AI-Driven Qualitative Synthesis represents a transformative approach to qualitative research, addressing the challenges of manual data analysis. As researchers face increasing demands for efficiency and accuracy, traditional methods often falter under pressure. The integration of artificial intelligence can streamline the synthesis of qualitative data, fostering quicker insights without compromising depth or rigor.
By leveraging AI capabilities, this innovative methodology enables researchers to analyze diverse datasets rapidly, reduce potential biases, and enhance the overall quality of insights. Understanding AI-Driven Qualitative Synthesis is essential for those looking to harness the power of technology in synthesizing qualitative research from mixed sources effectively. This section will explore its principles, algorithms, and practical applications, setting the stage for deeper exploration.
Analyze qualitative data. At Scale.
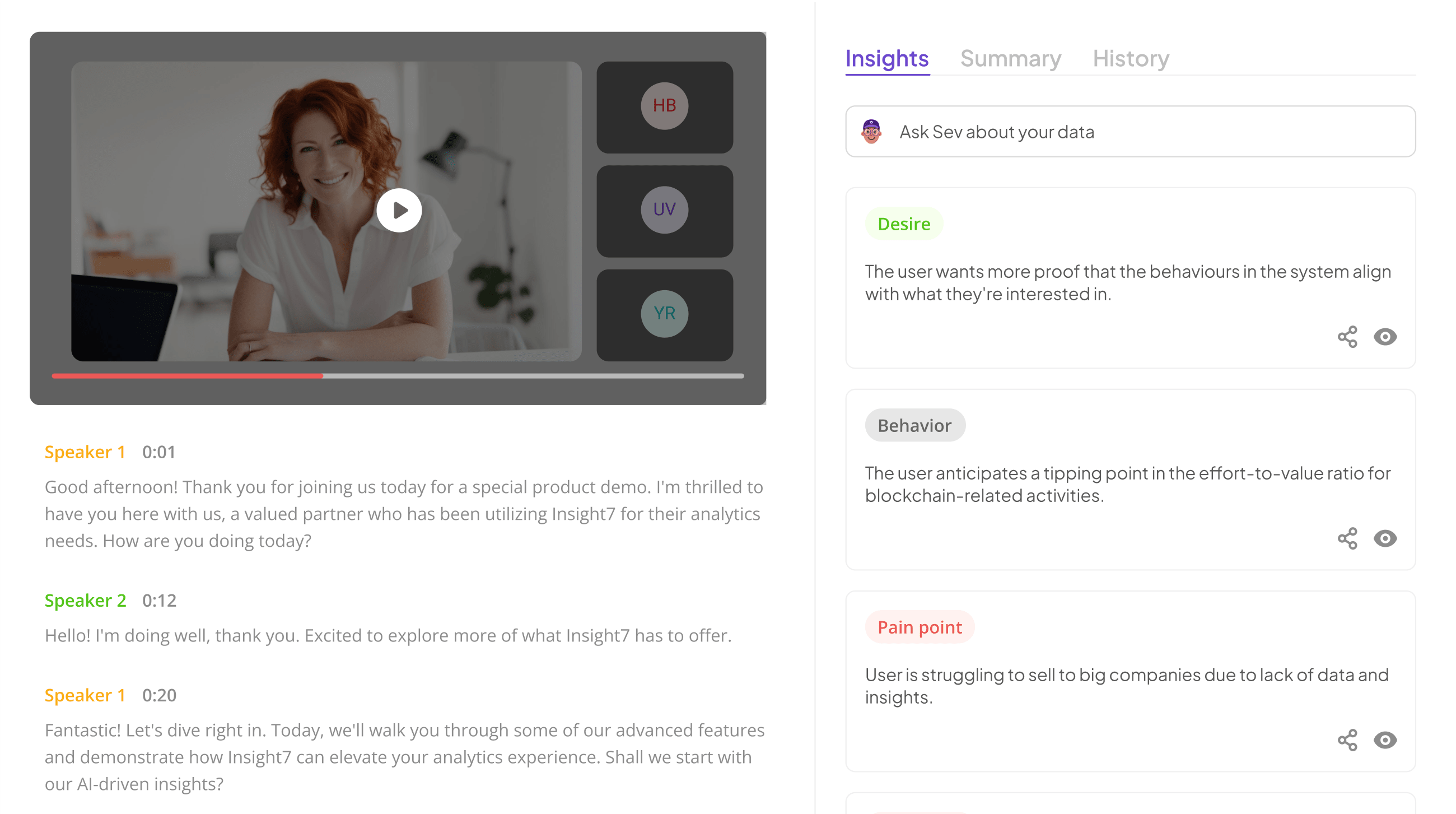
In this section, we will introduce the concept of AI-Driven Qualitative Synthesis and its relevance in synthesizing qualitative research from mixed sources. This provides a foundation for understanding how AI technologies can revolutionize the field of qualitative research.
