AI Test Reporting in usability testing is a game-changer, enhancing how teams evaluate applications and websites. Imagine a world where your testing feedback is generated automatically, allowing immediate access to insights that were once time-consuming to compile. These reports not only provide a snapshot of user experiences but also help in streamlining development processes.
The importance of understanding AI Test Reporting cannot be overstated. It enables organizations to grasp user behavior patterns swiftly and effectively. By automating the reporting process, teams can focus more on strategic improvements rather than getting bogged down in data collection. Such advancements significantly heighten the overall quality of usability testing efforts, ultimately leading to better user experiences and enhanced product performance.
Transcribe & extract insights from interviews. At Scale.
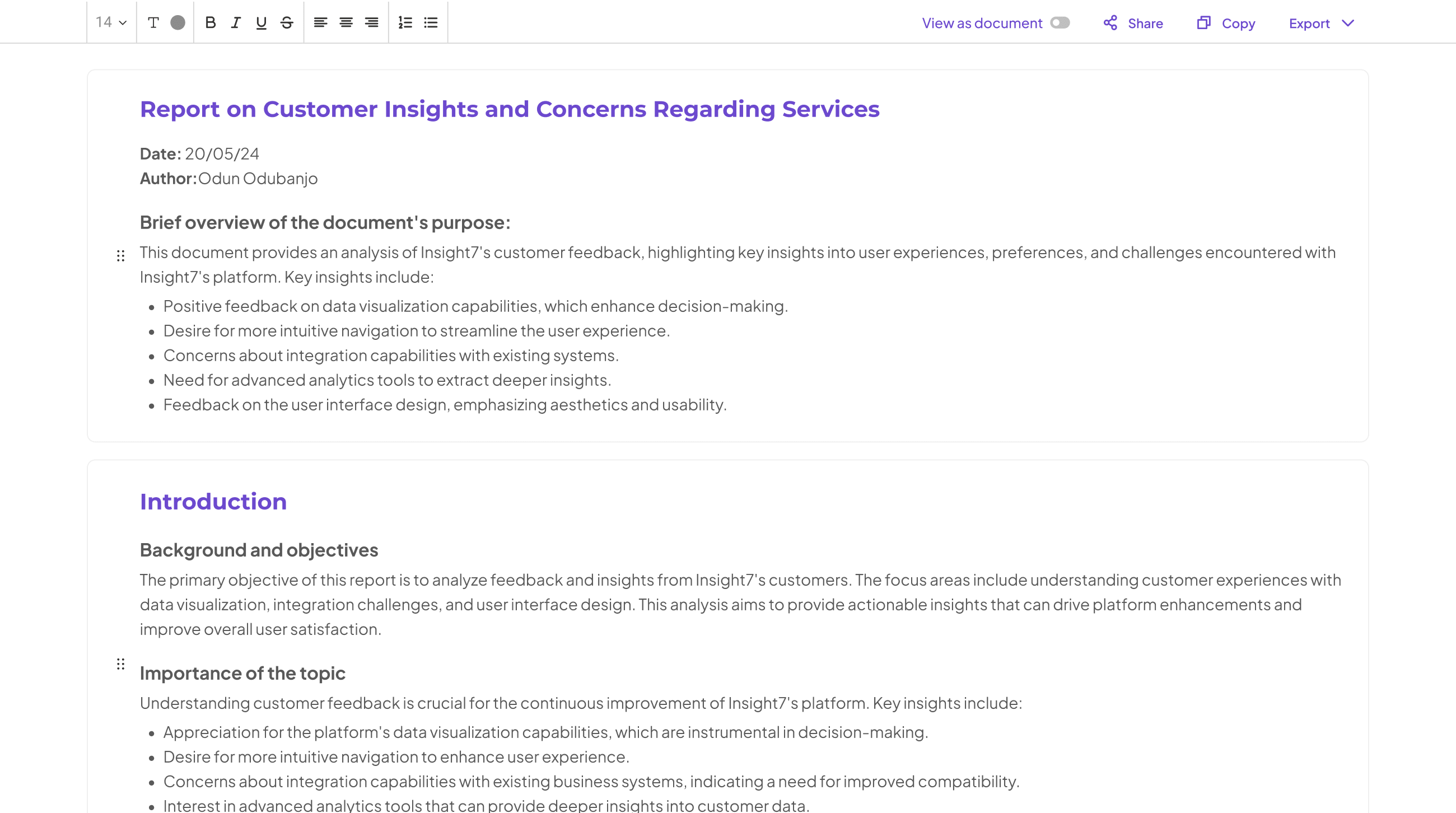
Key Features of AI Test Reporting in Usability Testing
In the realm of usability testing, AI Test Reporting stands out as a game-changing tool. The core features enhance both efficiency and accuracy in capturing user interactions. Firstly, automation significantly reduces the time spent on manual reporting. Testers can focus more on analyzing data and less on compiling it. By generating reports quickly, teams can expedite the decision-making process, ensuring that user feedback is acted upon promptly.
