Virtual Experimentation Models represent a transformative approach in simulated research, offering a new dimension in experimentation. These models enable researchers to replicate real-world processes in a controlled environment, leading to enhanced understanding and innovative solutions. Through the use of advanced simulations and data integration, Virtual Experimentation Models allow for the exploration of hypotheses and testing of scenarios that might be impractical or unsafe in real life.
Incorporating Digital Twins into these models further amplifies their effectiveness. By creating a dynamic, digital representation of physical entities, researchers can analyze data in real time and make informed decisions. Ultimately, this synergy between Virtual Experimentation Models and Digital Twins not only accelerates research but also enhances the reliability of findings, paving the way for groundbreaking applications across various domains.
Analyze qualitative data. At Scale.
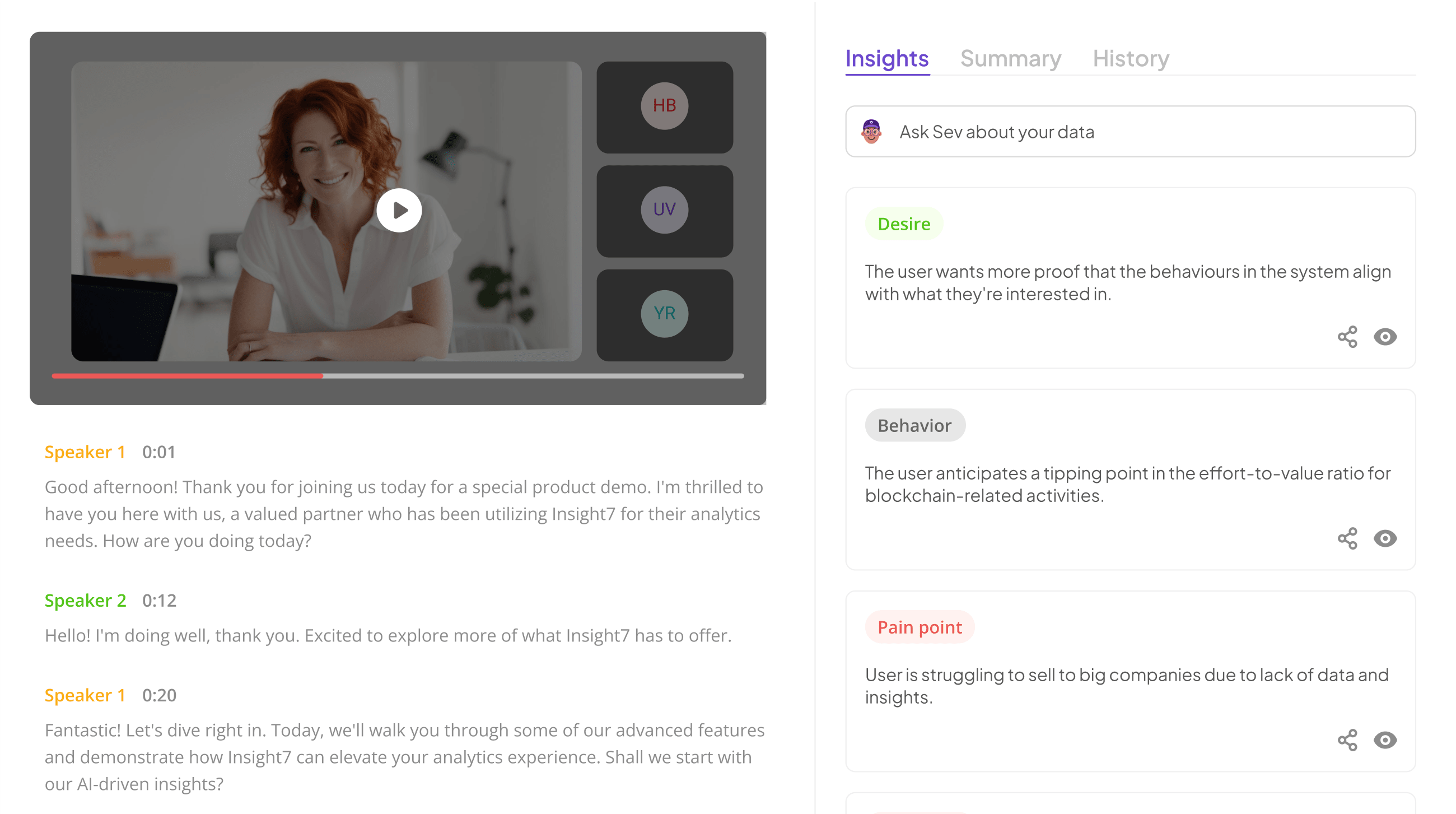
Understanding Virtual Experimentation Models and Digital Twins
Virtual Experimentation Models offer researchers an innovative way to simulate real-world scenarios. By creating digital representations that mirror physical entities, these models facilitate rigorous testing and analysis. Digital Twins play a pivotal role, as they connect real-time data with these virtual environments. This synergy enhances understanding and forecasting by providing significant insights into behavior and performance.