AI-Driven Qualitative Synthesis revolutionizes the way researchers handle qualitative data by integrating advanced technologies to synthesize insights from mixed sources. This approach addresses common challenges in qualitative research, such as biases and inconsistencies that arise from manual analysis. By leveraging artificial intelligence, researchers can efficiently process large volumes of information, enabling them to derive meaningful insights more rapidly.
One of the key benefits of AI-Driven Qualitative Synthesis is its ability to unify data from various sources, including interviews and open-ended survey responses. This synthesis practice enhances the reliability of findings, as AI tools can identify patterns and themes that might be overlooked by human analysts. Consequently, researchers can spend less time on repetitive tasks and focus on generating actionable insights, paving the way for innovative research methodologies that improve overall qualitative research outcomes.
The Principles of AI-Driven Qualitative Synthesis
AI-Driven Qualitative Synthesis is grounded in several foundational principles that shape its effectiveness and reliability in synthesizing qualitative research. First, the method emphasizes the integration of diverse data sources. By drawing from interviews, surveys, and open-ended responses, researchers can attain a holistic view of the subject matter. This multifaceted approach ensures that insights are comprehensive and encompass multiple perspectives.
Secondly, transparency is crucial. Maintaining clear methodologies and processes allows researchers to understand the algorithms applied in data analysis. This understanding fosters trust in the synthesized results while reducing biases and inconsistencies across the research team. Furthermore, continuous improvement is a core principle. As AI tools evolve, best practices in qualitative synthesis must also adapt to enhance accuracy and insight generation. By adhering to these principles, researchers can effectively harness AI to streamline and elevate the qualitative research process.
Extract insights from interviews, calls, surveys and reviews for insights in minutes
Delve into the core principles that guide the application of AI to qualitative research synthesis. These principles provide the necessary framework for effective integration of AI technologies.
To effectively harness AI-Driven Qualitative Synthesis, it is essential to understand the core principles guiding its application. First, seamless integration requires alignment between AI capabilities and qualitative research objectives. Balancing automated analysis with human intuition ensures a more nuanced understanding of qualitative data. Second, the emphasis on transparency is crucial; researchers must know how algorithms interpret data to maintain reliability and credibility. Third, iterative refinement of AI models is needed to enhance their performance, making sure they evolve with new data and insights.
Additionally, ethical considerations cannot be overlooked. Minimizing bias in algorithmic processing and upholding data privacy are essential steps in fostering trust among research participants. By remaining vigilant about these principles, researchers can effectively leverage AI technologies to synthesize qualitative data, leading to richer insights and innovative outcomes. This principled approach can result in a transformative impact on how qualitative research is conducted and understood in diverse fields.
Key Algorithms in AI-Driven Qualitative Synthesis
In the realm of AI-Driven Qualitative Synthesis, key algorithms play a pivotal role in processing and analyzing qualitative data. Various algorithms, such as natural language processing (NLP) and machine learning, are designed to identify themes and patterns across diverse datasets. These algorithms leverage computational power to significantly reduce the time and effort traditionally associated with qualitative analysis.
Understanding how these algorithms function is essential for researchers looking to streamline their synthesis processes. For instance, supervised learning algorithms allow researchers to train models on annotated data, enhancing the precision of theme identification. On the other hand, unsupervised learning algorithms can automatically cluster similar responses, revealing insights that may not be immediately apparent. As researchers navigate the complexities of mixed-source data, the application of these algorithms not only enhances efficiency but also helps in mitigating biases, leading to more reliable insights. By integrating key algorithms into qualitative research methodologies, researchers can harness the full potential of AI in their synthesis efforts.
