLP) enables machines to understand, interpret, and respond to human speech. This capability is crucial for AI-enhanced quality monitoring, as it allows systems to accurately transcribe conversations and identify key insights.
Two essential components drive this technology: acoustic modeling and language modeling. Acoustic modeling focuses on transforming sound waves into linguistic units, capturing the nuances of spoken language. In contrast, language modeling utilizes probability to predict the sequence of words, enhancing the system's ability to understand context and intent. Together, these components significantly improve the accuracy and efficiency of quality monitoring processes. With advancements in AI, businesses can harness speech recognition technology to automate feedback loops, track agent performance, and refine overall service quality.
- How AI speech recognition works.
AI speech recognition technology transforms spoken language into text, enabling more efficient quality monitoring processes. The technology typically employs algorithms to convert audio signals into words by analyzing sound waves and patterns. This process begins with capturing voice data, which is then digitized for analysis. Sophisticated models learn from vast amounts of data, allowing them to recognize speech variations, accents, and context. As a result, this enhances AI-Enhanced Quality Monitoring, providing real-time insights into agent performance.
Natural language processing (NLP) plays a critical role in this technology, allowing the AI to understand and interpret meaning beyond mere words. Coupled with machine learning, NLP enables the system to improve accuracy over time as it processes more conversations. Ultimately, AI speech recognition not only streamlines the evaluation of calls but also empowers organizations to gain valuable insights that can drive training and performance improvements in customer interactions. This synergy facilitates a more reliable and efficient quality monitoring system.
- The importance of natural language processing (NLP) in speech recognition.
Natural Language Processing (NLP) is essential for enhancing the effectiveness of speech recognition systems, especially in AI-enhanced quality monitoring. NLP allows machines to understand, interpret, and respond to human language more naturally, bridging the gap between spoken words and digital processing. This capability is crucial, as speech recognition technology increasingly needs to grasp context, nuances, and intent behind words spoken in real-world scenarios.
In AI speech recognition, NLP transforms raw audio data into meaningful insights by analyzing the semantics and structure of language. It empowers systems to identify not just the words being said but also their significance, ensuring more accurate assessments of interactions. The integration of NLP enables businesses to derive actionable insights from conversations, thereby improving compliance and enhancing the customer experience through targeted quality monitoring efforts. By utilizing NLP, organizations can ensure their quality measures align with customer expectations while optimizing engagement strategies.
Extract insights from interviews, calls, surveys and reviews for insights in minutes
Implementing AI-Enhanced Quality Monitoring With Speech Recognition
To implement AI-enhanced quality monitoring with speech recognition, itโs important to focus on integration and precision. Begin by assessing your current capabilities and determining the specific quality monitoring needs. Understanding the operational gaps will help tailor your approach. Following this assessment, select the most suitable AI tools that align with your requirements. This step is crucial as different technologies provide varying levels of accuracy and features.
Training the AI systems is equally vital for effective quality monitoring. Utilize relevant datasets to enhance the AI's contextual understanding of speech patterns and variations. This training phase will ensure that the system can accurately identify speakers and assess their performance. Ultimately, a well-implemented AI-enhanced quality monitoring system not only streamlines processes but also provides valuable insights that contribute to organizational improvement. Regular reviews and updates of the system will further enhance its effectiveness.
Steps to Integrate AI Speech Recognition
Integrating AI speech recognition into your quality monitoring processes begins with a clear understanding of your current capabilities. Step 1 involves assessing what you already have in place for monitoring, such as existing software, team skills, and procedural workflows. Identifying gaps will help you determine where AI can enhance your quality monitoring efforts.
Next, Step 2 focuses on choosing the right AI tools and technologies. There are many options available, and selecting a system that aligns with your specific needs is crucial. Consider factors such as accuracy, ease of integration, and adaptability to your unique operational context when making your choice.
Step 3 requires you to train the AI systems with high-quality datasets to achieve optimal performance. This training helps improve the accuracy of the AI-enhanced quality monitoring processes, ensuring that the insights generated are reliable and actionable. With these steps, organizations can effectively leverage AI speech recognition for more efficient and precise quality monitoring.
- Step 1: Assessing current quality monitoring needs and capabilities.