Exploring Virtual Experimentation Models requires grasping basic concepts, including their ability to mimic real systems under various conditions. Researchers can assess potential outcomes, test hypotheses, and visualize results without the risks associated with physical experimentation. This approach not only saves time and resources but also ensures accuracy in data interpretation. Ultimately, such models serve as invaluable tools for advancing knowledge in both academia and industry, bridging the gap between theoretical research and practical application.
Definition and Core Concepts
Virtual experimentation models represent a transformative shift in research methodologies. At the core of these models is the digital twin concept, a virtual representation that mirrors physical objects or systems. By creating a comprehensive digital replica, researchers can simulate experiments in a controlled environment, thus reducing risks and costs associated with physical experimentation.
These models enable a deeper understanding of complex systems by allowing for manipulation and testing of variables without real-world consequences. They provide insights into potential outcomes, which can then be applied to real-world applications. As research continues to advance, virtual experimentation models will play a crucial role, particularly in fields such as healthcare, engineering, and environmental science. This technology empowers researchers to innovate while minimizing resource expenditure and improving efficiency.
Benefits of Using Virtual Experimentation Models
Virtual Experimentation Models offer significant advantages in research settings, particularly in the realm of digital twins. One primary benefit is the ability to conduct experiments in a simulated environment without the constraints of physical limitations. Researchers can explore various scenarios, tweak parameters, and observe outcomes, all while minimizing costs and risks associated with traditional experimentation.
Another critical advantage lies in efficient data analysis. Virtual Experimentation Models harness real-time data inputs, enabling researchers to gather insights quickly and effectively. This rapid feedback loop facilitates informed decision-making, leading to improved product development and research outcomes. Furthermore, such models foster collaboration among teams, allowing for the sharing of insights across different domains and enhancing innovative approaches to problem-solving. As research continues to evolve, these benefits underscore the integral role of Virtual Experimentation Models in shaping the future of simulated research studies.
Extract insights from interviews, calls, surveys and reviews for insights in minutes
Steps to Implementing Digital Twins in Virtual Experimentation Models
Implementing Digital Twins in Virtual Experimentation Models requires a systematic approach. First, identifying the right research area is crucial. This step involves pinpointing specific challenges or processes that would benefit from a digital twin. By understanding the key components and objectives, researchers can streamline the implementation process effectively.
Next, creating accurate digital representations is essential. This involves developing detailed models that reflect the physical entities or processes under study. It’s important to capture essential parameters to enhance predictive accuracy. Finally, integrating real-time data into these models brings them to life. Real-time data allows for dynamic adjustments and simulations, providing deeper insights during experimentation. This holistic approach ensures that digital twins effectively enhance virtual experimentation models, facilitating more sophisticated research studies and analyses.
