The rapid evolution of technology has led to significant advancements in healthcare, with Healthcare AI Innovations emerging as a cornerstone in research. These innovations promise to enhance diagnostic accuracy, streamline clinical workflows, and personalize patient care. As researchers embrace AI tools, they unlock new potentials for analyzing complex data, resulting in groundbreaking discoveries that were previously unattainable.
The application of AI in healthcare research is not merely a trend; it signifies a fundamental shift in how medical knowledge is generated and applied. By harnessing AI capabilities, researchers can identify patterns in large datasets, predict outcomes, and improve efficiency in clinical trials. This transformative approach empowers healthcare professionals to make informed decisions, ultimately leading to better patient outcomes and a new era of precision medicine.
Analyze qualitative data. At Scale.
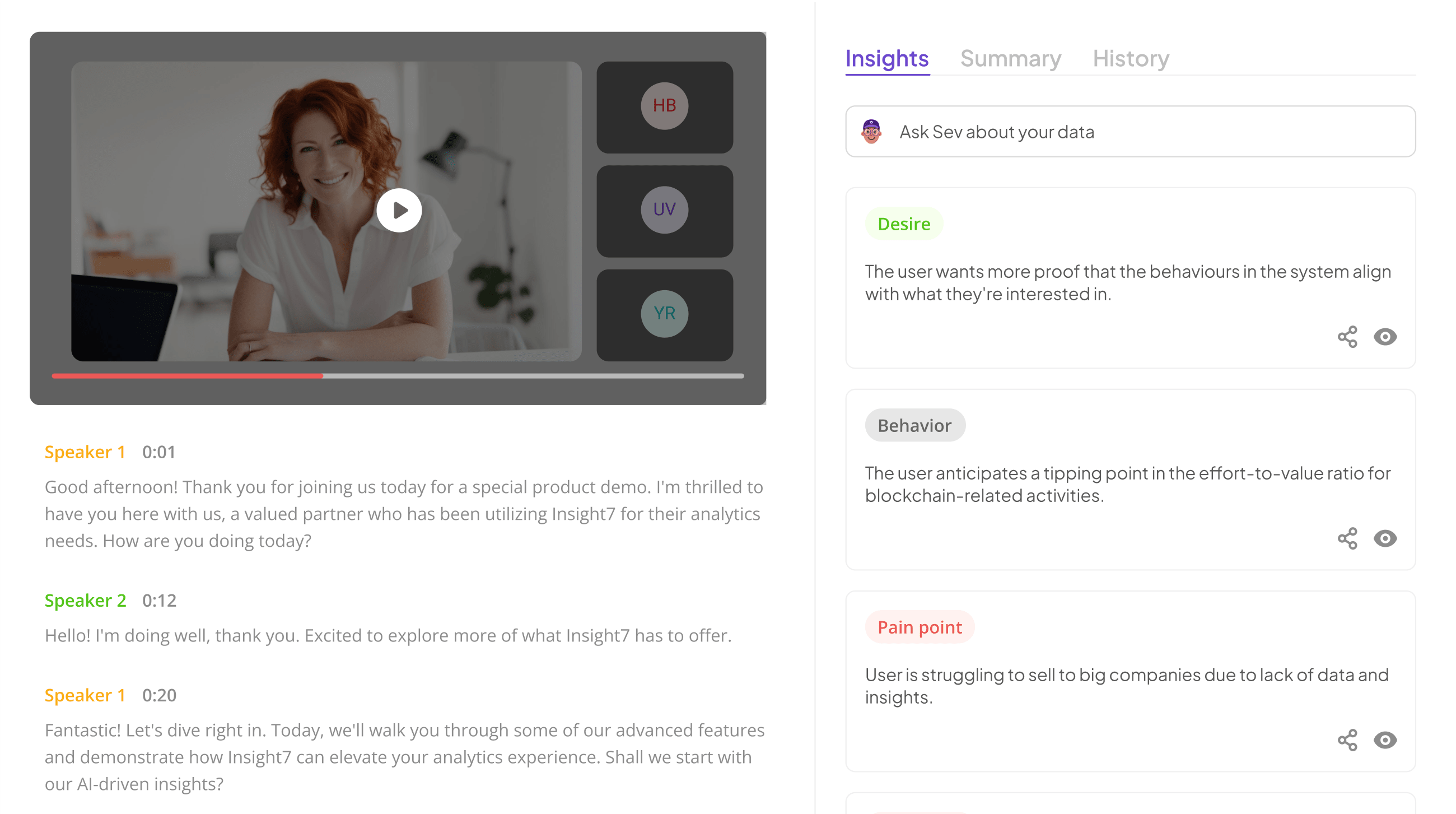
The Role of Healthcare AI Innovations in Diagnostics
Healthcare AI innovations play a transformative role in the realm of diagnostics, revolutionizing how medical professionals detect and analyze diseases. These advanced technologies utilize machine learning algorithms to sift through vast datasets, identify patterns, and make predictions that assist in early diagnosis. By enhancing accuracy and efficiency, these innovations reduce the burden on healthcare providers and enable them to offer tailored treatment to patients.