Understanding your current quality monitoring needs is the first crucial step in enhancing your processes with AI speech recognition. Begin by evaluating existing capabilities and identifying gaps that AI-enhanced quality monitoring can potentially fill. This evaluation should focus on key aspects, such as compliance requirements, call evaluation standards, and performance metrics that matter most to your organization.
Next, define the criteria for assessing quality. This can involve establishing a scoring system based on qualitative and quantitative measures. Consider customer feedback, efficiency of call handling, and adherence to compliance guidelines as core elements in your assessment. By laying a solid foundation in understanding your specific needs and capabilities, you can properly align AI speech recognition tools to support your goals and enhance the overall effectiveness of your quality monitoring strategy.
- Step 2: Choosing the right AI tools and technologies for speech recognition.
When choosing the right AI tools and technologies for speech recognition, it's crucial to consider specific features and capabilities. Start by analyzing the accuracy levels these tools provide. High accuracy in transcribing speech ensures more reliable data for AI-Enhanced Quality Monitoring. Also, evaluate whether the tool supports multiple languages and dialects, as this can enhance user experience and engagement.
Next, assess the integration capabilities of the tools with existing systems. Seamless integration with your current applications allows for a smooth workflow and better data management. Look for tools that offer customization options, enabling adjustments based on your unique quality monitoring requirements. Additionally, consider the scalability of the solution. As your monitoring needs evolve, your selected technology should adapt to growing demands without compromising performance. Prioritize user-friendly interfaces that facilitate quick adoption by your team, enhancing overall productivity.
- Step 3: Training AI systems with the right data sets for accurate monitoring.
Training AI systems with the right datasets is crucial for achieving effective AI-Enhanced Quality Monitoring. Selecting diverse and relevant datasets helps the system recognize various accents, dialects, and industry-specific jargon. This crucial step ensures the AI can accurately discern and classify the nuances of speech in calls, leading to improved insights into agent performance.
Moreover, continuously updating the training sets with real interaction data enhances the model's effectiveness. An iterative training process, where feedback is incorporated to refine the system, helps maintain accuracy over time. Creating a feedback loop that includes review and assessment of AI-generated results allows for ongoing adjustments. With well-trained AI, businesses can gain deeper insights into customer interactions, ultimately leading to enhanced service quality and client satisfaction.
Top Tools for AI Speech Recognition
When seeking top tools for AI speech recognition, it's essential to consider those that significantly enhance AI-Enhanced Quality Monitoring. Each tool listed below has unique strengths, making it easier for organizations to improve their quality assurance processes.
Google Speech-to-Text: This tool delivers exceptional accuracy and supports numerous languages, making it highly adaptable. Its ability to transcribe real-time audio significantly boosts efficiency in monitoring interactions.
IBM Watson Speech to Text: Renowned for its robust analytics capabilities, this tool can identify speaker nuances and context. This insight helps organizations focus on areas needing improvement.
Amazon Transcribe: Offering advanced deep learning capabilities, Amazon Transcribe provides real-time speech recognition. Its ability to generate textual data from conversations enables detailed compliance assessments.
Microsoft Azure Cognitive Services: This suite includes various APIs designed for custom solutions. Its versatility allows organizations to tailor tools specifically for their quality monitoring needs.
Insight7: Known for its seamless integration into existing services, Insight7 enhances the user experience. The tool offers detailed reports to assist in making data-driven decisions.
Utilizing these tools can revolutionize monitoring practices through effective AI speech recognition, driving significant gains in quality and compliance.
- Insight7: Leading tool for integrating AI in quality monitoring.
Insight7 stands out as a leading tool for integrating AI in quality monitoring. Its robust platform enables businesses to analyze large volumes of customer interactions seamlessly, enhancing the process of deriving actionable insights. By utilizing AI-Enhanced Quality Monitoring, organizations can gain a competitive edge in understanding customer needs, preferences, and pain points more effectively than ever before. The self-service nature of Insight7 simplifies the data analysis process, allowing teams to focus on strategic decision-making.
This tool not only speeds up insights generation but also fosters collaboration among stakeholders. With traditional monitoring methods falling short in keeping pace with the increasing flow of customer communication, Insight7 steps in to fill that gap. By integrating advanced AI capabilities, it transforms conversation data into valuable insights, driving businesses to adapt and innovate in response to customer feedback. This holistic approach ensures that companies can quickly act on insights, leading to better customer engagement and satisfaction.
- Google Speech-to-Text: Widely used service offering high accuracy and adaptability.