Explore the primary algorithms used in AI to synthesize qualitative data, highlighting how these algorithms process and analyze diverse datasets.
AI algorithms play a crucial role in synthesizing qualitative data, effectively transforming diverse datasets into actionable insights. One primary algorithm includes Natural Language Processing (NLP), which analyzes and interprets human language. NLP allows AI systems to identify themes, sentiments, and contexts within textual data. By utilizing machine learning techniques, these algorithms can learn and refine their understanding of language, making them adept at evaluating complex qualitative information.
Another vital algorithm is clustering, which groups similar data points based on specific traits or patterns. This process helps researchers discern relationships within qualitative data, providing clarity on emerging themes. Additionally, sentiment analysis algorithms assess attitudes and emotions expressed in data, further enriching the understanding of qualitative dimensions. Together, these AI-driven qualitative synthesis techniques enhance the research process, allowing investigators to navigate and analyze multifaceted datasets with greater efficiency and accuracy.
Ethical Considerations in AI-Driven Qualitative Synthesis
AI-Driven Qualitative Synthesis presents unique ethical considerations that researchers must navigate. One of the foremost concerns is data privacy, as sensitive information may be inadvertently exposed during synthesis. Ensuring confidentiality is paramount, particularly when handling interviews or open-ended survey responses. Researchers should implement robust data safeguarding measures to protect participant anonymity and comply with data protection regulations.
Additionally, bias within AI algorithms can skew results, presenting significant challenges in qualitative synthesis. It is crucial to actively identify and mitigate biases that may arise through AI's learning processes. Evaluating the training data and ensuring diverse representation can help neutralize these biases, promoting a more balanced analysis of qualitative data. By transparently addressing these ethical considerations, researchers can enhance the credibility and reliability of AI-Driven Qualitative Synthesis while fostering trust among participants and stakeholders.
Discuss the ethical considerations and challenges posed by employing AI in qualitative research synthesis, such as data privacy and bias mitigation.
Employing AI in qualitative research synthesis raises significant ethical considerations, particularly concerning data privacy and bias mitigation. Researchers must ensure that the personal data acquired from participants is securely handled and anonymized to protect individual identities. This responsibility includes following legal guidelines and ethical standards to maintain confidentiality. Moreover, AI algorithms can inadvertently perpetuate existing biases present in the training data, which can skew research findings. It is crucial to continuously assess and refine these algorithms to minimize such biases.
Another challenge researchers face is the transparency of AI-driven processes. Researchers should be clear about how AI tools interpret and analyze qualitative data, ensuring that insights generated can be trusted and validated. This transparency not only fosters ethical research practices but also enhances participant trust in the process. Ultimately, while AI-Driven Qualitative Synthesis offers innovative solutions, it is imperative to navigate these ethical challenges diligently to ensure responsible research outcomes.
Steps to Synthesize Qualitative Research Using AI-Driven Technologies
To synthesize qualitative research effectively using AI-driven technologies, researchers can follow a structured approach. The first step involves data collection and preparation. This includes gathering diverse qualitative data from interviews, focus groups, and surveys. Ensuring data quality and consistency is vital, as it sets the foundation for accurate synthesis.
Next, the analysis and interpretation phase utilizes AI-driven qualitative synthesis tools. These technologies take the prepared data and analyze it, extracting themes and insights that might be overlooked in manual coding. AI can swiftly process large datasets, offering researchers timely insights that enhance the decision-making process. Lastly, integration and presentation of the synthesized data are crucial for fulfilling research objectives. Researchers should present findings in a coherent manner, ensuring they are accessible and actionable for stakeholders. By following these steps, researchers can leverage AI to optimize their qualitative synthesis processes.
Break down the synthesis process into manageable steps, providing a clear roadmap for researchers.
To effectively synthesize qualitative research using AI-driven technologies, researchers can follow a structured roadmap. This roadmap consists of important steps that guide the synthesis process, ensuring clarity and efficiency. The first step involves the careful collection of mixed-source qualitative data, emphasizing the need for meticulous preparation to enhance data quality.