Secondly, AI-driven analytics improve data accuracy. These systems analyze large volumes of data to identify patterns and trends that might be missed through traditional methods. Enhanced reporting capabilities allow for detailed insights into user behavior and product performance, providing teams with actionable information. Additionally, customizable report formats ensure that findings align with specific client needs, facilitating a more tailored approach to usability testing outcomes. Overall, the key features of AI Test Reporting in usability testing create a robust framework that supports continuous improvement of user experiences.
Automation and Efficiency
Automation and efficiency are crucial components in the world of AI test reporting. By automating the reporting process, teams can significantly reduce the time and effort spent on manual data compilation. This efficiency allows for more focus on critical analysis instead of mundane tasks, ensuring that valuable insights are prioritized.
Additionally, AI test reporting streamlines the workflow by generating reports at scheduled intervals, such as monthly or weekly. This consistency ensures that stakeholders receive timely updates on usability testing outcomes without delay. The elimination of manual errors further enhances data accuracy, making the insights derived from these reports more reliable. When organizations embrace automation, they not only save resources but also enhance their capacity to make informed decisions based on real-time data, thus driving improved product outcomes.
Enhanced Data Accuracy
Accurate data collection is essential for any usability testing process. Enhanced data accuracy ensures that the insights derived from AI test reporting are reliable and actionable. By using advanced algorithms and sophisticated data processing techniques, businesses can gain deeper insights into user behavior and preferences. Employing these methods helps organizations make informed decisions based on high-quality data.
To achieve enhanced data accuracy, there are several key factors to consider:
Comprehensive Data Collection: Gathering data from diverse sources can provide a holistic view of user interactions. This diversity contributes to richer insights, allowing for better understanding and analysis.
Real-time Feedback Mechanisms: Implementing real-time feedback tools enables quick adjustments based on user interactions. Prompt responses can lead to more accurate results in usability assessments.
Regular Data Review and Calibration: Continuous review of collected data and regular calibration of data processing systems ensure ongoing accuracy. This helps in adapting to changing user behaviors and market dynamics.
By focusing on these aspects, organizations can significantly improve the accuracy of their AI test reporting, ultimately leading to more effective outcomes in usability testing.
[hanafe_template id=50845]How AI Usability Testing Report Generator Works
The AI Usability Testing Report Generator operates through a well-structured sequence of steps designed to streamline the process of AI test reporting. Initially, the system focuses on gathering data, which typically comes from various sources such as user interactions and feedback during testing sessions. Each interaction is analyzed to collect vital metrics that reflect user behavior and system performance. The accuracy of these insights is paramount, as they serve as the foundation for subsequent evaluation.
