Bias-Free Analytics is essential for fostering ethical AI practices in data analysis. In an increasingly data-driven world, it has become crucial to ensure that algorithms operate without prejudice. Recognizing the impact of bias on decision-making processes is the first step toward creating a more equitable landscape. By employing bias-free analytics, organizations can cultivate trustworthy insights that genuinely reflect diverse perspectives.
Implementing strategies to achieve bias-free analytics involves diligent data collection and model design. This not only identifies potential sources of bias but also ensures that data accurately represents the population it serves. Organizations committed to ethical AI must prioritize these practices, as they contribute to fairer outcomes and build trust in AI systems.
Analyze qualitative data. At Scale.
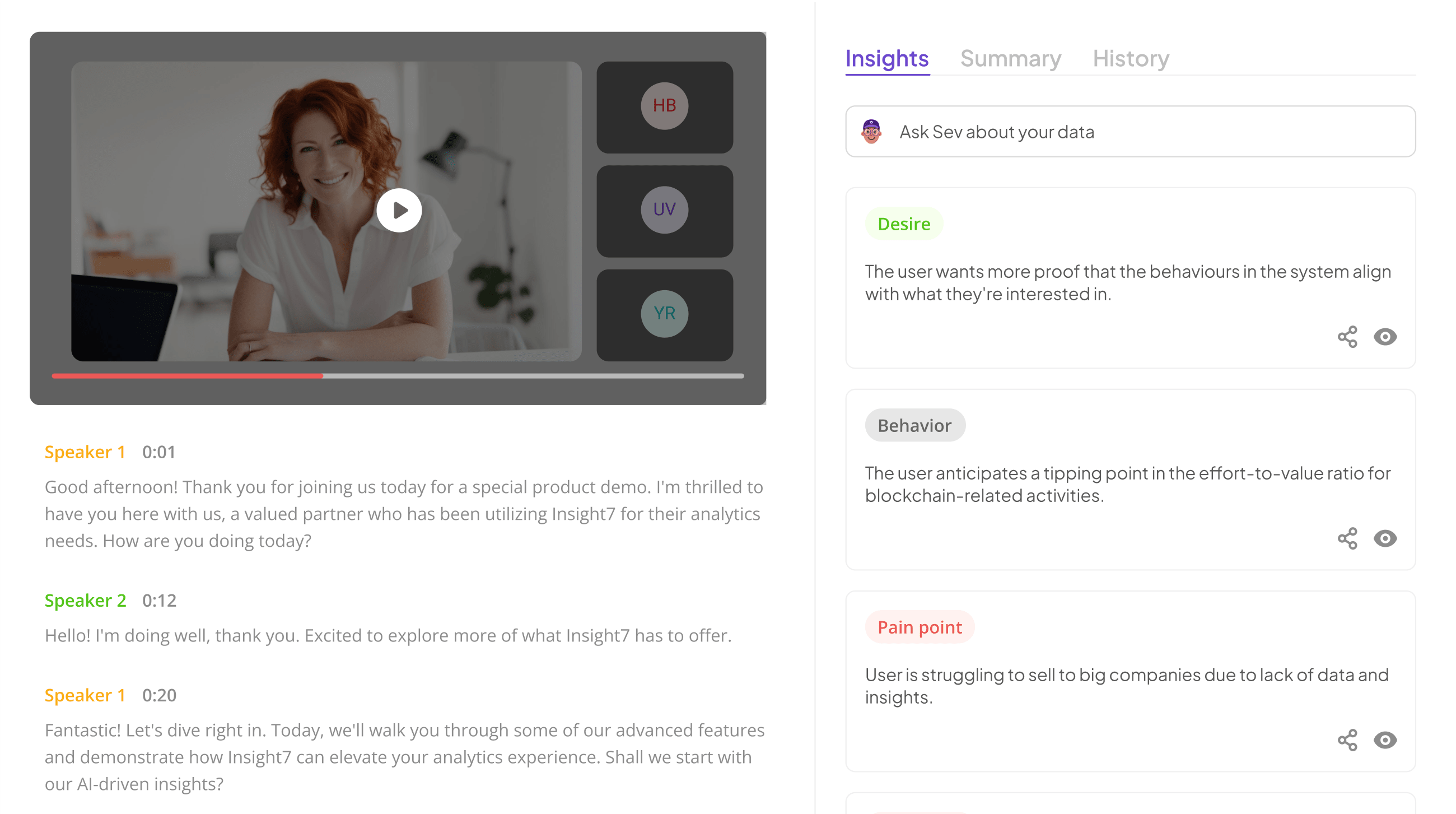
Understanding Algorithmic Bias in Data Analysis
Algorithmic bias can significantly skew data analysis outcomes, often leading to unintended, unjust consequences. Understanding this bias requires recognizing its various sources, which can include flawed data collection methods or societal stereotypes ingrained into algorithms. These biases can arise from historical data that reflect prejudices, skewing predictions and insights. By comprehensively analyzing data sources and their contexts, stakeholders can begin to identify where biases may be embedded.
