AI Scheduling Innovations have transformed workforce management, enabling organizations to navigate challenges in real-time. As businesses adapt to evolving demands, incorporating sophisticated forecasting features has become essential for optimizing agent scheduling. From dynamic load modeling to intraday prediction, these innovations enhance responsiveness and accuracy, ensuring that the right agents are available at peak times.
Skill-based routing forecasts and scenario planning further refine this process, allowing for personalized task assignments that match agent capabilities. With advancements in volume spike detection and shrinkage impact modeling, AI empowers managers to preemptively address fluctuations and minimize disruptions. As we explore these cutting-edge forecasting features, organizations can achieve unparalleled precision in scheduling, ultimately fostering a more efficient and satisfied workforce.
Analyze qualitative data. At Scale.
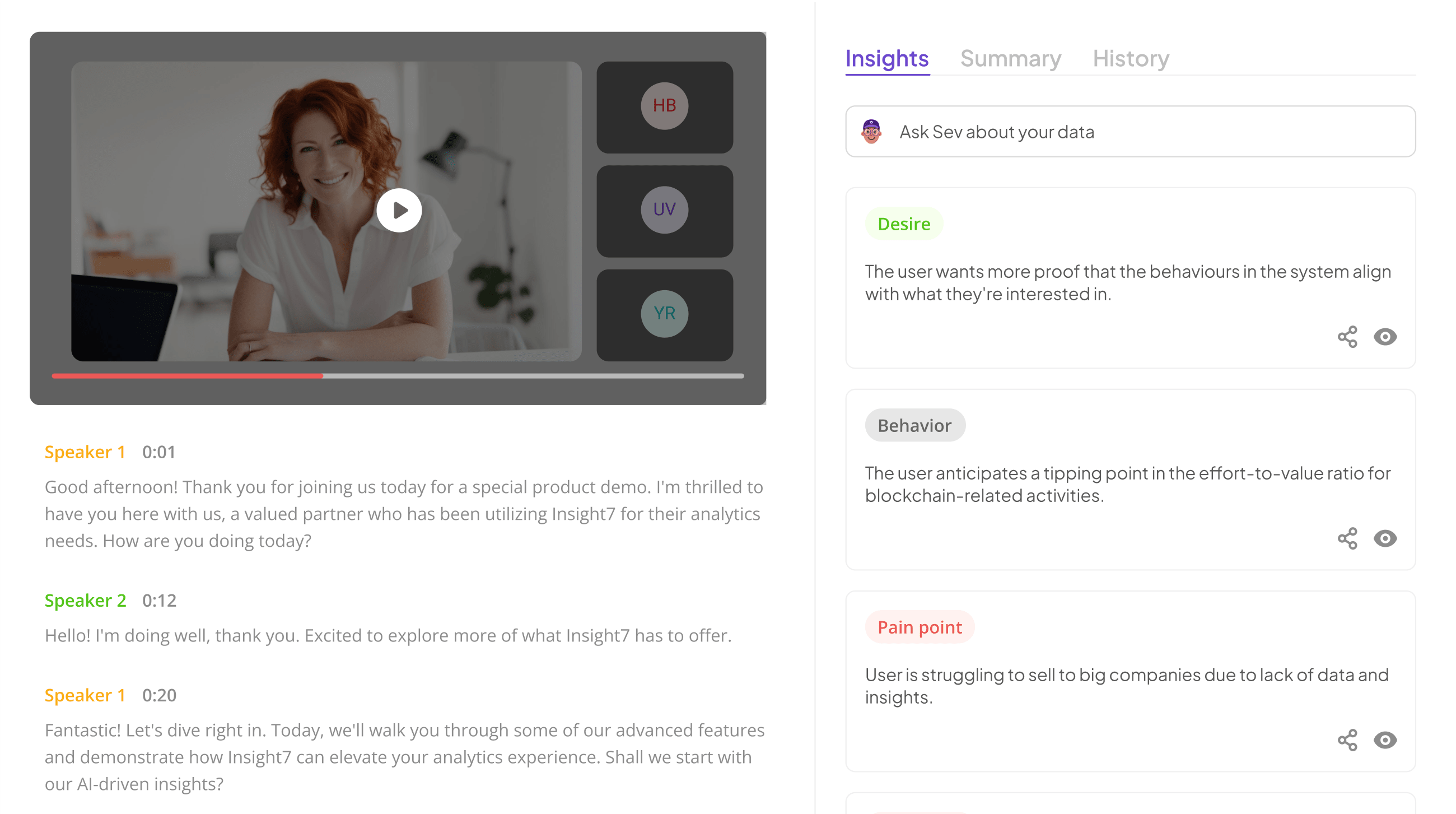
Understanding AI Scheduling Innovations
AI Scheduling Innovations provide transformative capabilities in workforce management that enhance scheduling precision. Understanding these innovations begins with recognizing how predictive technologies can optimize agent performance. Through dynamic load modeling, organizations can gain insights into varying workloads and adjust schedules accordingly. In tandem, intraday prediction allows for real-time adjustments to accommodate unexpected changes, leading to improved responsiveness and efficiency.