One noteworthy application is in medical imaging, where deep learning techniques improve image interpretation, ensuring that subtle abnormalities are not missed. Accurate readings from X-rays or MRIs can significantly impact patient outcomes by hastening treatment decisions. Additionally, AI-driven tools can automate routine tasks, thus allowing healthcare professionals to focus on more complex diagnostic challenges. Ultimately, integrating Healthcare AI innovations into diagnostics signifies a substantial leap toward personalized medicine, improved patient care, and a more effective healthcare system.
Machine Learning Techniques in Disease Detection
Machine learning techniques play a pivotal role in disease detection, enhancing the accuracy and speed of diagnoses. Healthcare AI innovations rely on various algorithms, enabling healthcare professionals to analyze vast datasets efficiently. These datasets include patient symptoms, medical histories, and even genetic information, allowing for comprehensive analysis and prediction of disease outcomes.
The primary approaches in machine learning for disease detection include supervised learning, unsupervised learning, and reinforcement learning. Supervised learning algorithms are trained on labeled data, helping to identify patterns linked to specific diseases. Unsupervised learning, on the other hand, detects hidden patterns in unlabeled data, providing insights into unknown health issues. Reinforcement learning mimics trial and error, optimizing decision-making processes in diagnostics. By incorporating these techniques, healthcare professionals can leverage AI innovations to improve patient care significantly, leading to more personalized and effective treatment strategies.
Deep Learning Applications in Medical Imaging
Deep learning applications in medical imaging represent a significant advancement in healthcare AI innovations. With the ability to analyze complex visual data, these tools have transformed diagnostics and patient care. Using convolutional neural networks, deep learning algorithms can identify patterns in medical images, such as CT scans and MRIs, facilitating early disease detection.
The effectiveness of these applications can be summarized in three key areas. First, they enhance diagnostic accuracy by reducing human error. Second, they enable faster processing of vast amounts of image data, providing timely insights for healthcare professionals. Lastly, they support personalized treatment plans by predicting patient responses based on imaging characteristics. As healthcare continues to embrace AI innovations, deep learning in medical imaging will undoubtedly play a crucial role in enhancing patient outcomes and streamlining healthcare workflows.
Extract insights from interviews, calls, surveys and reviews for insights in minutes
Top AI Tools Revolutionizing Healthcare Research
Advancements in healthcare research are significantly driven by innovative AI tools. These technologies are reshaping various aspects of medical research and diagnostics, facilitating faster, more accurate outcomes. Among the notable tools, TensorFlow powers predictive analytics, allowing for real-time insights into patient data. This innovation aids researchers in forecasting disease trends and improving treatment protocols.
Additionally, platforms like IBM Watson Health bring advanced AI capabilities to the forefront, enabling deep data analysis and generating actionable medical insights. Google Health AI is also noteworthy, as it revolutionizes patient care through enhanced imaging analysis and decision-making support. Furthermore, PyTorch Healthcare accelerates research efforts by providing a robust framework for developing complex models, ultimately contributing to breakthroughs in clinical research. Collectively, these healthcare AI innovations showcase a powerful movement towards more efficient and effective medical research practices.
insight7: Breaking New Ground in Healthcare AI Innovations
Healthcare AI innovations are at the forefront of transforming how we approach patient care and research. This evolution is primarily driven by the need for more efficient data analysis and interpretation. With traditional methods falling short in keeping pace with the rapid influx of data, AI solutions offer streamlined approaches to extracting valuable insights from vast datasets.