The impact of algorithmic bias extends beyond inaccuracies, affecting decision-making processes across industries. When bias infiltrates data analytics, the quality of insights derived can diminish, leading to inequitable solutions. This is why striving for bias-free analytics is essential for fostering fairness in AI systems. A commitment to eliminating biases in data collection and algorithm design will significantly enhance the integrity of analytical outcomes. This commitment encourages the creation of ethical AI frameworks that prioritize equitable treatment and transparency, ultimately promoting trust within the analytical process.
Recognizing Sources of Bias
Bias in data analysis can stem from various sources, impacting the integrity of conclusions drawn from that data. Awareness of these sources is essential for achieving bias-free analytics. First, biases can emerge from the data collection process, such as when the demographics of surveyed individuals are not representative of the broader population. This lack of representation skews results and undermines decision-making.
Second, algorithmic design can introduce bias. If algorithms are trained on unbalanced data sets, they may perpetuate existing prejudices or even amplify them. For example, if a predictive model is primarily based on historical data reflecting biased outcomes, it may lead to unfair predictions. Finally, user interpretation also plays a role; analysts may unconsciously allow their beliefs to influence the outcomes. Recognizing these sources of bias is critical to implementing solutions that ensure bias-free analytics in AI systems.
Impacts of Algorithmic Bias on Outcomes
Algorithmic bias in data analysis can significantly distort outcomes, impacting decision-making across various domains. When biases are embedded within analytical processes, they can lead to skewed interpretations and flawed conclusions. For instance, biased data may unfairly disadvantage certain groups while providing advantages to others, perpetuating inequality.
Recognizing these impacts is crucial for fostering bias-free analytics. The repercussions of algorithmic bias extend beyond inaccuracies; they can manifest as a loss of trust in AI systems and hinder progress toward equitable solutions. Acknowledging these factors, organizations must commit to ethical standards in data analysis. By ensuring that analytics processes are transparent, inclusive, and accountable, they can significantly enhance the integrity of their outcomes. Understanding how bias affects results is a vital first step toward developing more reliable AI tools that can serve diverse populations. This dedication to ethical practices will enable the creation of a more just and effective analytic environment.
Steps Toward Bias-Free Analytics in AI Systems
To achieve bias-free analytics in AI systems, organizations must implement strategic steps to ensure fairness and transparency in data processes. The starting point involves identifying potential biases in data sources, which requires a thorough evaluation of how data is collected and the context in which it exists. Understanding a dataset's nuances can reveal systemic inequalities and lead to more thoughtful approaches.
Next, ensuring representative data sampling is crucial. This means incorporating diverse perspectives and demographics to reflect the entire population accurately. Without this representation, analytics can inadvertently reinforce existing biases. Additionally, incorporating fairness directly into the model training process is essential, alongside rigorous evaluations and tests aimed at uncovering any hidden biases. This proactive approach establishes a foundation for bias-free analytics, ensuring that AI systems contribute positively to decision-making and analysis.
