Predicting customer experience (CX) score decline is crucial for any business aiming to maintain satisfaction and loyalty. Understanding the trends in CX score forecasting enables organizations to proactively address issues before they escalate. By anticipating declines, teams can focus on targeted improvements, ensuring that they remain aligned with customer expectations and preferences.
CX score forecasting empowers companies to enhance their strategies effectively. By analyzing historical data and customer feedback, organizations can identify potential pitfalls and develop tailored action plans. This proactive approach not only prevents loss of customers but also fosters a culture of continuous improvement, ultimately leading to a more resilient and responsive business environment.
Analyze & Evaluate Calls. At Scale.
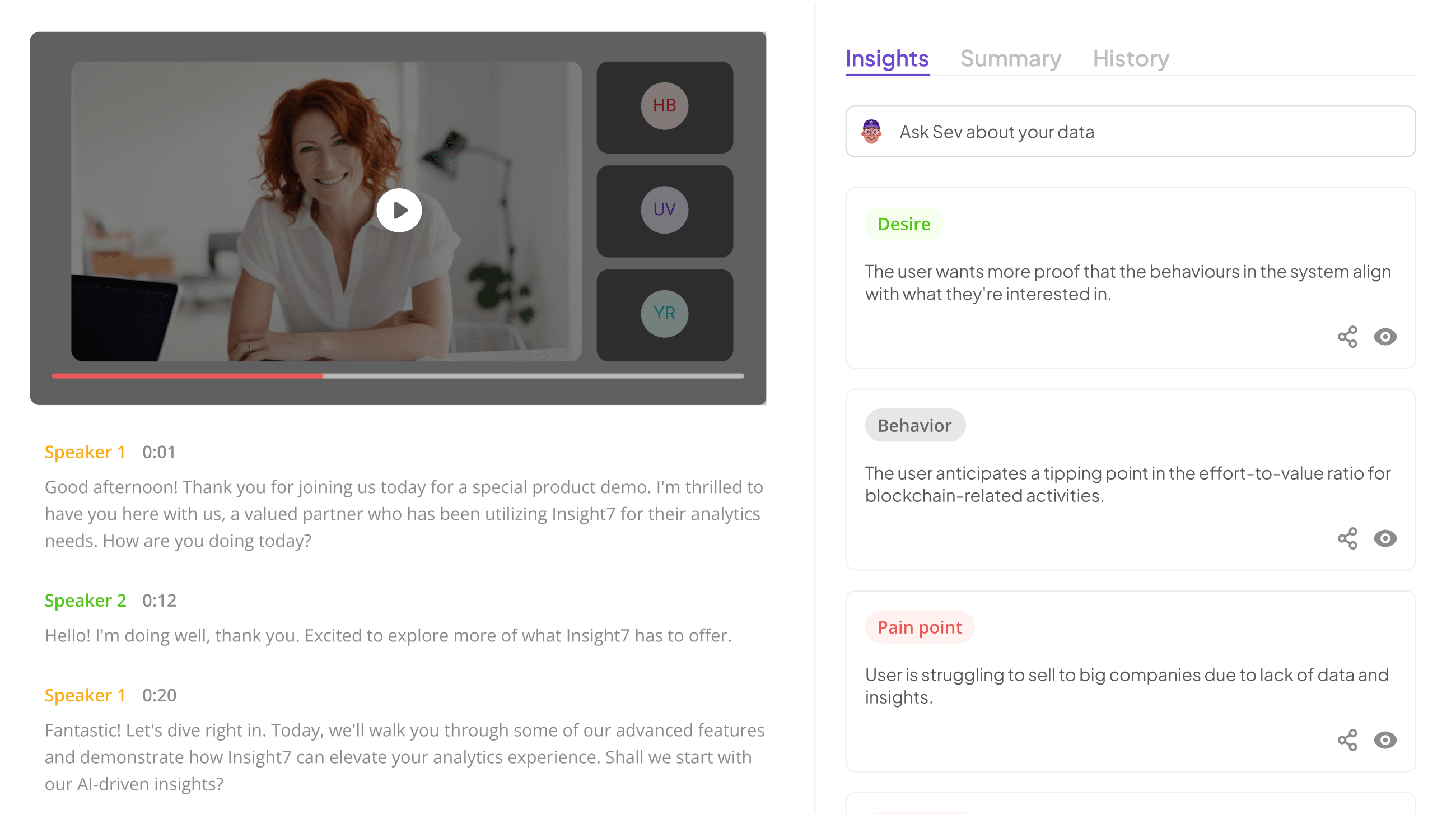
Understanding Predictive Modeling in CX Score Forecasting
Predictive modeling plays a pivotal role in CX Score Forecasting, allowing businesses to anticipate declines before they occur. By analyzing historical data, organizations can identify patterns that signal potential drops in customer experience scores. Understanding these patterns forms the core of effective CX Score Forecasting, as it bridges the gap between data analytics and strategic decision-making.