Once data is gathered, the next phase is critical analysis and interpretation. Here, AI-driven qualitative synthesis comes into play, allowing for deeper insights through advanced algorithms that recognize patterns and relationships within the data. Following analysis, researchers must integrate and present the synthesized findings in a coherent manner. This presentation should align with research objectives, making insights impactful and actionable. By clearly mapping out these steps, researchers can navigate the complexities of qualitative synthesis with confidence, ultimately leading to more reliable outcomes.
Step 1: Data Collection and Preparation
Data collection and preparation are vital initial steps in conducting AI-driven qualitative synthesis. Start by gathering qualitative data from diverse sources, such as interviews, focus groups, and open-ended survey responses. Ensuring data quality is essential; this means verifying the accuracy and consistency of the information collected. Transcribing interviews accurately is particularly crucial, as this will form the backbone of your dataset. It allows for a seamless integration of insights later in the analysis.
Next, organize your data methodically. Create a coding scheme to group similar themes and insights, which simplifies the subsequent analysis phase. Ensure that your data is stored securely, especially if it contains sensitive information. This structured preparation sets the stage for AI tools to efficiently parse through the data, extracting rich insights and meaningful patterns, ultimately enhancing the depth of your qualitative synthesis.
Detail how mixed-source qualitative data can be collected and prepped for AI analysis, emphasizing the importance of data quality and consistency.
To effectively collect and prepare mixed-source qualitative data for AI analysis, researchers must prioritize data quality and consistency. Begin by gathering data from various formats, such as interviews, surveys, and focus groups, ensuring that each source is relevant to the research objectives. Itโs crucial to standardize data formats during collection. This includes transcribing interviews accurately and categorizing survey responses to facilitate ease of analysis.
Next, focus on cleaning the data, which involves removing duplicates, correcting errors, and ensuring that the dataset is devoid of bias. Consistency is key here; all researchers involved must adhere to the same data collection protocols to minimize discrepancies. This preparation stage establishes a trustworthy foundation for AI-Driven Qualitative Synthesis, leading to more reliable insights. By rigorously adhering to quality standards, researchers can enhance the interpretative power of AI, ultimately delivering more valuable, actionable results.
Step 2: Analysis and Interpretation with AI-Driven Qualitative Synthesis
In this step of the process, we delve into Analysis and Interpretation using AI-Driven Qualitative Synthesis. This involves using advanced AI tools to analyze qualitative data collected from various sources, such as interviews and surveys. Such synthesis helps researchers derive nuanced insights quickly and effectively, overcoming the traditional barriers of time-consuming manual analysis.
AI technologies can streamline interpretation by identifying patterns and themes within complex data. By employing algorithms that analyze diverse qualitative inputs, researchers can gain a deeper understanding of the information at hand. This automated analysis not only reduces the risk of human bias but also ensures that insights are delivered faster. Overall, utilizing AI-Driven Qualitative Synthesis enables a more efficient approach to extracting valuable conclusions from multifaceted qualitative research. Through this method, researchers can more effectively address client demands for timely and accurate insights.
Explain how AI technologies analyze qualitative data to derive meaningful insights, showcasing the interpretative capabilities of AI.
AI technologies analyze qualitative data through advanced techniques that transform vast amounts of unstructured information into meaningful insights. By utilizing natural language processing (NLP) and machine learning algorithms, AI can recognize patterns, themes, and sentiments within text data. This process enables researchers to derive deeper interpretations that can influence decision-making and strategic initiatives.
Moreover, AI-driven qualitative synthesis showcases the interpretative abilities of AI by providing context-specific insights. For instance, it can extract relevant quotes and sentiments based on the researcher's defined themes, allowing for a nuanced understanding of complex issues. This ability not only enhances the richness of the data but also promotes a more data-informed approach to qualitative research. By embracing AI in qualitative analysis, researchers can achieve a level of efficiency and depth that may be challenging through traditional methods. This evolution signifies a pivotal step toward more meaningful and actionable research outcomes.
Step 3: Integration and Presentation of Synthesized Data
The integration and presentation of synthesized data is crucial for conveying the findings derived from AI-Driven Qualitative Synthesis. Once you have analyzed and interpreted your qualitative data, it's time to connect the dots. This step involves merging insights from various sources into coherent themes that accurately reflect your research objectives. Proper integration not only enhances understanding but also fosters an environment where stakeholders can grasp complex insights easily.