Through careful attention to these steps, organizations can move closer to achieving bias-free analytics in their AI systems, fostering trust and integrity in their data practices.
Extract insights from interviews, calls, surveys and reviews for insights in minutes
Implementing Ethical Guidelines for Data Collection
Implementing ethical guidelines for data collection is crucial in fostering a framework for Bias-Free Analytics. First, it is essential to identify potential biases in data sources. This includes understanding where the data originates and the demographics it represents. By scrutinizing these sources, analysts can pinpoint areas where bias may creep in, which is a vital step toward ensuring fair outcomes.
Next, ensuring representative data sampling is equally important. This involves consciously selecting a diverse range of samples that accurately reflect the population of interest. A well-rounded dataset minimizes the risk of inadvertently favoring one group over another. Notably, both identifying biases and ensuring representative samples will contribute significantly to the development of systems that perform ethically and effectively.
By taking these steps, organizations can create a data-driven environment that prioritizes fairness and accountability, leading to robust AI systems that uphold ethical standards.
- Identifying Potential Biases in Data Sources
Identifying potential biases in data sources is crucial in the journey toward Bias-Free Analytics. Bias can stem from various origins, such as historical data, social stereotypes, or selection processes that disproportionately represent certain groups. By critically examining the data's sourcing and collection methods, we can uncover underlying issues that contribute to biases. This proactive identification allows us to address potential inequities before they influence outcomes.
Moreover, understanding the context in which data is gathered is essential. Data collected in a specific environment may reflect localized biases not applicable in broader scenarios. For instance, geographical or cultural factors can shape the information captured. Conducting a thorough audit of data sources helps ensure that we utilize accurate, representative data sets. This step promotes fairness and transparency in AI solutions, fostering a commitment to ethical standards in data analysis across all industries.
- Ensuring Representative Data Sampling
Ensuring representative data sampling is critical in creating effective and fair AI systems. The quality of the data we collect directly impacts the insights derived from it. First, itโs essential to define the target population accurately, ensuring all relevant groups are represented. In doing so, we minimize the risk of perpetuating existing biases that may skew analysis results.
Second, employing stratified sampling techniques can enhance representation from various demographics. This approach allows for a more balanced sample that reflects the diversity found in a broader population. By incorporating these methods, organizations can work toward achieving bias-free analytics. This ultimately leads to improved decision-making, fostering trust and confidence in AI technologies. A commitment to representative sampling not only enriches data quality but also supports ethical standards necessary for responsible AI deployment.
Algorithm Design for Bias-Free Analytics
Designing algorithms that achieve bias-free analytics is crucial in ensuring ethical AI practices. The first step involves incorporating fairness into model training, which means using diverse training datasets that reflect varied populations. This approach helps to reduce the risk of perpetuating existing inequalities. Moreover, actively seeking out and mitigating potential biases during the model development process can lead to more accurate and equitable outcomes.
The next step is to systematically evaluate and test algorithms for possible biases. Regularly assessing model predictions against fairness metrics ensures that any disparities are identified and addressed early on. By creating transparent evaluation criteria, organizations can facilitate accountability and encourage continuous improvements. Overall, focusing on these critical aspects will contribute significantly to achieving bias-free analytics in data analysis, allowing AI systems to provide more reliable and equitable insights across all sectors.
- Incorporating Fairness in Model Training
Incorporating fairness in model training is crucial for achieving Bias-Free Analytics within AI systems. First, it is essential to understand that models learn from data, which may contain inherent biases. Therefore, it is important to identify these biases during the training phase. This involves scrutinizing the datasets, examining the demographics represented, and recognizing potential stereotypes embedded in the data. Analysts should ensure that training data reflects a diverse set of scenarios to promote equitable outcomes for all users.