To implement predictive modeling successfully, itโs essential to grasp several key components. First, data accuracy is crucial; ensuring that the information used is clean and relevant sets a solid foundation. Then, selecting appropriate modeling techniques tailored to business needs enhances predictive capabilities. Lastly, continuous monitoring and adjustment of models improve their effectiveness over time. By following these principles, organizations can harness the full potential of CX Score Forecasting, allowing them to proactively address customer concerns and boost overall satisfaction.
The Basics of Predictive Modeling
Predictive modeling serves as a powerful technique that allows businesses to foresee potential trends, particularly in customer experience (CX) scores. It involves analyzing historical data to make informed predictions about future outcomes, helping organizations preemptively address declines. Using statistical algorithms and machine learning, these models extract patterns and insights from complex datasets, enabling companies to understand what influences customer satisfaction.
In the context of CX Score Forecasting, understanding key factors such as customer feedback, purchase behavior, and service interactions is crucial. Models allow businesses to identify risk factors for score decline, thereby facilitating proactive measures. By integrating predictive modeling techniques, companies can significantly enhance their ability to manage customer experiences, ensuring they not only react to issues as they arise but also anticipate them before they negatively impact satisfaction levels. This not only fosters a more responsive approach but also drives strategic decision-making across various touchpoints.
How Predictive Modeling Applies to CX Score Forecasting
Predictive modeling plays a crucial role in CX score forecasting by enabling organizations to identify potential declines before they occur. By analyzing historical data, businesses can uncover patterns that indicate changes in customer sentiment or experience. This process not only helps in recognizing declining scores but also allows for timely interventions to enhance the overall customer experience.
One of the primary advantages of predictive modeling is its ability to utilize various data points, such as customer feedback, service interactions, and performance metrics. These insights can inform strategies to improve service quality and customer satisfaction. Additionally, businesses can tailor their approaches based on the predictions, ensuring resources are allocated effectively. Ultimately, this proactive stance in CX score forecasting translates into improved customer loyalty and retention, fostering long-term relationships with clients.
Extract insights from interviews, calls, surveys and reviews for insights in minutes
Steps to Implementing CX Score Forecasting
To effectively implement CX Score Forecasting, organizations should begin with meticulous data collection and preparation. This initial step involves gathering relevant data from diverse sources, including customer interactions and feedback mechanisms. Cleaning and structuring this data ensures accuracy and readiness for analysis. The integrity of data is paramount, as it underpins all subsequent forecasting efforts.
Once data is prepared, the next phase is choosing the right predictive analytics tools. Opt for platforms such as Insight7, IBM Watson Analytics, or Tableau to facilitate robust analysis. These tools can streamline the process of model building and testing, enabling accurate predictions.
Building and testing predictive models is the final step. Collaborate with data analysts to develop models that can recognize patterns in CX scores. Testing these models will validate their effectiveness and refine forecasting accuracy. Following these steps will lead your organization towards a more proactive approach in managing customer experiences and anticipating potential score declines.
Step 1: Data Collection and Preparation
Effective CX Score forecasting begins with a thorough approach to data collection and preparation. Initially, it is vital to gather relevant customer interaction data from various sources like surveys, transcripts, and behavioral analytics. This comprehensive dataset will serve as the foundation for formulating predictive models. Ensuring the accuracy and completeness of the data is essential to avoid skewed results.
After gathering the data, preparation involves cleaning and structuring it for analysis. This includes eliminating duplicates, filling in missing values, and categorizing information for better insights. Properly prepared data allows organizations to identify trends and patterns essential for behavioral predictions. In this step, itโs important to integrate both quantitative metrics and qualitative feedback, providing a holistic view of customer experiences. By adhering to these principles, businesses can facilitate robust CX Score forecasting, ultimately leading to improved customer satisfaction and retention.
Step 2: Choosing the Right Predictive Analytics Tools
Selecting the right predictive analytics tools is a pivotal step in successfully anticipating a decline in CX score. To effectively forecast these scores, you must choose tools that specialize in data analysis, visualization, and model building. The tools selected should not only cater to your organization's data needs but also support intuitive usage for better team adoption.
When evaluating predictive analytics tools, consider factors like data integration capabilities, ease of use, and analytical depth. Tools like IBM Watson Analytics offer robust features for extracting insights from complex datasets, while platforms like Tableau and Microsoft Power BI excel in visualizing those insights for your team. Ultimately, the aim is to select a tool that aligns with both your immediate and long-term CX score forecasting objectives, enabling you to monitor customer feedback and adjust strategies in real-time. A well-chosen analytics tool can empower your team to foresee trends and respond proactively to ensure a positive customer experience.
- Insight7
In the realm of CX Score Forecasting, understanding the patterns behind customer experiences is crucial. Predictive modeling serves as a powerful tool, allowing businesses to anticipate potential declines in customer satisfaction. By analyzing historical data, organizations can identify warning signs and address issues proactively, thereby enhancing customer loyalty and trust.