To effectively present your synthesized data, consider the following approaches:
Theme Development: Identify key themes arising from your analysis, which will guide the interpretation of the data.
Visualization Techniques: Utilize graphs, infographics, or interactive dashboards to make data accessible and engaging. This helps clarify relationships between various insights.
Structured Reporting: Create concise reports that summarize findings alongside relevant evidence. This can include direct quotes or notable statistics from your data.
By focusing on these elements, researchers can ensure that their synthesized data resonates with audiences and serves its intended purpose. Presenting qualitative research insights through AI effectively bridges the gap between data and actionable conclusions.
Guide researchers on how to integrate and present the data synthesized by AI, ensuring it meets research objectives.
Integrating and presenting data synthesized by AI requires a thoughtful approach to ensure researchers meet their objectives. Begin by organizing the synthesized insights around key themes, as this helps to create a cohesive narrative. Clearly identify which insights directly respond to your research questions, enhancing the relevance of your findings. Consider incorporating supporting quotes to add depth and credibility to the presented data, which not only enriches context but also offers clear evidence from the subjects of your research.
To effectively communicate your findings, create structured reports that summarize key insights, methodologies, and conclusions. Use visuals like charts or graphs where appropriate, as they can help convey complex data in an accessible manner. This visual representation, combined with a narrative that ties together various data points, will greatly enhance the clarity and impact of your research. Ensuring transparency regarding your data sources builds trust, critical for the integrity of AI-driven qualitative synthesis.
Tools for AI-Driven Qualitative Synthesis
In the realm of AI-Driven Qualitative Synthesis, choosing the right tools is crucial for effective analysis. Various advanced software solutions can streamline the process and enhance the quality of insights gathered from qualitative data. Researchers are increasingly turning to tools that facilitate the extraction of themes and patterns from interviews, open-ended survey responses, and focus groups. Key tools are designed not only to analyze data but also to ensure consistency and reduce bias in findings.
Among the prominent tools for AI-driven synthesis are NVivo, Dedoose, MAXQDA, and ATLAS.ti. These platforms offer features tailored for qualitative researchers, such as automatic coding and visualization capabilities. NVivo excels in managing complex data sets, while Dedoose integrates quantitative and qualitative analysis seamlessly. MAXQDA is celebrated for its user-friendly interface, and ATLAS.ti is favored for its robust collaboration features. By utilizing these tools, researchers can effectively navigate the challenges of qualitative data synthesis, enhancing both the efficiency and depth of their analysis.
Explore the leading tools available for conducting AI-driven qualitative synthesis, enabling researchers to select appropriate technologies for their needs.
To explore AI-driven qualitative synthesis, researchers can leverage a variety of advanced tools designed for analyzing mixed qualitative data. Each tool offers unique features that can greatly enhance the efficiency and accuracy of qualitative research processes. Selecting the right technology hinges on your specific needs, such as customizability, ease of use, and compatibility with existing workflows.
First, consider NVivo, known for its robust data management capabilities. It supports intricate coding and visualization, making it suitable for in-depth analyses. Next, Dedoose specializes in integrating qualitative and quantitative research, ideal for mixed-methods studies. MAXQDA stands out with its powerful analytics and user-friendly interface, perfect for researchers seeking versatility in data visualization. Lastly, ATLAS.ti offers comprehensive coding tools and a collaborative environment, perfect for teams working on extensive qualitative datasets. These tools empower researchers to effectively synthesize qualitative insights, leading to quicker project turnarounds and more robust conclusions.
insight7
In the realm of AI-driven qualitative synthesis, the insights derived from data can lead to significant breakthroughs. By harnessing artificial intelligence, researchers can efficiently analyze qualitative data collected from varied sources. This approach not only enhances the understanding of complexities but also uncovers nuanced patterns that might be overlooked in traditional analysis.