Step 1: Identifying the Right Research Area
Identifying the right research area is essential for effective application of virtual experimentation models. Begin by aligning your study goals with specific industrial challenges or theoretical frameworks. This ensures relevance and enhances the value of your findings. Consider the areas where digital twins can replicate complex processes or systems, as these models allow researchers to simulate scenarios that can lead to significant insights and innovations.
Next, focus on the technologies available in the chosen field. Analyzing current trends and advancements can illuminate how digital twin technology might transform research capabilities. This approach invites collaboration across interdisciplinary teams, allowing for diversified perspectives and richer results. Ultimately, selecting an appropriate research area not only facilitates practical experimentation but also opens doors to potential applications in various sectors. By being strategic in your identification process, you set a solid foundation for impactful research using virtual experimentation models.
Step 2: Creating Accurate Digital Representations
Creating accurate digital representations is a critical step in developing effective virtual experimentation models. To begin, it’s essential to collect comprehensive data on the physical object or process being simulated. This data forms the foundation of the digital twin, ensuring that the model closely mirrors real-world conditions. Precision in this initial stage greatly influences the reliability of subsequent experiments.
Next, the representation must capture both static configurations and dynamic behaviors. Employing advanced algorithms and simulation software is vital for translating complex interactions into actionable insights. Continuously validating the digital twin against empirical data enhances its accuracy and efficiency in predictive simulations. By iterating on these models through real-time adjustments, researchers can maintain the relevance and applicability of their virtual experimentation frameworks.
Overall, the success of virtual experimentation models hinges on creating precise digital representations, enabling researchers to explore possibilities and drive innovative solutions.
Step 3: Integrating Real-Time Data
Integrating real-time data into virtual experimentation models is crucial for enhancing the accuracy and relevance of simulated research studies. This step involves seamlessly connecting live data streams with digital twins, allowing for immediate analysis and decision-making. By incorporating real-time data, researchers can adjust their models dynamically, reflecting changes in variables and conditions. This ensures that models remain relevant to ongoing real-world developments, providing insights that are both timely and actionable.
To effectively integrate real-time data, consider the following key elements: 1) Data Sources, which identify the various channels from which data can be collected; 2) Data Processing Methods, essential for converting raw data into a usable format; and 3) Real-time Analysis, which focuses on employing techniques to derive insights instantly. By prioritizing these elements, researchers can significantly improve the predictive power of their virtual experimentation models, leading to more reliable outcomes and smarter decision-making in simulated environments.
Essential Tools for Building Digital Twins
Building digital twins requires a variety of essential tools that automate and enhance the modeling process. These tools provide the infrastructure necessary to create accurate and dynamic virtual representations of physical assets. Critical to this endeavor are advanced software platforms that facilitate the integration of real-time data and simulations, ultimately enabling effective virtual experimentation models.
Among the top options for constructing digital twins are Siemens Digital Industries Software and IBM Digital Twin Exchange. Siemens offers a comprehensive suite that supports the entire lifecycle of digital twins. On the other hand, IBM’s platform emphasizes connectivity and data management, making it easier to analyze interactions within complex systems. Meanwhile, tools like ANSYS Twin Builder and Azure Digital Twins provide specialized capabilities for simulation and deployment, allowing researchers to visualize and predict outcomes precisely. Selecting the right set of tools empowers teams to derive meaningful insights from their digital models and deepen their understanding of the systems they study.
insight7
Virtual experimentation models have emerged as a vital component in the realm of simulated research studies. These models offer researchers the ability to create highly accurate digital representations of physical systems, enabling them to conduct experiments in a virtual environment. This approach not only preserves resources but also accelerates the pace of innovation by facilitating rapid testing of hypotheses without the logistical challenges associated with physical experiments.
The efficacy of virtual experimentation models lies in their capacity to integrate real-time data and adaptive algorithms. By continuously updating the digital twin, researchers can simulate various scenarios and predict outcomes with increased precision. As we move forward, adopting these models will become essential for organizations seeking to enhance their research capabilities. Ultimately, virtual experimentation models represent a paradigm shift in how we approach research, promising to unlock new avenues for exploration in diverse fields.