Implementing effective predictive modeling involves several key steps. First, compile relevant data from various sources, ensuring a comprehensive understanding of customer interactions. Next, select appropriate analytics tools that can handle the complexity and scale of your data. Finally, build and rigorously test predictive models to ensure accuracy in forecasting CX scores. By following these structured steps, organizations can transform data into actionable insights, ultimately leading to improved customer experiences.
- IBM Watson Analytics
IBM Watson Analytics offers an intuitive platform for users aiming to enhance their CX Score forecasting efforts. By providing powerful data visualization and analysis tools, it empowers businesses to quickly uncover trends and insights that could signal a potential decline in customer experience metrics. Users can conduct detailed queries across varied datasets, enabling them to assess patterns and draw meaningful connections from customer feedback.
One of the standout features of this platform is its ability to analyze qualitative and quantitative data simultaneously. Thus, it helps organizations gauge customer sentimentsโboth positive and negativeโapproaching predictive modeling from multiple angles. This comprehensive approach ensures that businesses stay ahead of potential downturns in their CX scores, allowing them to take proactive measures. Ultimately, IBM Watson Analytics transforms raw data into actionable insights, making it an invaluable resource for CX Score forecasting and improving overall customer satisfaction.
- SAS Customer Intelligence 360
SAS Customer Intelligence 360 is a powerful tool designed to enhance customer experience by providing advanced analytical insights. In the context of CX Score Forecasting, it allows organizations to understand customer behaviors more deeply and anticipate potential declines in their CX scores. By integrating various data sources, this platform supports businesses in extracting valuable insights that can inform strategic decisions.
With real-time analytics, companies can monitor changing customer sentiments and identify trends affecting their satisfaction levels. This empowers them to address issues proactively before they escalate. In essence, SAS Customer Intelligence 360 serves as a cornerstone for effective CX Score Forecasting, equipping businesses with the knowledge needed to create superior customer experiences and drive lasting loyalty. By leveraging predictive modeling, organizations can continually refine their approach to customer engagement and maintain a competitive edge in the market.
- Tableau
Tableau serves as a powerful tool in the realm of CX Score Forecasting, enabling organizations to visualize and analyze data effectively. With its user-friendly interface, Tableau allows users to create interactive dashboards that present key metrics at a glance. This facilitates a deeper understanding of customer experience trends over time, helping stakeholders identify potential score declines proactively.
A pivotal aspect of utilizing Tableau involves capturing relevant data and transforming it into actionable insights. By connecting various data sources, Tableau compiles vital information regarding customer interactions and feedback. This enables organizations to pinpoint patterns and anomalies in customer satisfaction levels. Ultimately, employing Tableau not only enhances visualization capabilities but also fosters informed decision-making, ensuring that strategies are aligned with improving the overall customer experience and mitigating risks associated with decline.
- Microsoft Power BI
Microsoft Power BI plays a pivotal role in CX Score Forecasting by transforming data into insightful visualizations. This powerful tool allows organizations to monitor customer feedback trends and identify potential declines in customer experience scores. Users can easily analyze various data points, including comments and satisfaction ratings, to grasp the factors influencing customer perceptions. By employing intuitive dashboards, businesses can pinpoint areas needing attention, driving proactive responses to improve overall customer experience.
Additionally, Power BI facilitates comparative analysis across different datasets, such as contrasting feedback from various locations. This capability enables organizations to discover patterns or discrepancies in customer sentiment, enhancing their understanding of regional customer behaviors. Furthermore, the integration of advanced analytics allows stakeholders to explore predictions based on historical data, on preventing declines in CX. Thus, leveraging Microsoft Power BI effectively supports informed decision-making for strategic customer experience improvements.
Step 3: Building and Testing Predictive Models
In this crucial step of building and testing predictive models, the aim is to create a robust framework for CX Score forecasting. Begin by selecting the appropriate algorithm suited to your dataset and desired outcomes. Common models include regression analysis, decision trees, and neural networks. These algorithms can provide insights into which factors most significantly influence customer experience scores.
Once your models are built, rigorous testing is essential. Validate your models by using historical data to gauge their predictive accuracy. Split your data into training and test sets to evaluate how well your model performs in real-world scenarios. Adjust parameters as needed to optimize your model's performance, ensuring it effectively identifies potential declines in customer experience scores. Each iteration helps refine your predictions, enabling businesses to proactively address issues that could lead to a drop in customer satisfaction.
Conclusion: Enhancing Business Strategies with CX Score Forecasting
Integrating CX Score Forecasting into your business strategy can significantly elevate customer experience management. This approach allows businesses to pinpoint potential declines in customer satisfaction, giving teams ample time to implement corrective measures. By prioritizing data-driven insights, organizations can foster a proactive culture that values customer feedback and adapt training accordingly.
Moreover, utilizing predictive modeling enhances decision-making processes by turning raw data into actionable strategies. By regularly analyzing customer interactions, businesses can refine services and address common issues swiftly. In essence, CX Score Forecasting empowers companies to not only understand their current standing but also to anticipate future trends, ensuring lasting success in an ever-evolving market.