To successfully conduct AI-driven qualitative synthesis, it's essential to follow a structured approach. First, qualitative data must be meticulously gathered and prepared to ensure high quality for the AI tools. Next, the advanced algorithms process this data, interpreting themes and sentiments. Finally, the synthesized results should be presented in a manner that aligns with research objectives, allowing stakeholders to make informed decisions based on clear insights. Embracing AI in qualitative research opens new avenues for innovation and depth in understanding human experiences.
Positioning insight7 as a top tool for synthesizing qualitative research through its advanced AI capabilities.
AI-Driven Qualitative Synthesis stands at the forefront of qualitative research methodologies, significantly enhancing how insights are derived from complex data sets. The integration of advanced AI capabilities streamlines the process of analyzing qualitative data, ensuring that researchers can effectively manage time and resources. By automating analysis, researchers reduce biases and inconsistencies often encountered in team-based analyses. The result is a more efficient synthesis process that delivers rapid and reliable insights.
When exploring the capabilities of this advanced tool, it is essential to understand that it offers unique features gearing towards qualitative synthesis. First, its robust algorithms successfully process vast volumes of mixed-source data, identifying patterns and themes with precision. Next, real-time collaboration features facilitate seamless communication between team members, minimizing discrepancies in data interpretation. Lastly, the ability to generate comprehensive reports swiftly empowers researchers to respond to client needs promptly, ultimately enhancing decision-making and outcomes. Embracing such an AI-driven tool transforms the landscape of qualitative research.
Additional Tools for AI Synthesis
Additional tools for AI synthesis play a significant role in enhancing the efficiency of qualitative research. Various software programs are designed to facilitate AI-driven qualitative synthesis, ensuring researchers can analyze data more quickly and with greater accuracy. For instance, tools like NVivo and Dedoose provide robust features necessary for organizing and coding data, which aid in unveiling critical insights from interviews and open-ended survey responses. Their capabilities streamline the manual aspects of data handling, enabling researchers to focus on interpretation rather than tedious data management.
Moreover, MAXQDA and ATLAS.ti further support researchers by offering advanced functionalities for visualizing relationships and themes. These tools can integrate various data types, allowing for a more comprehensive analysis. By effectively utilizing these additional tools for AI synthesis, researchers can produce reliable insights, enhance collaboration within teams, and meet the increasing demand for timely reporting in qualitative research projects. Embracing these technologies can significantly impact research efficiency and the quality of outcomes.
- NVivo
NVivo serves as a vital tool in the realm of AI-Driven Qualitative Synthesis, facilitating the analysis of qualitative data collected from various sources. Researchers often face the challenge of managing and interpreting extensive qualitative datasets. By employing NVivo, users can efficiently organize, code, and analyze data, thereby uncovering meaningful insights that might otherwise be overlooked. This software combines human expertise with AI capabilities, enhancing the overall process of qualitative synthesis.
One key feature of NVivo is its ability to integrate diverse data types, including interviews, surveys, and social media content. As a result, researchers can synthesize findings across these platforms seamlessly. Additionally, NVivo supports various analytical techniques that facilitate the identification of patterns and trends, essential for drawing comprehensive conclusions. By leveraging NVivo's functionalities, researchers can enrich their qualitative analysis and ultimately achieve a more profound understanding of their subject matter, making it an indispensable asset in AI-Driven Qualitative Synthesis.
- Dedoose
Dedoose serves as an invaluable tool within the realm of AI-Driven Qualitative Synthesis. It simplifies the intricate process of qualitative data analysis, allowing researchers to efficiently upload and organize multiple data sources. Users can harness its powerful features to conduct in-depth analyses of interviews, surveys, and other qualitative data with ease. Additionally, the platform's intuitive interface streamlines the extraction of themes, quotes, and visualizations, making it easier to translate findings into actionable insights.
By incorporating robust functionalities like native transcription services and bulk data uploads, it boosts both accuracy and efficiency. This aligns seamlessly with the tenets of AI-Driven Qualitative Synthesis, transforming how researchers interact with qualitative data. Ultimately, utilizing such tools enhances collaboration and enables teams to derive meaningful conclusions from complex datasets, paving the way for informed decision-making and strategic initiatives.