Once potential biases are identified, the next step involves implementing fairness-enhancing interventions in model training. Techniques such as re-weighting training samples or introducing fairness constraints can be highly effective. Testing and validating these models for bias is essential after training. Continuous monitoring of the model's predictions will help uncover any lingering disparities and allow data scientists to adjust the model accordingly. These strategies contribute significantly to the creation of fairer AI systems and support the overarching goal of Bias-Free Analytics.
- Evaluating and Testing for Bias in Models
Evaluating and testing for bias in models is a critical step toward achieving Bias-Free Analytics. This process begins by establishing baseline metrics that assess model performance across various demographic groups. These metrics help identify potential discrepancies that could indicate bias, ensuring that the model treats all inputs fairly and equitably.
Next, itโs important to continually validate models against test datasets that represent different populations. Techniques such as cross-validation and fairness metrics should be employed to monitor performance over time. Regular audits can highlight areas for improvement, allowing data scientists to refine algorithms and enhance fairness. By instituting these practices, organizations can promote ethical AI development, leading to better trust and transparency in data analysis outcomes. Creating an environment of accountability not only mitigates bias but also drives innovation in AI technologies.
Tools to Foster Bias-Free Analytics
To achieve bias-free analytics, utilizing effective tools is essential. Various platforms offer capabilities to detect and mitigate biases during data analysis. For instance, tools like insight7 provide advanced methods for spotting inconsistencies within data sets, ensuring that any potential biases are addressed early in the process.
Additionally, IBM Watson OpenScale enables continuous monitoring of AI models, allowing analysts to track and assess biases over time. Google Cloud AI Platform is another valuable resource, focusing on fairness and interpretability, essential components of ethical analytics. Other tools, such as Microsoft Fairlearn, help in evaluating AI systems right at the design stage, while TensorFlow Model Analysis aids in visualizing fairness metrics effectively. By integrating these tools into data practices, organizations can work towards achieving bias-free analytics, thereby fostering ethical AI in their analyses.
- insight7: Advanced tool for detecting and mitigating bias in data analysis.
Recognizing the growing complexity of data analysis, insight7 emerges as a pioneering tool designed to detect and mitigate biases in data efficiently. This advanced platform facilitates real-time analysis, enabling data analysts to scrutinize vast amounts of customer interactions seamlessly. Users can easily identify patterns and anomalies that may indicate potential biases, ensuring a commitment to Bias-Free Analytics throughout the data lifecycle.
The tool empowers organizations to proactively tackle algorithmic bias by integrating ethical considerations into data evaluation. It enhances model training by offering insights into fairness and representation, critical for reliable analytics. With insight7, analysts can better comprehend the implications of their findings, shifting from passive data interpretation to active, informed decision-making. This innovative tool not only helps companies stay ahead of their competition but also fosters a culture of accountability and awareness regarding the implications of bias in AI.
Achieving Bias-Free Analytics in data analysis is crucial for fostering trust and fairness in AI systems. The journey involves recognizing and addressing algorithmic biases that can distort analytical outcomes. To understand this importance, we must first identify the potential sources of bias, including unrepresentative data and flawed algorithms, which can lead to significant misinterpretations and inequalities in decisions.
As we navigate this complex landscape, we must actively implement ethical guidelines that prioritize the integrity of data collection processes. This includes ensuring that our data sources are diverse and representative, enabling us to build models that reflect the wide range of perspectives in the real world. Embracing fairness in model training is also essential, as it ensures that predictions do not reinforce existing biases. Ultimately, taking these steps towards Bias-Free Analytics helps to create systems that not only perform accurately but also uphold ethical standards and promote social equity.
- IBM Watson OpenScale: Offers features for tracking, measuring, and mitigating bias in AI models.
IBM Watson OpenScale provides essential capabilities for tracking, measuring, and mitigating bias in AI models, fostering a commitment to Bias-Free Analytics. By using sophisticated algorithms and detailed analytics, this platform allows organizations to gain insights into potential biases lurking within their models. As data is analyzed, users can identify disparities in outcomes based on various demographic factors, thus addressing concerns about fairness in decision-making processes.