Additionally, skill-based routing forecasts are critical in tailoring tasks to agents based on their unique expertise. This not only boosts productivity but also employee satisfaction. Furthermore, scenario planning empowers organizations to prepare for future changes, ensuring that agent schedules remain robust and adaptable. By integrating these AI scheduling innovations, businesses can effectively navigate complexities, enhance service delivery, and maintain operational efficiency, positioning themselves competitively in the market.
Dynamic Load Modeling and Intraday Prediction
Dynamic load modeling is essential for optimizing workforce schedules by analyzing historical data and predicting fluctuations in workload. This technique enables organizations to better anticipate demand and allocate resources efficiently, thereby minimizing idle time and improving overall productivity. By understanding dynamic load patterns, managers can enhance their scheduling strategies and ensure that the right number of agents are available exactly when needed.
Intraday prediction further refines this approach by providing real-time insights into workload changes throughout the day. It empowers organizations to respond promptly to unexpected spikes or drops in demand, ensuring seamless service delivery. Together, dynamic load modeling and intraday prediction form a core part of AI scheduling innovations, enabling more precise and effective agent scheduling. This ultimately fosters a more responsive customer service environment, resulting in improved satisfaction and operational efficiency.
- Explanation of dynamic load modeling and its impact on scheduling.
Dynamic load modeling is a critical component of modern AI scheduling innovations. It involves analyzing workload fluctuations to ensure optimal resource allocation throughout the day. By understanding when demand peaks and troughs occur, organizations can efficiently assign agents to specific tasks, minimizing idle time and maximizing productivity.
The impact of dynamic load modeling on scheduling is profound. It allows for proactive adjustments in real-time, accommodating unexpected changes such as sudden volume spikes or reduced agent availability. As a result, businesses can maintain high service levels and enhance customer satisfaction. This approach ultimately leads to better forecasting accuracy, ensuring agents are available precisely when needed while supporting overall operational efficiency. In a world where agility is vital, dynamic load modeling stands as an essential tool in the AI-driven scheduling arsenal.
- How intraday prediction enhances real-time response in AI scheduling.
Intraday prediction is a pivotal feature in AI scheduling innovations, significantly improving real-time responsiveness for workforce management. By analyzing current conditions and updated data, intraday prediction allows an AI system to make informed adjustments to schedules dynamically. This adaptability enhances operational efficiency and ensures agents are optimally aligned with fluctuating demand throughout the day.
Moreover, intraday prediction enables organizations to promptly respond to unexpected events, such as sudden volume spikes or agent absences. With accurate real-time insights, businesses can recalibrate their workforce effectively, ensuring customer needs are met without delay. This proactive approach not only minimizes disruptions but also increases overall agent productivity by keeping them engaged with relevant tasks at all times. Embracing intraday prediction within AI scheduling enhances agility, positioning businesses to thrive in an ever-changing landscape.
Skill-Based Routing Forecasts and Scenario Planning
Skill-based routing forecasts play a crucial role in optimizing the scheduling of agents in a dynamic work environment. By analyzing agent skills and matching them to the tasks that require specific expertise, organizations can improve customer interactions significantly. This targeted approach not only enhances service quality but also boosts agent productivity. It empowers scheduling innovations by ensuring the right agent is assigned to the appropriate task, ultimately leading to higher customer satisfaction rates.