Next, the data processing stage involves analyzing the gathered information using advanced algorithms. These algorithms classify interactions and highlight key performance indicators, providing a clearer picture of usability issues. After processing, the system generates comprehensive reports that summarize findings, user engagement levels, and overall performance metrics. These reports can be tailored for different stakeholders, allowing for easy adjustments and edits as needed. Ultimately, this automated approach within the AI usability testing framework ensures timely and accurate AI test reporting, enabling teams to make informed decisions swiftly.
Step 1: Gathering Data
In the process of AI Test Reporting, the first critical step is gathering data. This phase is essential because the quality and relevance of the data collected can significantly impact the insights derived from the analysis. Start by identifying the key metrics you want to capture, such as user interactions and feedback during usability testing sessions. This foundational stage informs subsequent analysis and reporting, ensuring that meaningful patterns surface.
Next, employ various methods to collect data effectively. Options include surveys, interviews, and direct observation during testing sessions. Choose approaches that suit your target audience and testing goals. Once gathered, this data will serve as a rich source of insights that can highlight user experiences, frustrations, and areas for improvement. By meticulously managing this step, you set the stage for comprehensive evaluation and informed decision-making, illustrating the value of AI test reporting in usability testing.
Step 2: Data Processing
The second pivotal step in the AI Usability Testing Report Generator is data processing. This phase transforms raw data into actionable insights, a crucial component of AI test reporting. It begins by organizing the collected data, ensuring clarity and ease of access. Various techniques can be applied here, including statistical analysis and data visualization, to identify patterns and trends that inform product development.
In this stage, users can employ advanced querying tools. These tools facilitate comparisons across different datasets, such as analyzing feedback from specific locations or product versions. The results offer a deeper understanding of user sentiments. Ultimately, effectively processing data paves the way for generating comprehensive reports, enhancing strategic decision-making and improving product outcomes. By effectively transforming raw feedback into structured insights, the process sets the foundation for informed product decisions and improved user experience.
Step 3: Report Generation
The report generation phase is where AI test reporting truly comes to life. Once the data is gathered and processed, it transforms into a structured format ready for interpretation. At this stage, stakeholders can access insights on user experiences and usability metrics in an easily digestible manner. This enables decision-makers to align their strategies closely with user feedback.
A well-designed reporting mechanism can include customizable parameters. Users can receive monthly summaries or detailed analytics depending on their needs. This flexibility ensures that the reports provide relevant information tailored to specific project demands. Additionally, automating the generation process minimizes human error and saves valuable time. Overall, effective report generation serves as a cornerstone in harnessing AI's capabilities to drive actionable insights and improve usability in testing.
Top AI Usability Testing Tools
AI usability testing involves evaluating how well an AI system performs in real-world scenarios. Selecting the right tools for this process is crucial, as they streamline the evaluation and reporting of usability insights. Various AI usability testing tools can help you generate effective AI test reporting, allowing teams to gather data seamlessly and analyze user interactions accurately.
insight7: This tool provides deep insights through advanced analytics and helps teams understand user behavior better.
TestRail: Known for its project management capabilities, TestRail allows testing teams to track and manage usability tests efficiently.
UserTesting: This platform connects with real users, providing qualitative feedback about the usability of AI applications.
Loop11: A fantastic option for remote usability testing, Loop11 supports automated report generation, enhancing the speed of decision-making.
Lookback: This tool focuses on user experience through live feedback, helping form insights into AI usage in real-time scenarios.
Each tool offers unique benefits that enhance the process of AI test reporting, ensuring teams can deliver actionable insights effectively.
insight7
The effective use of AI Test Reporting is crucial in enhancing the usability testing process. Organizations today are inundated with customer signals, and understanding these signals in a timely manner is essential. Traditional manual methods fall short, making it difficult to analyze data quickly and draw actionable insights. This gap opens the door for automated tools that streamline reporting processes, ensuring a more efficient path from data collection to insight generation.
To address common challenges, several vital issues can be highlighted:
Time Consumption: Manually analyzing interview transcripts and user feedback can take valuable hours, delaying decision-making.