Key features of this platform include continuous monitoring and transparency in the AI lifecycle. Stakeholders can set parameters that trigger alerts for potential bias, enabling proactive adjustments before problems escalate. Users can engage in iterative testing to validate model performance against fairness metrics, ensuring a more equitable application of AI. By emphasizing the importance of Bias-Free Analytics, Watson OpenScale empowers organizations to create ethically sound and fair AI systems, ultimately enhancing trust and reliability in automated decision-making.
- Google Cloud AI Platform: Provides resources for fairness and interpretability in AI models.
The foundation of ethical AI relies on transparency and fairness, particularly in model development. Key resources exist within cutting-edge platforms to promote fairness and interpretability in AI models. These tools are essential for organizations aiming for bias-free analytics while ensuring that their models generate equitable outcomes. They enable practitioners to understand and dismantle biases within their datasets, ultimately enhancing the trustworthiness of AI-driven decisions.
One significant advantage of these platforms is their capability to analyze model performance across diverse demographic groups. This analysis helps highlight any bias present, guiding data scientists in refining their models. Furthermore, interpretability features provide insights into how models make predictions, fostering accountability and allowing stakeholders to assess ethical implications. By prioritizing fairness and transparency, organizations can move closer to ensuring that their AI systems operate without bias, leading to more equitable results and user satisfaction.
- Microsoft Fairlearn: An open-source toolkit for assessing and improving the fairness of AI systems.
In the pursuit of Bias-Free Analytics, addressing algorithmic fairness has become crucial in AI development. One effective strategy involves utilizing an open-source toolkit that assesses and improves fairness within AI systems. This resource empowers developers and organizations to identify potential biases inherent in their models. By offering tools for evaluating the fairness of algorithms, it makes the journey toward bias-free analytics more accessible and systematic.
To begin with, the toolkit includes methodologies for assessing various fairness metrics throughout the model's lifecycle. Next, it provides features to improve model outcomes, allowing users to implement adjustments based on the insights gained. By integrating this toolkit into the development process, practitioners can systematically analyze their AI systems' biases, ensuring ethical standards are met. Consequently, this transformative approach fosters the creation of AI solutions that are not only effective but also equitable and just.
- TensorFlow Model Analysis: Allows for the evaluation and visualization of fairness metrics.
TensorFlow Model Analysis (TFMA) plays a pivotal role in achieving Bias-Free Analytics by facilitating the evaluation and visualization of fairness metrics. This tool allows data scientists to assess their machine learning models comprehensively, focusing on fairness across different demographic groups. By employing TFMA, practitioners can identify potential areas where their models may exhibit biases, ensuring that the systems they deploy do not inadvertently reinforce existing inequalities.
Moreover, TFMA supports various metrics, including statistical parity and equal opportunity, allowing users to visualize how their models perform relative to these standards. By incorporating these insights, organizations can make informed adjustments to their algorithms, fostering more ethical decision-making. This commitment to analyzing fairness criteria exemplifies the importance of proactive measures in data analysis, emphasizing the need for constant vigilance against algorithmic bias. In doing so, it ultimately helps create a more equitable framework for AI implementations in diverse applications.
Conclusion: Achieving Bias-Free Analytics in Ethical AI
To achieve bias-free analytics in ethical AI, organizations must prioritize transparency and accountability throughout the data analysis process. This journey requires a continuous commitment to recognizing sources of bias and implementing robust ethical guidelines for data collection. By fostering an environment where diverse perspectives are valued, businesses can significantly mitigate biases in their algorithms.
Ultimately, the pursuit of bias-free analytics hinges on a collaborative effort to design and test models that emphasize fairness. By adhering to these principles, organizations can build AI systems that serve all users equitably, resulting in more accurate and trustworthy insights. This dedication to ethical AI not only enhances data integrity but also cultivates consumer trust, fostering a more inclusive digital landscape.