In the world of research, data serves as a powerful tool for making informed decisions. As researchers delve deeper into their studies, the need for effective analysis becomes paramount. Top data analysis tools streamline the process, allowing for easier extraction of insights from complex datasets. The ability to discern patterns and trends can significantly influence research outcomes, ultimately guiding strategic decisions.
The selection of the right tool can also enhance the overall productivity of research projects. Whether you're a seasoned researcher or just starting, understanding the capabilities of these tools is essential. In this section, we will explore the best examples of data analysis tools that stand out in the field, helping researchers unlock the full potential of their data.
Analyze qualitative data. At Scale.
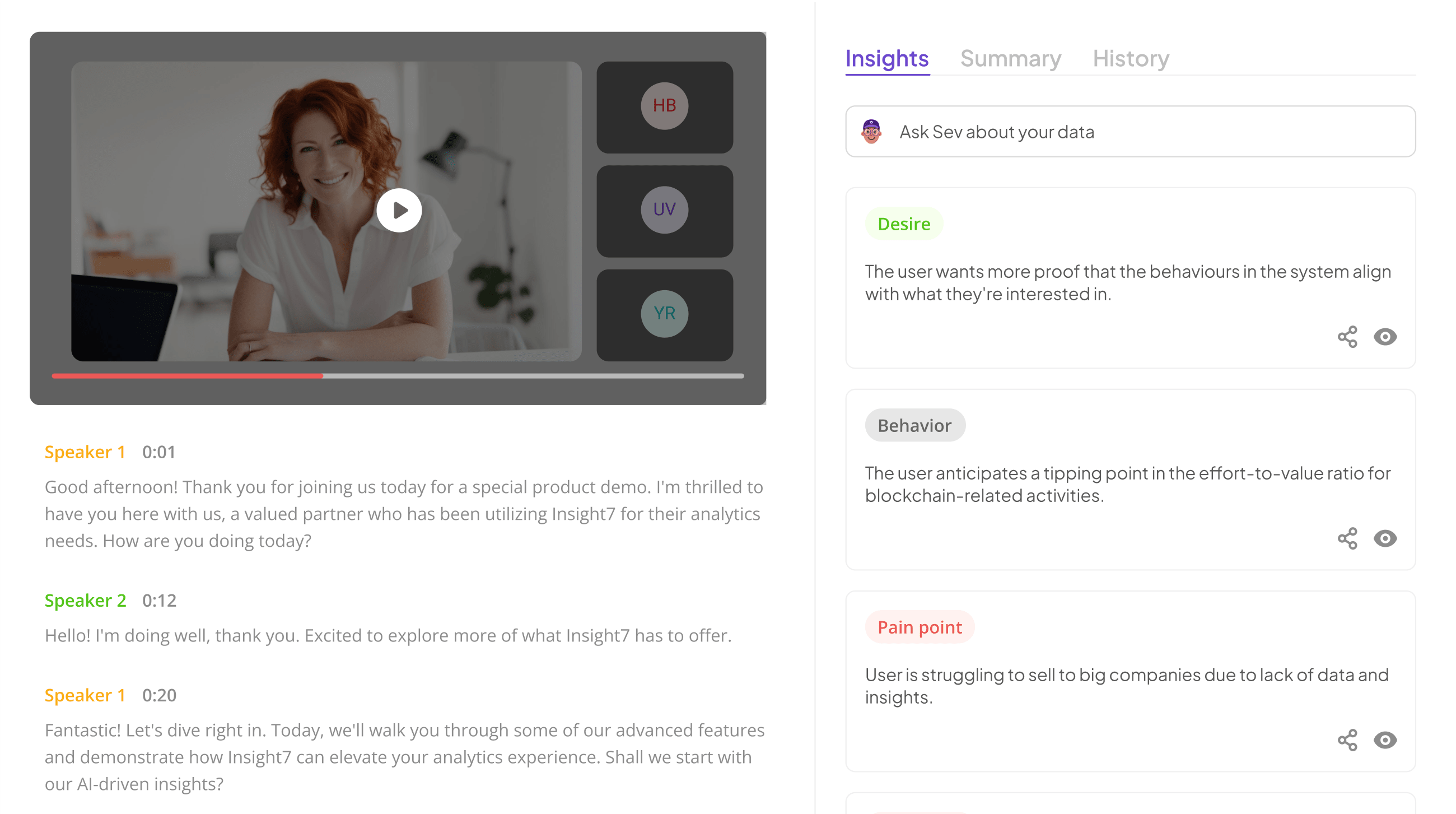
Understanding the Importance of Data Analysis Tools
Data analysis tools play a pivotal role in transforming raw information into meaningful insights. The importance of these tools in research cannot be overstated, as they facilitate the identification of trends, patterns, and relationships within data. Proper analysis not only aids in informed decision-making but also enhances the overall efficiency of the research process.