Scenario planning complements skill-based routing by allowing organizations to anticipate future changes in workload, resource availability, and client demands. This proactive strategy helps businesses adjust their scheduling models effectively, preparing them for various potential outcomes. By integrating AI into scenario planning, organizations can simulate different situations, identifying the best possible responses to maintain operational efficiency. Together, skill-based routing forecasts and detailed scenario planning underscore the remarkable potential of AI scheduling innovations in enhancing workforce management strategies.
- The role of skill-based routing forecasts in personalizing agent tasks.
Skill-based routing forecasts significantly enhance the personalization of agent tasks, leading to improved efficiency and customer satisfaction. These forecasts enable organizations to assign tasks that align with individual strengths by analyzing agent skills, preferences, and historical performance. This tailored approach not only boosts productivity but also contributes to higher morale among agents, as they engage in work that aligns with their capabilities.
As part of AI scheduling innovations, skill-based routing forecasts provide vital insights. They help identify the right agents for specific tasks, ensuring that customer interactions are handled by individuals most qualified to respond. This optimization of task assignment reduces handle times and enhances the overall service quality. Furthermore, it allows for more dynamic and agile workforce management, adapting to changing demand in real-time, which is increasingly crucial in todayโs fast-paced business environment.
- Scenario planning for future-proof agent scheduling through AI.
Scenario planning serves as a vital component in future-proofing agent scheduling through AI. By simulating various operational scenarios, businesses can anticipate potential challenges and devise effective solutions. This proactive approach not only enhances scheduling precision but also mitigates risks associated with sudden demand fluctuations or unexpected shrinkage in agent availability.
The integration of AI in scenario planning allows for more accurate forecasting of agent needs under diverse conditions. For instance, by modeling various customer demand patterns, organizations can optimize their workforce allocation accordingly. AI scheduling innovations empower decision-makers to explore multiple outcomes and adjust strategies in real-time, fostering a resilient and adaptable operation. By embracing this dynamic framework, businesses are better equipped to navigate uncertainties and ensure seamless service delivery to their customers.
Extract insights from interviews, calls, surveys and reviews for insights in minutes
Advanced AI Forecasting Features for Scheduling Precision
Advanced AI forecasting features play a crucial role in optimizing agent scheduling precision. These features leverage cutting-edge algorithms to enhance the scheduling process with remarkable accuracy and adaptability. Dynamic load modeling, for instance, evaluates workload fluctuations in real-time, allowing organizations to adjust schedules proactively. Similarly, intraday prediction enables businesses to respond effectively to unexpected changes in call volume, ensuring that agents are available when needed most.
In addition, features like volume spike detection and shrinkage impact modeling collaborate to mitigate potential disruptions. Volume spike detection identifies sudden increases in demand, prompting timely adjustments to agent schedules. Meanwhile, shrinkage impact modeling examines factors such as absenteeism and training, providing insights that empower leaders to optimize workforce deployment. By integrating these advanced AI scheduling innovations, organizations can achieve greater scheduling efficiency, ultimately improving service levels and enhancing customer satisfaction.
Volume Spike Detection and Shrinkage Impact Modeling
Effective volume spike detection is crucial in workforce planning, especially during peak periods. By anticipating sudden increases in call volume, organizations can proactively adjust agent schedules, ensuring enough personnel are available to handle customer demands. This capability minimizes wait times and enhances service quality during busy intervals, which is essential for maintaining customer satisfaction.
Additionally, shrinkage impact modeling plays a significant role in understanding and mitigating the effects of agent availability. By analyzing factors such as absenteeism, breaks, and training, organizations can better forecast the number of agents needed to meet demand. This modeling helps ensure operational efficiency, preventing overstaffing or understaffing situations. Together, these strategies exemplify how AI scheduling innovations transform workforce management, maximizing productivity while minimizing disruptions during high-demand periods.
- How volume spike detection preemptively adjusts schedules.
Volume spike detection plays a crucial role in enhancing agent scheduling efficiency. By anticipating shifts in call volume, AI algorithms can adjust schedules proactively. This ensures that the right number of agents is available at peak times, preventing service delays and customer dissatisfaction. When a sudden increase in demand is detected, schedules can be dynamically altered, allowing organizations to meet high-volume demands without compromising service quality.