- MAXQDA
MAXQDA serves as an essential tool for those seeking to enhance their approach to qualitative research synthesis. In the realm of AI-Driven Qualitative Synthesis, this software offers features that facilitate the analysis of diverse qualitative data sources. Researchers can efficiently organize and code their data, making it easier to extract meaningful insights from large volumes of information.
The functionalities provided by MAXQDA streamline the synthesis process. Users can seamlessly integrate text, audio, and video data, allowing for a holistic examination of the gathered information. Furthermore, the software employs advanced analytical tools, which can aid in visualizing data and identifying patterns that may not be apparent at first glance. As researchers increasingly rely on AI technologies, incorporating tools like MAXQDA can significantly enhance their ability to draw comprehensive conclusions from mixed qualitative sources.
- ATLAS.ti
In the world of AI-Driven Qualitative Synthesis, effective tools play a crucial role in enhancing the research process. One such tool is instrumental in streamlining the synthesis of qualitative data from mixed sources. It empowers researchers to organize, analyze, and visualize data efficiently. This tool provides intuitive features that facilitate the coding of qualitative data, making it easy to identify themes and patterns.
Furthermore, the tool harnesses advanced algorithms that aid in data interpretation. By utilizing AI capabilities, it helps researchers uncover insights that may be hidden in complex datasets. As researchers sift through interviews, open-ended surveys, and other qualitative materials, this tool becomes an essential companion, ensuring that no valuable information goes unnoticed. Ultimately, embracing such sophisticated tools allows researchers to focus more on analysis rather than getting bogged down by data management, fostering deeper insights and informed decision-making in the synthesis process.
These tools are discussed in terms of their features and how they support AI-driven qualitative synthesis.
The landscape of qualitative synthesis is evolving, thanks to advancements in AI technologies. The tools available today offer robust features that streamline the qualitative research process, making it more efficient and less prone to human error. For instance, tools like NVivo and Dedoose provide functionalities such as automated coding, thematic analysis, and collaborative platforms, greatly enhancing the synthesis process. These features not only reduce the time spent on manual tasks but also increase the reliability of the insights generated.
In terms of AI-driven qualitative synthesis, the ability to analyze vast amounts of data quickly offers significant advantages. Advanced algorithms enable these tools to identify patterns and themes in qualitative data, providing researchers with actionable insights. Furthermore, the user-friendly interfaces of these tools allow researchers from various backgrounds to utilize AI effectively, bridging the gap between complex technology and practical application in qualitative research. As researchers continue to explore these tools, they are likely to realize improved consistency, speed, and depth in their qualitative analyses.
Conclusion: The Future of AI-Driven Qualitative Synthesis
The journey of AI-Driven Qualitative Synthesis is just beginning, yet its potential for transforming research is immense. As researchers increasingly grapple with data complexity, the integration of AI tools aids in streamlining analysis, reducing biases, and promoting consistency in insights. Looking ahead, we can expect rapid advancements in AI technologies, enhancing their capacity to collaborate with human creativity and critical thinking.
Moreover, the future will likely see the development of more intuitive AI platforms that make qualitative synthesis accessible to diverse fields. This evolution can empower researchers to convert vast amounts of qualitative data into actionable insights more efficiently than ever before. Embracing these changes will be crucial for academics and practitioners who seek to elevate the quality and impact of their work.
Summarize the potential of AI-Driven Qualitative Synthesis in transforming how qualitative research is conducted and synthesized, offering insights into future developments.
AI-Driven Qualitative Synthesis holds immense potential to revolutionize qualitative research by streamlining processes and enhancing data analysis. Researchers often face challenges such as time-consuming manual analysis and inconsistencies in interpreting findings. AI technologies can significantly reduce the time required for synthesizing diverse qualitative data, allowing researchers to focus on generating meaningful insights rather than labor-intensive tasks. By automating coding and identifying themes, AI can uncover patterns that might be overlooked in traditional analyses.
Looking to the future, advancements in AI will likely result in even more sophisticated synthesis methods. Expect improvements in natural language processing and machine learning capabilities, which will enhance the accuracy and richness of insights derived from qualitative data. As AI continues to evolve, its integration with qualitative research will create opportunities to unlock deeper understanding, encouraging collaborative and informed decision-making processes. This transformation paves the way for richer analysis and greater accessibility of qualitative insights across industries.