Top Data Analysis Tools enable researchers to visualize data and derive actionable insights that might otherwise remain hidden. By effectively utilizing these tools, researchers can compare different data sets, uncover anomalies, and understand consumer sentiments. This process is essential for optimizing product development and improving customer experiences. The right data analysis tool can streamline workflows, improve accuracy, and ultimately lead to more successful research outcomes. In an era where data-driven decision-making is paramount, mastering these tools is essential for any researcher aiming to achieve impactful results.
Why Top Data Analysis Tools are Crucial for Research
Top Data Analysis Tools play a pivotal role in research, serving as the backbone of effective data-driven decision-making. They help researchers sift through large volumes of data and extract meaningful insights, minimizing the risk of error and bias. As research methodologies become increasingly complex, these tools facilitate deeper analysis, enabling researchers to identify patterns and trends that may not be immediately evident. This analytical proficiency can lead to groundbreaking discoveries and informed strategies.
Moreover, utilizing top data analysis tools empowers researchers to visualize their findings comprehensively. With features such as dynamic dashboards and customizable reports, the presentation of data becomes clearer and more impactful. It encourages collaboration and communication among teams, supporting an informed approach to resource allocation and strategic development. Ultimately, adopting the right data analysis tools elevates the quality of research, ensuring that insights gleaned are not only accurate but also actionable in driving progress.
How to Choose the Right Tool for Your Research Needs
Choosing the right tool for your research needs can significantly influence the quality and efficiency of your results. Start by identifying the specific requirements of your research project. Consider factors such as the type of data you will analyze, the complexity of your analysis, and your level of expertise with data analysis software. The Top Data Analysis Tools vary widely, offering different functionalities and ease of use, so aligning the tool's capabilities with your goals is essential.
Next, assess the tools available within the context of your research. For instance, if you're dealing with large datasets that require powerful analytics and visualization, tools like Tableau or Python may be ideal. Conversely, for more straightforward analyses, Microsoft Excel can suffice. Evaluate each option based on user support, cost, and integration with other software. By taking these steps, you can choose a tool that aligns well with your research demands, ultimately enhancing your analytical capabilities.
Extract insights from interviews, calls, surveys and reviews for insights in minutes
Top Data Analysis Tools for Researchers
Data analysis is an essential part of research, offering insights that drive decision-making and strategic planning. When discussing the top data analysis tools, several software options stand out for their functionalities and user-friendly interfaces. Researchers benefit from tools such as R Programming, which supports advanced statistical analysis, and Python, known for its versatile data manipulation capabilities with libraries like Pandas and NumPy.
Utilizing visualization software like Tableau can greatly enhance data presentation, turning complex datasets into understandable graphics. Additionally, IBM SPSS offers powerful statistical analysis features, making it popular in academic and professional environments. Microsoft Excel remains a staple for many, providing simple yet effective solutions for data organization and preliminary analysis. Each of these tools plays a significant role in improving research efficiency and accuracy, making them indispensable in todayโs data-driven landscape.
Insight7: An Overview and Key Features
Insight7 serves as an innovative platform designed to simplify the data analysis process for researchers. It allows users to engage with data effortlessly, ensuring that insights can be derived without extensive training. This user-centric approach helps democratize access to valuable research findings, making it suitable for everyone, regardless of their expertise in data analysis.
One of the standout features of Insight7 is its intuitive library interface, where all files are stored and can be easily accessed for analysis. Users can transcribe calls, evaluate customer experiences, and pinpoint friction points in a seamless manner. The platform excels in extracting significant insights such as pain points, desires, and behaviors from customer interactions. With the ability to group multiple files into projects, researchers can analyze large sets of data efficiently. Overall, Insight7 is a powerful tool in the realm of data analysis for research, providing actionable insights with minimal effort.