Moreover, this feature works hand-in-hand with shrinkage impact modeling, which assesses the effects of factors such as absenteeism on workforce availability. Together, they create a more resilient scheduling framework that adjusts on the fly. Overall, volume spike detection stands as a vital component of AI Scheduling Innovations, enabling businesses to respond effectively to unpredictable interactions and maintain operational effectiveness.
- Importance of shrinkage impact modeling in reducing operational disruptions.
Shrinkage impact modeling plays a crucial role in minimizing operational disruptions within organizations. This process uses predictive analytics to estimate the percentage of workforce availability loss over time. Understanding workforce shrinkage allows companies to better prepare for potential shortfalls in staffing. By anticipating these disruptions, businesses can strategically modify agent schedules, ensuring optimal coverage and maintaining service quality.
Moreover, accurate shrinkage impact modeling provides insight into when operational challenges may arise. This information enables teams to proactively implement adjustments and streamline workflows before issues escalate. In conjunction with AI scheduling innovations, this modeling becomes an effective tool for enhancing overall efficiency. Ultimately, leveraging shrinkage impact modeling leads to more accurate staffing decisions, improved resource allocation, and a smoother operational flow. By addressing workforce shrinkage head-on, organizations can significantly reduce the risk of service failures and enhance agent productivity.
AI-Powered Reforecasting and Agent Adherence Tracking
AI-powered reforecasting dramatically enhances scheduling accuracy by dynamically adjusting predictions based on real-time data. As call volumes fluctuate throughout the day, this technology continuously refines forecasts, ensuring that agents are scheduled in alignment with actual demand. By utilizing historical performance data and recognizing patterns in agent availability, organizations can anticipate needs more effectively. This capability allows for proactive adjustments, minimizing downtime and overstaffing.
Agent adherence tracking complements reforecasting by monitoring performance in real-time, providing insights into how closely agents follow their schedules. Accurate adherence data is vital for understanding operational efficiency and making necessary adjustments. When combined, these two features form a robust framework for optimizing agent schedules. This dual approach not only improves efficiency but also enhances agent satisfaction, creating a more balanced workflow. Moreover, AI scheduling innovations ultimately drive enhanced service quality by ensuring that the right agents are available when needed most.
- The benefits of AI-powered reforecasting in maintaining scheduling accuracy.
AI-powered reforecasting is a transformative feature that significantly enhances scheduling accuracy. By utilizing machine learning algorithms, organizations can dynamically update forecasts based on real-time data. This capability allows businesses to swiftly adapt to fluctuating demands, ensuring that staffing levels align closely with workload expectations.
Another important benefit lies in minimizing disruptions. As unexpected factors emergeโlike sudden volume spikesโAI reforecasting quickly recalibrates schedules, reducing the risk of underperformance. Furthermore, it smoothens the integration of new agents into existing workflows by adjusting training and onboarding timelines based on evolving requirements.
Overall, the adoption of AI technology in reforecasting can greatly improve not only operational efficiency but also employee satisfaction by ensuring that agents are equipped and available when needed most. With AI scheduling innovations, companies can maintain a fine-tuned balance between service quality and resource allocation.
- Techniques for tracking agent adherence and its significance in AI scheduling.
Tracking agent adherence is a vital technique in improving the efficiency of AI scheduling innovations. This process involves monitoring agents’ compliance with their schedules, ensuring they are available and ready to assist during designated times. Accurate tracking allows organizations to identify patterns of adherence, which can be critical in adjusting overall scheduling strategies. By understanding when agents are not adhering to their schedules, businesses can account for this variance in future planning, ultimately leading to enhanced operational efficiency.
Moreover, the significance of tracking agent adherence extends beyond mere attendance. It serves as a metric for evaluating individual agent performance and productivity levels. By integrating agent adherence data with AI scheduling systems, organizations can make dynamic adjustments, like reallocating tasks to different agents based on real-time adherence information. This synergy not only optimizes resource utilization but also enhances customer service by ensuring that appropriately skilled agents are available during peak demand periods. Thus, effective tracking of agent adherence is essential for unlocking the full potential of AI-powered scheduling solutions.