AI-Enhanced Quality Monitoring can be significantly improved by incorporating widely used speech recognition services. Among these, one service stands out for its high accuracy and adaptability. This technology not only excels in converting spoken language into text but also enhances the quality monitoring process by providing real-time feedback. As organizations strive to transition from order-takers to consultative sales approaches, using advanced voice transcription tools allows teams to refine their interactions meaningfully.
One impressive aspect of this service is its ability to understand different accents and dialects. This adaptability facilitates accurate communication across diverse customer bases, ensuring that businesses can engage effectively with all clients. Moreover, transcription accuracy allows for detailed analysis of customer interactions. By leveraging insights derived from AI-enhanced analysis, organizations can identify areas of improvement and implement necessary training, improving overall service quality and customer satisfaction. This symbiotic relationship between advanced speech recognition and quality monitoring presents a powerful opportunity for organizations aiming to innovate their customer engagement strategies.
- IBM Watson Speech to Text: Known for its analytics and support for various languages.
The analytics capabilities of AI speech recognition tools play a crucial role in enhancing quality monitoring across various sectors. One notable aspect is the ability to support multiple languages, which broadens the accessibility of insights derived from conversations. This feature proves invaluable, particularly for businesses operating in diverse markets, as it allows for accurate monitoring of customer interactions in different languages.
Moreover, the analytical power embedded within such systems enables organizations to quickly process and analyze vast amounts of audio data. By converting speech into text, companies can efficiently extract relevant insights and identify trends or pain points that may have gone unnoticed. The combination of language support and advanced analytical capabilities facilitates a deeper understanding of customer sentiments, ultimately driving improvements in service quality and customer satisfaction. This approach transforms traditional quality monitoring into a more dynamic and effective process, making it essential for organizations aiming to stay competitive in todayโs global market.
- Amazon Transcribe: Offers real-time speech-to-text capabilities with deep learning.
With advancements in technology, real-time speech-to-text capabilities have become increasingly vital for enhancing AI-Enhanced Quality Monitoring. This innovation allows organizations to convert audio recordings into written transcripts instantly, streamlining the process of analyzing conversations. By leveraging deep learning, the technology continually improves its accuracy, ensuring that transcriptions reflect the nuances of human speech.
The ability to transcribe conversations on a large scale not only aids in quality monitoring but also supports data-driven decision-making. Organizations can quickly identify trends, extract key insights, and respond to customer needs effectively. This efficient transcription process empowers teams to visualize and analyze conversations, providing a solid foundation for improving service quality and customer satisfaction. By integrating this cutting-edge technology into their monitoring processes, businesses can not only enhance their operational efficiency but also foster a more insightful approach to understanding their customers' voices.
- Microsoft Azure Cognitive Services: Provides a suite of speech recognition APIs.
Microsoft Azure Cognitive Services offers a comprehensive suite of speech recognition APIs designed to enhance various applications, including AI-enhanced quality monitoring. These APIs are tailored to convert spoken language into text with remarkable accuracy, playing a vital role in analyzing conversations in real time. By leveraging this technology, businesses can automate the process of monitoring agent-client interactions, ensuring that quality assurance is both efficient and effective.
Integrating these advanced speech recognition capabilities allows organizations to maintain high standards of compliance and performance. The engine is trained to distinguish between different speakers, making it easier to identify individual agent contributions. Additionally, by generating detailed performance reports, companies can pinpoint areas for improvement, fostering a culture of continuous learning and adaptation among their teams. In this way, adopting cutting-edge speech recognition APIs is a key step towards achieving superior quality monitoring and customer service excellence.
Conclusion: The Future of AI-Enhanced Quality Monitoring and Speech Recognition
The future of AI-Enhanced Quality Monitoring suggests transformative advancements in how organizations assess and improve performance. By integrating sophisticated speech recognition technologies, businesses can achieve enhanced accuracy and efficiency in monitoring interactions. This shift not only streamlines quality assessment but also provides actionable insights, allowing teams to tailor training and support according to individual performance metrics.
Moreover, as AI continues to evolve, we can anticipate even more sophisticated algorithms that will adapt to diverse communication styles. This adaptability will lead to more personalized feedback and better compliance management, fundamentally reshaping the quality monitoring landscape. Embracing these innovations will empower organizations to foster better customer experiences and drive overall productivity.