Additional Tools Enhancing Research Efficiency
To bolster research efficiency, several tools can act as powerful allies in the data analysis process. One significant aspect to consider is the interoperability of these tools, which allows researchers to streamline workflows. Utilizing software like R Programming or Python with libraries such as Pandas and NumPy, researchers can efficiently process and analyze complex datasets, drawing meaningful insights with relative ease.
Furthermore, tools like Tableau and IBM SPSS offer advanced visualization capabilities that enable researchers to present their data in compelling and understandable formats. Microsoft Excel, despite being a more traditional tool, still remains essential for data organization and initial analysis. By integrating these various tools, researchers can enhance their analytical capabilities, leading to more efficient, reliable results that can ultimately drive informed decision-making within their fields.
- R Programming
R Programming has emerged as one of the top data analysis tools in research, primarily due to its powerful statistical capabilities and rich visualization options. Using R, researchers can manipulate, model, and visualize data efficiently. It offers an array of packages tailored for various analytical needs, making it versatile across different research fields.
One of the most significant advantages of R is its community support and comprehensive documentation. This user-friendly environment allows researchers, even those new to programming, to quickly learn and apply techniques. Furthermore, R's open-source nature ensures that users have access to a wide array of packages for specialized tasks, from data cleaning to advanced statistical modeling. Utilizing R Programming can significantly enhance the depth and quality of analysis in research projects, ultimately enabling researchers to derive actionable insights from their data.
- Python (with Pandas and NumPy)
Python has emerged as one of the top data analysis tools because of its versatility and ease of use. With libraries like Pandas and NumPy, researchers can efficiently manipulate and analyze large datasets. Pandas, known for its powerful data manipulation capabilities, allows users to work with structured data with ease by providing dataframes and simple methods for filtering, aggregating, and cleaning data. NumPy, on the other hand, focuses on numerical calculations, offering an array of high-performance mathematical tools essential for analyzing research data.
Together, these libraries empower researchers to perform complex operations and statistical analysis, making data-driven insights more accessible. For instance, they can seamlessly handle missing data, run statistical tests, and visualize results, all within a single Python environment. This integration of data handling, analysis, and visualization underlines why Python, with Pandas and NumPy, is regarded as one of the top data analysis tools in research, facilitating informed decisions and deeper insights.
- Tableau
Tableau plays a pivotal role among the top data analysis tools, offering researchers powerful visualization capabilities. With its user-friendly interface, Tableau allows users to create interactive and shareable dashboards, simplifying complex data insights. This enables researchers to uncover patterns, trends, and relationships that might otherwise remain hidden when relying solely on raw data.
Researchers benefit from Tableau through features like drag-and-drop functionality, which facilitates swift data manipulation. Furthermore, its ability to connect to multiple data sources enhances collaborative analysis, making it easier to combine various datasets for a comprehensive view. This helps teams in identifying actionable insights tailored to their research objectives. Whether studying market trends or customer behavior, Tableau equips researchers with the tools necessary to transform data into visual narratives, effectively conveying findings to stakeholders and guiding strategic decisions.
- IBM SPSS
IBM SPSS stands out as one of the top data analysis tools in research due to its robust capabilities and user-friendly interface. Researchers can efficiently manage and analyze large datasets, which is crucial for drawing meaningful insights. With its advanced statistical features, this tool helps in identifying patterns and relationships within the data, allowing for a deeper understanding of the underlying trends.
Moreover, the software offers various visual data representation options, such as charts and graphs, which can enhance the presentation of findings. Users can customize their analyses, making it adaptable to diverse research needs. By leveraging its powerful statistical algorithms, researchers can confidently make informed decisions based on the analyzed data. Overall, incorporating such a powerful tool can significantly elevate the quality and impact of research outcomes.
- Microsoft Excel
Microsoft Excel serves as one of the top data analysis tools due to its versatility and user-friendly interface. Researchers consistently turn to Excel for tasks ranging from simple calculations to complex data analysis. Its ability to handle large datasets makes it invaluable for organizing and interpreting data efficiently. With features like pivot tables and charts, users can visualize trends and patterns that emerge from their findings, thus enhancing the clarity of their insights.