Fragmented Insights: When insights are scattered across multiple platforms or files, collaboration becomes inefficient, creating silos of information.
Actionable Strategies: Without a structured reporting tool, translating data into practical business strategies can seem insurmountable.
By adopting AI Test Reporting, organizations can simplify their processes and focus on what truly mattersโturning customer insights into strategic actions that drive success.
TestRail
TestRail serves as a powerful platform for managing test cases and reporting on testing activities. It streamlines the process, making it easier for teams to document their testing efforts and track results. By integrating with various testing tools, TestRail enables consistent collection and organization of data across multiple testing cycles. This seamless integration supports effective collaboration among team members and helps maintain accountability throughout the testing process.
In the context of AI Test Reporting, TestRail can significantly enhance reporting accuracy. The platform allows users to generate detailed reports quickly, showcasing key metrics and insights derived from testing activities. This capability not only saves time but also makes it easier for stakeholders to understand the quality of products. Ultimately, utilizing TestRail can aid in delivering thorough and insightful AI test reporting, improving decision-making and ensuring higher-quality outcomes.
UserTesting
UserTesting plays a critical role in the realm of AI usability testing. This process involves evaluating user interactions with a product to collect valuable insights. With the advent of AI test reporting, organizations can streamline how they gather and interpret user feedback. It allows for a systematic exploration of how real users navigate and utilize products, generating data that reveals patterns and areas needing improvement.
The application of AI in this space transforms traditional methods into more effective practices. By leveraging AI technologies, companies can analyze user interactions at scale and generate comprehensive reports. This automation enables faster decision-making and enhances overall user experiences. Ultimately, thorough user testing using AI test reporting facilitates continuous improvement, helping organizations to stay aligned with user needs and preferences. This approach not only enriches the usability of products but also nurtures customer satisfaction and loyalty.
Loop11
Loop11 offers a unique perspective on AI test reporting by providing a robust platform for usability testing. Users can conduct tests and gather insights seamlessly, enriching the user experience. The tool enables researchers to set up tests that can engage participants effortlessly, ensuring that valuable feedback is collected. This feedback loops back into the system, creating a cycle that enhances the overall testing process.
Moreover, Loop11 simplifies data analysis, allowing for clear visualizations of user experience metrics. Participants' journeys are tracked meticulously, presenting researchers with actionable insights. This way, stakeholders can make informed decisions based on concrete data. By integrating AI into the usability testing workflow, Loop11 not only streamlines data collection but also elevates the quality of AI test reporting. Its user-centric approach reinforces the commitment to optimizing every aspect of usability testing.
Lookback
Lookback enables users to gain deeper insights into their usability testing processes by providing robust analysis features. This tool offers real-time feedback during user sessions, ensuring a dynamic and interactive approach to understanding user behavior. By recording and analyzing interactions, Lookback allows teams to identify usability issues and enhance the overall user experience.
With AI test reporting capabilities, Lookback transforms raw user data into actionable insights. It systematically processes user interactions, highlighting key trends and areas for improvement. Teams can easily evaluate participants' feedback, aligning it with objective performance metrics. This combination of subjective and objective data promotes data-driven decision-making, enhancing usability testing outcomes and ultimately product success.
Conclusion: The Future of AI Test Reporting in Usability Testing
The future of AI test reporting in usability testing appears promising and transformative. As technology continues to evolve, AI-generated reports are expected to become increasingly sophisticated and tailored to user needs. With the ability to analyze vast amounts of data instantly, AI test reporting can provide insights that were previously difficult to obtain. This shift will not only enhance the reporting process but will also unlock opportunities for deeper user understanding.
In this landscape, organizations can expect more automated, accurate, and user-centric reports. By streamlining the reporting process, teams can focus on actionable insights rather than data collection. This will empower decision-makers to utilize AI test reporting effectively, ultimately leading to improved user experiences and more successful products in the long run. Embracing these advancements will be essential for staying competitive in the evolving market.