Measuring and Predicting with AI Scheduling Innovations
Measuring and predicting within the realm of AI Scheduling Innovations involves sophisticated techniques that enhance workforce management. Central to this process are forecasting accuracy benchmarks. These benchmarks allow organizations to assess the precision of their scheduling predictions. By establishing these standards, companies can pinpoint discrepancies and improve their scheduling strategies effectively.
Another crucial aspect is SLA variance prediction, which helps maintain operational efficiency. By anticipating variations in service level agreements, companies can proactively address potential disruptions. This foresight not only satisfies client expectations but also enhances overall productivity. The combination of these features supports a comprehensive approach to scheduling, allowing for a dynamic response to changing workloads and staff availability, ultimately driving improvements in service quality and agent satisfaction.
Forecasting Accuracy Benchmarks and SLA Variance Prediction
Establishing forecasting accuracy benchmarks is crucial for optimizing agent scheduling. These benchmarks serve as reference points, allowing organizations to evaluate the effectiveness of their forecasting methods. By comparing predicted performance against actual outcomes, businesses gain insights that drive improvements in scheduling precision. Accurate benchmarks highlight areas needing refinement, ensuring resources are employed effectively and reducing staffing gaps during peak times.
SLA variance prediction also plays a significant role in this context. By anticipating deviations from service level agreements, agents can be guided more effectively, enhancing overall customer satisfaction. AI scheduling innovations empower organizations to analyze historical data and detect patterns, leading to more accurate forecasts. Incorporating these tools into existing operations not only improves efficiency but also establishes a robust framework for ongoing enhancements in workforce management. Ultimately, understanding these metrics paves the way for more agile and responsive scheduling, ensuring that customer expectations are consistently met.
- Establishing benchmarks for assessing forecasting accuracy in agent scheduling.
In agent scheduling, assessing forecasting accuracy is crucial for operational success. Establishing benchmarks allows organizations to objectively evaluate the effectiveness of their scheduling strategies. These benchmarks should reflect key performance indicators, such as accuracy rates, forecasting errors, and adherence levels. By focusing on these metrics, businesses can identify gaps in performance and areas needing improvement.
Incorporating AI Scheduling Innovations can significantly enhance this benchmarking process. For instance, integrating volume spike detection and dynamic load modeling allows organizations to adjust their benchmarks dynamically based on real-time data. This adaptability, coupled with AI-powered reforecasting, ensures that benchmarks remain relevant in an ever-evolving operational landscape. Ultimately, clear benchmarks for forecasting accuracy will enable organizations to optimize agent scheduling, maintain service level agreements, and improve overall customer satisfaction.
- Predicting SLA variance to sustain operational efficiency and client satisfaction.
Predicting SLA variance is crucial for maintaining operational efficiency and ensuring client satisfaction in service-oriented environments. By utilizing advanced AI scheduling innovations, organizations can gain insights into potential deviations from Service Level Agreements (SLAs). Accurately forecasting SLA variance enables teams to proactively adjust their resources and strategies, ultimately minimizing delays and enhancing service delivery.
To achieve this, AI scheduling innovations leverage dynamic load modeling and intraday predictions, allowing teams to anticipate workload fluctuations and allocate staff accordingly. Furthermore, understanding volume spike detection and shrinkage impact modeling aids in recognizing periods of high demand and potential staffing gaps. By effectively predicting SLA variance, businesses can not only uphold customer expectations but also foster a more efficient workplace where agents are optimally utilized, leading to overall improvements in client satisfaction.
Analyze qualitative data. At Scale.