In addition to basic functions, Excel allows for advanced statistical analysis and financial modeling. By employing formulas and functions, researchers can conduct in-depth analyses, making data-driven decisions more effective. Furthermore, Excel supports importing data from various sources, allowing researchers to centralize their data analysis efforts seamlessly. Ultimately, Excel remains a powerful asset in a researcherโs toolkit, delivering significant value within the realm of data analysis.
Steps to Implement Top Data Analysis Tools in Research
Implementing top data analysis tools in research begins with identifying your specific research objectives. Clear objectives guide the selection of an appropriate tool that aligns with the goals of your study. Once the objectives are established, the next step is to choose the right tool for data collection. Whether you're opting for R programming or Python, each tool offers unique features suitable for different types of data and methodologies.
After selecting the tool, focus on data processing and analysis. This stage is vital for identifying trends and patterns that emerge from the collected data. Finally, interpreting and presenting your findings will allow you to communicate insights effectively. A well-structured presentation ensures that stakeholders understand the results and their implications. By following these steps, researchers can maximize the benefits of the top data analysis tools, ultimately enhancing the research outcomes.
Step 1: Identifying Your Research Objectives
Identifying your research objectives is the foundational step in any data analysis project. Without clear objectives, the entire research process can become vague and misdirected. Begin by reflecting on the specific questions you want to answer. Consider what insights you hope to gain and how they will influence your decisions. Establishing these goals will guide your choice of the top data analysis tools suitable for your research.
Next, prioritize your research objectives based on their importance and feasibility. Are you looking for detailed insights, or do you need high-level summaries? Clarifying these distinctions helps determine the best tools to employ. For instance, if you're focused on data visualization, specific software will serve better than others aimed at statistical analysis. By taking the time to articulate your research objectives, you'll enhance your data analysis outcomes and ultimately improve the quality of your insights.
Step 2: Selecting the Appropriate Tool for Data Collection
Selecting the appropriate tool for data collection is a critical step in the research process. The right tool can greatly enhance the quality and reliability of your findings. You should begin by evaluating your specific research objectives and the type of data you plan to collect. This consideration will guide you in choosing from the top data analysis tools available.
Several factors influence your decision. First, consider the complexity of the data you'll handle. Simple surveys or basic statistical analysis may work well with user-friendly tools like Microsoft Excel. However, for more complex data processing, programming languages like R or Python might be more appropriate. Additionally, think about budget constraints, the expertise of your team, and the scalability of the tool. Understanding these elements will enable you to select a tool that not only meets your current needs but also adapts to future project requirements.
Step 3: Data Processing and Analysis
Data processing and analysis are pivotal elements in extracting meaningful insights from research data. Once data is collected, it is essential to organize and process this information to uncover trends and patterns. Reliable data analysis tools streamline this phase, allowing researchers to visualize relationships within the data.
In this step, some of the top data analysis tools facilitate various analyses. Each tool has unique features designed to assist in identifying significant insights. For instance, tools like Python enhance data manipulation, while Tableau offers dynamic visualizations. Moreover, with IBM SPSS, advanced statistical analyses can be conducted more efficiently. The integration of these tools not only enhances productivity but also ensures that researchers arrive at reliable conclusions, guiding their decisions effectively. Overall, a robust data processing and analysis strategy strengthens the foundation of research outcomes.
Step 4: Interpreting and Presenting Findings
Interpreting and presenting findings is a pivotal step in the data analysis process. By utilizing top data analysis tools, researchers can transform raw data into actionable insights that drive decision-making. This phase involves sifting through the collected data to extract meaningful patterns and trends that effectively communicate the story behind the numbers.
To achieve this, consider focusing on key elements such as clarity, relevance, and engagement. Start by summarizing the data in visual formats, like charts and graphs, which can enhance understanding. Then, articulate the findings clearly, emphasizing significant trends or anomalies. Tailoring your presentation to your audience's needs ensures the insights generated resonate. Ultimately, a well-structured presentation not only informs but also inspires stakeholders to act on the insights derived from your research.
Conclusion: Maximizing Research Potential with Top Data Analysis Tools
In conclusion, maximizing research potential hinges on the effective utilization of top data analysis tools. By integrating these tools, researchers can significantly enhance their ability to derive insightful conclusions and make data-driven decisions. Each tool offers unique features suitable for various research needs, whether you prioritize deep statistical analysis or user-friendly visualizations.