The innovative technologies being implemented are reshaping diagnostics, therapeutic strategies, and overall patient outcomes. AI tools now assist healthcare professionals in identifying patterns and anomalies that would have been missed with manual analysis. As these innovations continue to develop, healthcare providers can expect improved efficiency in decision-making processes, ultimately leading to safer, more effective patient care strategies. Embracing these advancements signifies a commitment to progress in the healthcare sector, ensuring that we meet the growing demands for high-quality health services.
TensorFlow: Enhancing Predictive Analytics in Healthcare
In the realm of healthcare, TensorFlow plays a pivotal role in enhancing predictive analytics, driving forward Healthcare AI Innovations. By employing this powerful framework, healthcare professionals can analyze vast amounts of patient data to uncover patterns that inform timely decision-making. This capability is crucial for predicting patient outcomes, optimizing treatment plans, and improving overall patient care.
TensorFlow specializes in handling multi-dimensional data, enabling the development of sophisticated predictive models. For instance, through the use of neural networks, healthcare data can be processed to foresee potential health risks before they manifest. Moreover, the integration of TensorFlow facilitates the implementation of real-time analytics, empowering clinicians with insights that are both actionable and reliable. As the healthcare sector continues to evolve, embracing these advanced analytics tools will be essential to enhance efficiency and patient outcomes.
IBM Watson Health: Advanced AI for Medical Insights
Advanced AI tools are transforming the landscape of healthcare research by generating vital medical insights. They help in synthesizing vast amounts of data to facilitate better clinical decisions. With the integration of state-of-the-art algorithms, caregivers can analyze patient data efficiently, leading to enhanced diagnostic accuracy and personalized treatment plans.
The innovative applications of AI in healthcare range from predictive analytics to image processing. For instance, automated systems can analyze medical images at a speed and accuracy that surpass human capabilities. These systems also assist in identifying disease patterns and predicting patient outcomes, thus revolutionizing the way healthcare providers approach patient management. Advanced AI for medical insights not only increases operational efficiency but also substantially improves patient care, making it a vital part of modern healthcare research. As healthcare AI innovations continue to evolve, they promise to further bridge the gap between technology and patient care, enhancing overall health outcomes.
Google Health AI: Transforming Patient Care
In the realm of Healthcare AI Innovations, patient care is undergoing dramatic transformations. These technological advancements aim to enhance the overall healthcare experience, improving accuracy and efficiency in treatment delivery. The integration of AI tools in patient care helps transform clinical workflows while ensuring that healthcare professionals can focus more on patient interactions rather than administrative tasks.
One of the significant impacts of AI in healthcare is its capacity to analyze vast amounts of data swiftly. For example, AI algorithms can scrutinize medical records, lab results, and imaging studies to identify patterns that humans might overlook. This can lead to early diagnosis and personalized treatment plans that cater to individual patient needs. Consequently, improved patient outcomes and satisfaction become possible as AI continues to evolve and redefine the capabilities of healthcare systems. Embracing these AI innovations will ultimately shape a more responsive and effective healthcare environment.
PyTorch Healthcare: Accelerating Research with AI
In recent years, PyTorch has emerged as a pivotal player in the healthcare AI innovations landscape. By providing an accessible framework, it accelerates research across various medical fields. Researchers harness its capabilities to develop sophisticated algorithms that improve diagnostics, treatment plans, and patient outcomes. This transformative potential has led to groundbreaking advancements in understanding complex diseases and optimizing healthcare workflows.
The versatility of PyTorch enables the deployment of innovative models that can analyze vast datasets efficiently. Researchers can experiment with different architectures, enhancing their ability to create tailored solutions for unique healthcare challenges. As teams collaborate globally, PyTorch fosters an environment where AI-driven insights can be rapidly translated into clinical applications. This continuous feedback loop not only enhances research efforts but also ensures that the benefits of healthcare AI innovations are felt across communities, paving the way for improved patient care.
Conclusion: The Future of Healthcare AI Innovations
The ongoing advancements in healthcare AI innovations signal a transformative period for medical research and patient care. As technology evolves, the integration of AI tools will enhance diagnostic accuracy and streamline research processes. This fusion of artificial intelligence and healthcare promises not only improved patient outcomes but also more efficient resource management within healthcare systems.
Looking ahead, the potential for AI in healthcare is immense. From predictive analytics that anticipate patient needs to powerful imaging techniques that enhance diagnostic capabilities, the future is bright. As we continue to develop and implement these innovations, a more personalized and responsive healthcare environment will emerge, ultimately improving the quality of care for all patients.