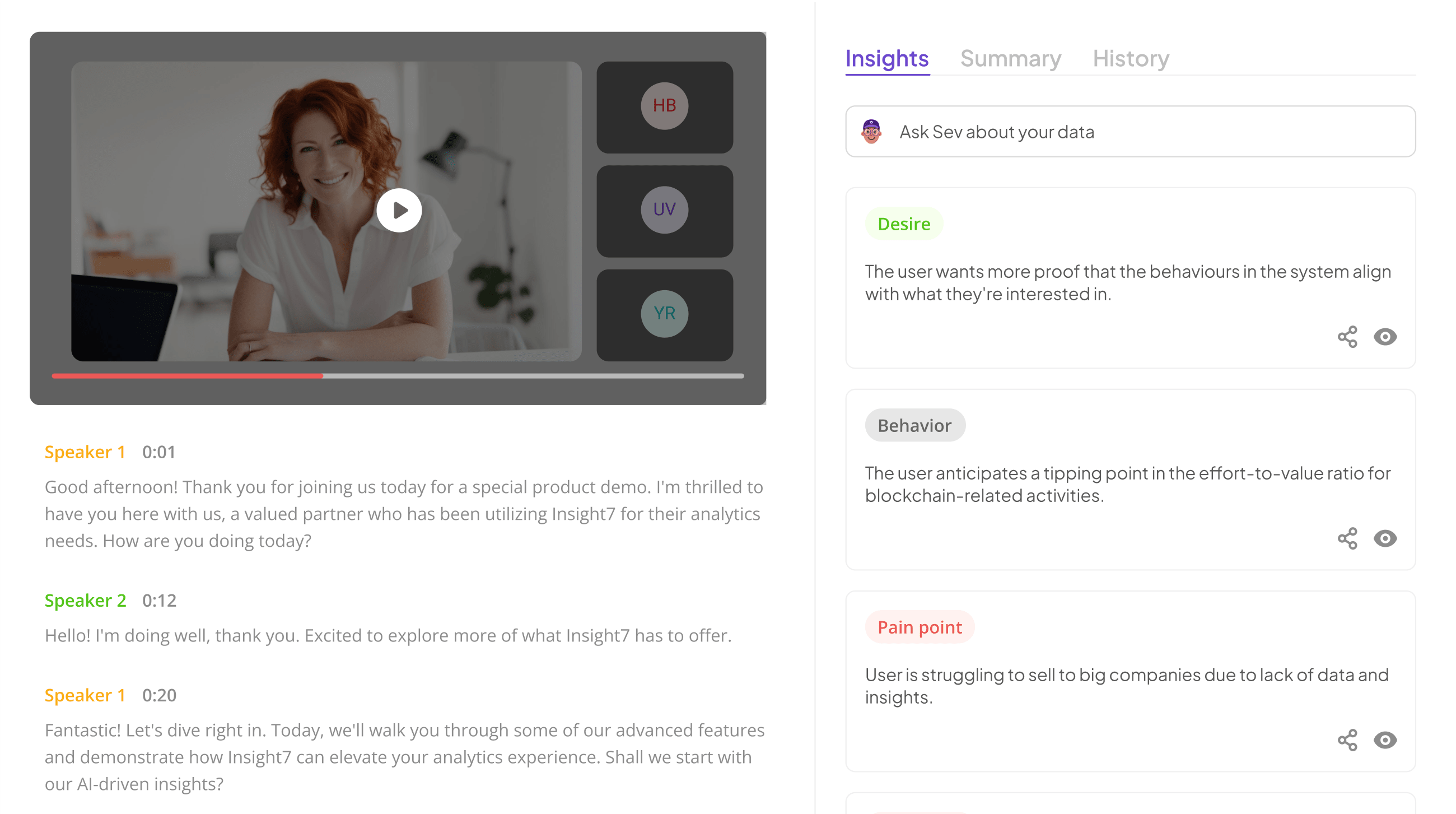
Conclusion: The Future of AI Scheduling Innovations
AI scheduling innovations will undoubtedly redefine workforce management as we look toward the future. These advancements will empower organizations to adopt precision tools like dynamic load modeling and intraday predictions. Accompanying these technologies are skill-based routing forecasts, which tailor tasks to individual agent strengths. This synergy fosters greater efficiency and ensures that each customer interaction is optimized for success.
Furthermore, scenario planning and volume spike detection will enhance our ability to forecast operational needs accurately. By integrating AI-powered reforecasting and agent adherence tracking, companies can respond proactively to changing demands. Ultimately, embracing these AI scheduling innovations will ensure not only improved scheduling accuracy but also enhanced overall productivity and service quality in a rapidly evolving landscape.