Research that employs these tools can uncover trends and patterns hidden within complex datasets. Ultimately, valuing and mastering the top data analysis tools not only streamlines the research process but also enhances the quality of insights drawn from the data, empowering researchers to achieve their goals with greater confidence.
Analyze qualitative data. At Scale.
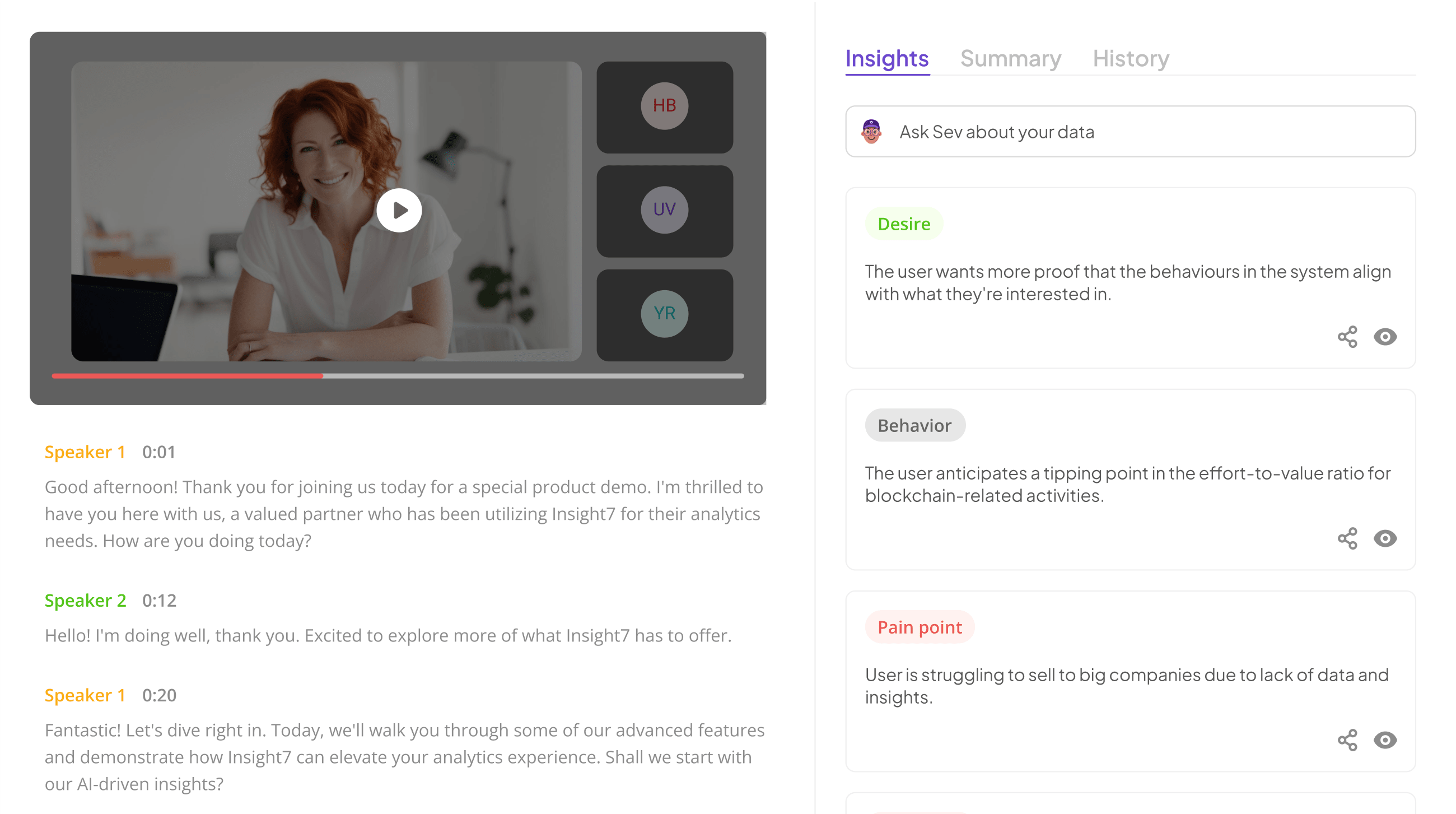