Siemens Digital Industries Software
Siemens Digital Industries Software provides innovative tools that facilitate the creation and management of Virtual Experimentation Models. These models form the foundation for developing digital twins, allowing researchers to simulate, analyze, and optimize processes in a virtual environment. By utilizing these powerful software solutions, organizations can enhance their research capabilities and accelerate experimentation without the constraints of physical constraints.
The integration of digital twins into research enables an unprecedented level of insight and precision. Researchers can gather real-time data and apply it to their virtual models, ensuring that simulations closely reflect real-world scenarios. Consequently, the collaboration between virtual experimentation and digital twin technology empowers organizations to make informed decisions quickly, ultimately leading to improved research outcomes and innovation. By adopting these tools, academic and industrial researchers can drive significant advancements in their respective fields.
IBM Digital Twin Exchange
The IBM Digital Twin Exchange offers a unique environment for researchers to explore and implement virtual experimentation models. This platform allows users to create accurate digital twins that simulate real-world scenarios, aiding in various research applications. By transforming physical entities into their digital counterparts, researchers can conduct experiments without the limitations and risks associated with traditional methods.
Collaborating within this exchange enhances the research process by providing access to a broad range of simulation tools and data sets. Users can analyze and manipulate digital representations to develop insights that inform decision-making in diverse fields. The ability to rapidly prototype and iterate on virtual experimentation models promotes innovation while reducing costs and time. Ultimately, harnessing the capabilities of a digital twin exchange empowers researchers to advance their studies effectively, fostering progress across multiple disciplines.
ANSYS Twin Builder
In the realm of simulated research, ANSYS Twin Builder plays a pivotal role in constructing Virtual Experimentation Models that mirror real-world scenarios. This sophisticated tool enables researchers to create accurate digital representations of physical systems, thus facilitating extensive testing and validation. By integrating physics-based modeling with real-time data, users can derive meaningful insights and make informed decisions.
When utilizing ANSYS Twin Builder, users benefit from its robust features. First, it enables the seamless integration of various data sources, enriching the model with real-world measurements and performance indicators. Second, it offers powerful simulation capabilities to evaluate different scenarios, which helps in identifying optimal operational strategies. Finally, its user-friendly interface allows researchers to collaborate and iterate quickly, enhancing the overall efficiency of the experimentation process. These features collectively drive innovation and contribute to more effective virtual experimentation in research studies.
Azure Digital Twins
Azure Digital Twins provides a robust framework for creating digital replicas of physical environments. This technology enables researchers to simulate various scenarios, enhancing their understanding of complex systems. By employing virtual experimentation models, teams can analyze data patterns and relationships that may otherwise be difficult to observe. With Azure Digital Twins, the integration of real-time sensor data facilitates the creation of dynamic models that evolve with changing conditions.
Implementing Azure Digital Twins enhances the accuracy of predictions related to research studies. Users can construct highly detailed simulations that mirror real-life situations, allowing for thorough testing and validation of hypotheses. Additionally, collaborative features empower teams to share insights and improve outcomes collectively. Ultimately, Azure Digital Twins plays a crucial role in advancing simulated research studies, offering a comprehensive approach to understanding and optimizing real-world applications.
Conclusion: The Future of Virtual Experimentation Models in Research
The future of Virtual Experimentation Models in research promises to revolutionize how scientists conduct studies and analyze data. Emerging technology, particularly through the integration of digital twins, enhances our ability to create precise simulations. These models will likely lead to faster discoveries while minimizing resource expenditure, allowing researchers to focus on innovation.
Moreover, as Virtual Experimentation Models evolve, they will facilitate collaboration across disciplines. This synergy will open new avenues for experimentation, making research more adaptive and responsive to real-world challenges. Ultimately, the ongoing development of these models stands to enhance research reliability and depth, ushering in a new era of scientific exploration.